
Promoting a sale on a product often has a huge impact on the number of its sales overall. However, the successful execution of a sales promotion is possible if and only if the increase in sales volume is accounted for in all phases of the supply chain.
The sales promotion of one product may have significant secondary effects on the sales of other products not in promotion – a fact that is often forgotten or left with little attention. Disregarding these secondary effects leads to suboptimal planning and prevents planners from reaching the full profit potential of a sales promotion.
This whitepaper discusses these secondary effects of a sales promotion, often referred to as the cannibalization and halo effects and their causes and effects in demand forecasting.
Understanding the cannibalization and halo effects in consumer behavior
Consider this: a customer is standing in front of the fresh meat cabinet at their local supermarket with a hand-written sticky note — next item on the shopping list: 2 lbs. of ground beef. When looking at the cabinet, they spot at least 15 options from different brands with varying fat content, all matching the vague criteria on their list.
A few of the options are organic, and some of the options have already been shaped into burger patties and seasoned. Will they pick the same brand they used last time or try something else in the hope of making an even better burger? Oh, never mind! Product X is at a 20 % discount, so they go straight to the savings and buy product X instead.
It is easy to think of these examples of how sales promotions affect shopping behavior. If two products are almost perfect substitutes for each other, and one is on substantial discount, the promoted product likely ends up in the customer’s shopping basket. Consequently, that same sales promotion might decrease or cannibalize the sales of a similar product.
As an entirely opposite phenomenon, some products, like gin and tonic water, are frequently bought together; hence promoting one product may increase the sales of its complement. This consequence, in turn, is called the halo effect.
Identifying the cannibalization and halo effects through sales data
In order to take cannibalization and halo effects into account in demand forecasting, it is beneficial first to recognize relevant relationships out of millions of possibilities. The simplest, yet not very practical, way to recognize these correlations would be to rely on common sense and list the potential relationships manually. Extra-lean ground beef probably cannibalizes similar products, but does it also cannibalize standard ground beef? How about organic extra-lean ground beef?
Figuring out all relevant relationships using common sense is not quite as easy as it might first seem, and manually generating and maintaining a list for thousands of products is by no means feasible. Hence, the only practical option is to identify the relationships from historical data using machine learning techniques.
In the retail business, two useful datasets are often available for this purpose. The first option is to identify product substitutes and products frequently bought together from receipt-level transactions and loyalty card data using processes such as association rule learning.
For instance, if two products are never included in the same shopping basket or if the preference of a single customer seems to vary between two similar products arbitrarily, there is a high probability that the products are, in fact, substitutes and would, most likely, be in a cannibalization relationship with each other. The same technique is straightforward for relationships involving the halo effect. If two products are bought more frequently together than they would be separate, they are probably in a halo relationship.
The second option for identifying the cannibalization and halo effect in your data is by examining SKU-store-level time series data. Consider a system consisting of only one pair of products during normal sales periods (outside promotions) and with both products in stock. This system should be in a kind of equilibrium. Both products are bought somewhat randomly, and the proportional sales of each product are affected by different factors, such as brand, price, and display.
It is hard to draw any useful conclusions on cannibalization or halo relationships based on the equilibrium sales alone. However, when the equilibrium goes off-balance – as a consequence of a promotion, for instance – the relationships become prominent.
Suppose two products are in a cannibalization relationship. In that case, a sales promotion of the first product should increase its sales while decreasing the sales of the second product compared to its usual equilibrium. Thus, the effects of the cannibalizing promotion on the sales of the two products are negatively correlated. On the other hand, a halo effect would result in a positive correlation.
Analyzing the strength and significance of these correlations is a robust and relatively efficient way of identifying cannibalization relationships from large masses of sales data. As an added benefit, this technique provides easily quantifiable information on the strength and relevance of the relationships, facilitating the filtering of unimportant relationships and highlighting more prominent ones.
From cannibalization and halo relationships to more accurate forecasts
After the relevant relationships are known, the dynamic effects of promotions can be introduced in the forecast calculation using a similar approach to the calculation of normal promotional effects.
Since the halo effect increases the sales of all its supplements during the same promotion period, the halo effect can simply be considered a special kind of promotion. However, the halo effect is weaker than the effect of the original campaign unless it involves products that always sell together.
Correspondingly, cannibalization materializes as special promotions with a negative effect on sales. As seen in Figure 1, usually, the primary effect of a promotion on a product’s own sales is much more prominent than the secondary cannibalization effect, which originates from the promotional activities of a substitute product.
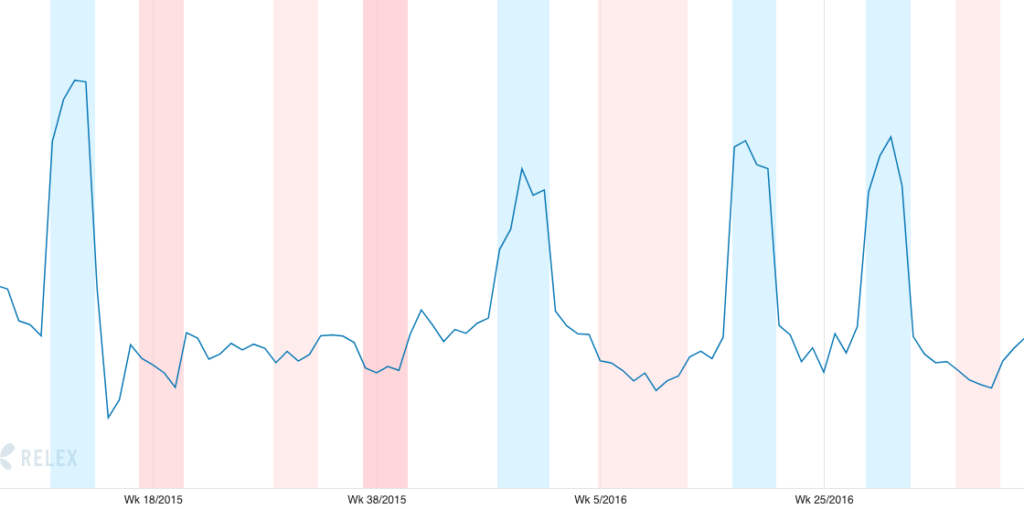
A robust supply chain technology can use machine learning to identify cannibalization relationships based on historical sales and promotional data. The system incorporates known cannibalization and halo relationships in forecasting to improve the forecast accuracy during promotions, cutting the spoilage of cannibalized products and increasing the availability of haloed products.
In addition, the forecast accuracy outside all promotional effects can improve because the machine learning algorithm correctly interprets the biasing cannibalization and halo effects as being promotion-related and not part of baseline demand.
The need for visibility into the cannibalization and halo effects for suppliers and wholesalers
The effects of a sales promotion can extend beyond the retail part of the supply chain, affecting the inventory of suppliers and wholesalers. As mentioned, the cannibalization and halo effects influence the success or detriment of similar products. Without visibility into these additional promotional needs, supplier and wholesalers maybe not be able to provide the necessary inventory or effective service level to remain competitive and service their retail partners’ needs.
For a wholesaler, the lack visibility into the demand of their retail partners can lead to two undesirable outcomes: a stockpile of unsold products due to the cannibalization effect, or a decrease in service level for a needed product due to the halo effect. This could disrupt the wholesaler’s service level or risk their financial investment by holding a product that is not selling in stores.
However, maintaining visibility into retail forecasts and potential promotional effects can give wholesalers an advantage by allowing them to prepare for promoted items and complementary products to maximize the benefits of a sales promotion.
Consumer goods companies that lack supply chain visibility may run into similar issues when retailers prefer to purchase specific versions or brands of one product over another. Retailers may choose products from a specific supplier based on their customers’ affinity for a particular product or group of products featured in a sales promotion and their halo effect counterparts.
Supply chain collaboration technology can alleviate this lack of visibility and suppliers can better understand retailer demand, adjust production accordingly, and ensure that the right products are available at the right time.
In addition to the benefits of maintaining an accurate demand forecast, investing in collaboration tools that increase visibility across the entire supply chain can help suppliers, wholesalers, and retailers better prepare together for the potential influence of the cannibalization and halo effects. Collaboration ensures that everyone benefits from the sale, and inventory and service levels remain stable during promotions.