1. DIY retail strategies only succeed with the right supply chains
DIY retail has always been a challenging industry. DIY and home improvement retailers typically offer an extensive assortment—sometimes up to 80,000 SKUs per location across multiple regions with hundreds, even thousands of stores and up to a million SKUs in online channels.
These retailers must also grapple with more seasonal and slow-moving products, bulky items, and high-value goods than their peers in other retail sectors. The size of the DIY assortment combined with that assortment’s complexity makes effective, efficient supply chain execution an incredibly challenging undertaking.
If you are a DIY or home improvement retailer, you need the right planning tools and an understanding of how to apply those tools intelligently for effective supply chain management. Doing so will enable your business to successfully address your key challenges, such as:
Seasonal products that require advanced forecasting and replenishment planning as well as granular analysis to ensure inventory reaches the right stores, with delivery timed to maximize sales and minimize end-of-season markdowns.
Items with long lead times are expensive to move and often require retailers to deal with international suppliers, which means lead times and deliveries can be unpredictable. DIY retailers need to be able to forecast up to a year in advance and optimize orders for supplier requirements and the lowest cost of delivery to stores.
Slow-moving items present forecasting challenges due to their sparse sales data. When they do sell, they sometimes sell in large quantities, creating “lumpy” demand that can be even harder to manage. The ability to effectively use what little data these items generate and manage replenishment down to the store-product level is essential to balance safety stock and out-of-stocks.
Omnichannel retail for DIY has grown to the point where retailers can no longer operate online channels as an “add-on” service exempt from standard efficiency requirements. Broad assortments with a high proportion of slow movers and unpredictable demand further accentuate the need for operational efficiency across all channels.
In this best practice guide, we’ll highlight key approaches for increasing responsiveness and efficiency in DIY and home improvement supply chains. You’d be hard-pressed to find a single retailer employing all these best practices. Instead, we encourage you to prioritize the most feasible and impactful development areas from your business’s unique perspective.
1.1 Harnessing the power of AI to optimize the DIY and home improvement supply chain
Today’s retailers collect massive amounts of data on transactions and interactions with consumers both on- and offline. That’s precisely why retail is the perfect match for artificial intelligence (AI), which makes it possible to leverage that data into faster, more accurate decisions. This is an invaluable asset in an industry where retailers must control millions of goods flows and accurately match supply to demand at hundreds or even thousands of locations daily.
Retailers don’t need “AI”—they need to employ several AI algorithms in their analytics toolbox to supplement “old hat” technologies like statistical analysis and rules-based heuristics. Machine learning algorithms, for example, consider hundreds of potential demand-influencing factors when forecasting retail sales—something no human demand planner could ever achieve.
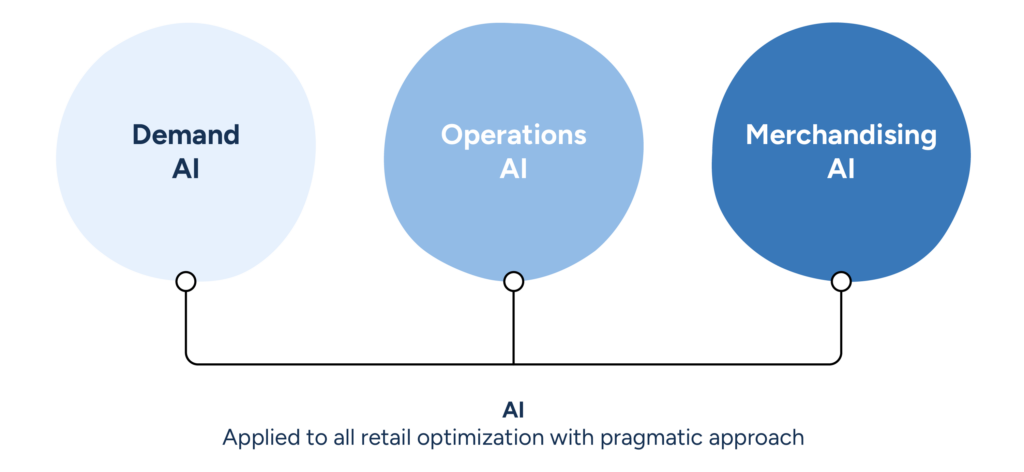
The application of AI in DIY retail is not limited to demand forecasting. Retailers reap even more significant benefits when they leverage AI to optimize the full range of their operations—from inventory optimization to workforce optimization to price and promotion planning.
2. Demand forecasting is the engine running your DIY supply chain
Demand forecasting is the engine that runs your DIY retail supply chain. However, despite the high-quality technology available, many notable DIY retailers have yet to put together a plan to achieve truly data-driven forecasting.
2.1 Granular, data-driven forecasting Is a must for home improvement retailers
Granular forecasting is not just a best practice—it’s a must-have in today’s DIY and home improvement retail space. Without detailed forecasts, it’s impossible to correctly position inventory in the supply chain to maximize sales and minimize costs. Granular forecasts are also the planning foundation for resource and capacity management, so they should be considered a prerequisite for profitable operations.
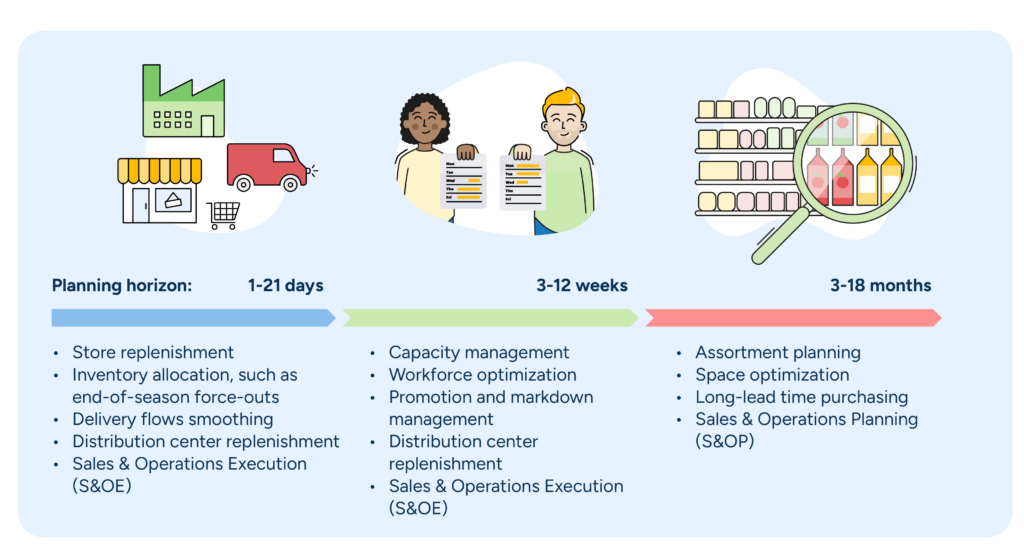
2.2 Machine learning delivers high value in DIY demand forecasting
Although RELEX was ahead of the curve in incorporating machine learning into retail demand forecasting, the practice has become mainstream. Today’s retail technology vendors either already apply machine learning or are rushing to update their legacy systems to offer it.
Machine learning allows a demand forecasting system to learn automatically and improve its predictions using data alone, with no additional programming needed. Because DIY retailers generate enormous amounts of data, machine learning technology quickly proves its value.
Of course, machine learning algorithms aren’t new—they’ve been around for decades. But they have never been able to access as much data or data-processing power as they can today. Though DIY and home improvement retailers may have struggled to update their forecasts quickly, large-scale data processing and in-memory technology now enable millions of forecast calculations within the space of a single minute.
Machine learning makes it possible to incorporate a wide range of demand-influencing factors and relationships into sales forecasts. This is enormously valuable, as weather data alone can consist of hundreds of factors that can impact demand.
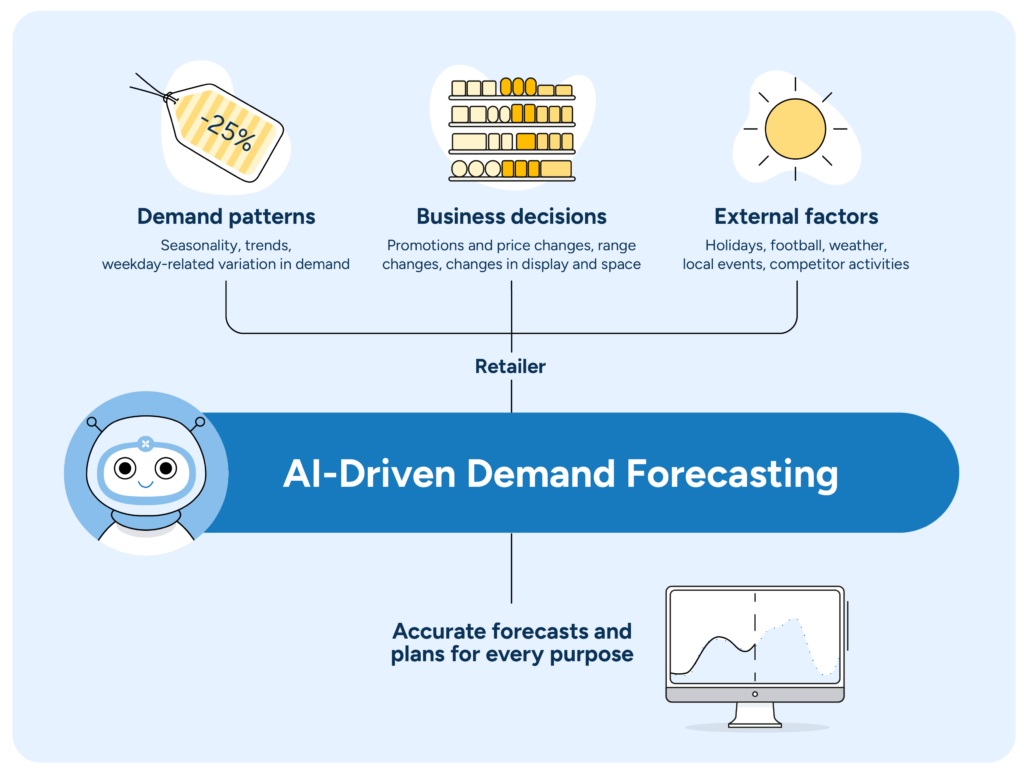
But we should share a word of caution here: it does make a difference how machine learning is implemented. Although DIY retailers can collect massive amounts of data, their data is often limited to the store and SKU levels. Slow-moving products like lawnmowers or washing machines may not generate enough sales transactions to study without aggregation; master data on past promotions and product displays may be lacking; and as products move in and out of range, data may not be available for the exact SKU a retailer is attempting to forecast.
Machine learning forecasting must be set up correctly to make it less vulnerable to data issues, which would cause a “garbage in, garbage out” scenario. Implemented the right way, though, machine learning effectively addresses common challenges with retail data to deliver benefits across all facets of DIY demand forecasting.
Machine learning delivers only equivalent or slightly better accuracy than traditional time series-based demand forecasting in simple scenarios- like forecasting a predictably recurring demand variation. However, machine learning-based forecasting clearly outperforms traditional forecasting approaches when dealing with complex situations like overlapping promotions.
2.3 Typical forecasting challenges for DIY and home improvement retailers
Next, we will discuss how DIY retailers can overcome typical forecasting challenges, including:
- Forecasting slow movers.
- Predicting demand for new products and stores.
- The impact of promotions and price & display changes.
- Improving omnichannel demand forecasting accuracy.
Of course, DIY and home improvement retailers also have to deal with the effects of external factors such as extreme weather events. Still, these complex challenges are difficult to forecast ahead of time and typically need to be addressed through quick reallocation of available inventory.
2.3.1 Forecasting slow movers
With assortments of up to 80,000 items per store, DIY retailers typically deal with a lot of slow-moving products—from high-value power tools and furniture to inexpensive special-fit fasteners that nonetheless play an essential role in a customer’s home improvement project. Slow movers generate very little sales data, and what data does exist is spread out over a long period, so it can be difficult (or impossible) to accurately forecast when an item will sell at the store level. Seasonal patterns or sales trends can also be hard to spot at the store level, even when they substantially impact distribution center inventory requirements or supplier demand.
While store-level forecast accuracy may not be crucial for managing these items, systematic forecast errors can have dire consequences upstream in your supply chain:
- Failing to recognize a downward sales trend will result in costly overstock at your distribution centers.
- Failing to understand seasonal patterns or upward trends in sales will hurt product availability and cause lost sales due to stockouts.
To effectively forecast slow-mover demand across your supply chain, you need a planning system that can analyze data from multiple product locations simultaneously to help identify effects in scarce data situations. You need a system that combines this granular, product-location-day level sales forecasting data with the ability to automatically identify and apply sales patterns across multiple stores within a region or for a product category in a specific store or sales channel.
By taking advantage of a best-in-class planning system, retailers can more accurately forecast and place orders for slow movers with adequate lead time. This approach can ensure that home improvement retailers’ DCs are adequately stocked, that stores are appropriately replenished, and that customers can find the inventory they need when they need it. For example, German DIY retailer Hellweg improved product availability for their slow-moving inventory by implementing a centralized, data-driven demand planning system.
2.3.2 Predicting demand for new products and stores
Because machine learning relies on finding patterns in historical sales data, new products with no historical sales data can prove challenging. Fortunately, routines are also available to improve the management of new product introductions.
When introducing a new product, the most common approach is to assign it a reference product to serve as a blueprint for its sales pattern until it accumulates enough sales data of its own. It’s far more efficient to use a system that can automatically compare product attributes (for example, product group, brand, pack size, color, or price point) to assign the most relevant reference product. You can apply the same approach to find suitable reference stores for new store openings.
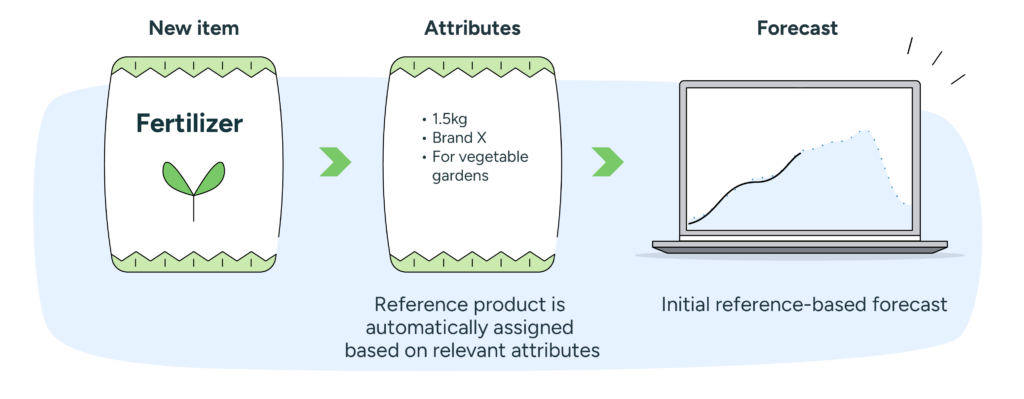
2.3.3 Forecasting the impact of promotions and price & display changes
Your business decisions as a retailer are also an important source of demand variation, from promotions and price changes to how you display products throughout your stores. Although retailers plan and control these changes themselves, many in the DIY retail industry are still incapable of accurately predicting their impact.
Machine learning allows DIY retailers to accurately model a product’s price elasticity or how strongly a price change will affect that product’s demand. However, price elasticity alone doesn’t capture the full impact of a price change.
Often, a product’s pricing in relation to other products in its category also has a significant impact. In many categories, the product with the lowest price captures a disproportionally large share of demand. Machine learning-based demand forecasting makes it quite straightforward to consider a product’s price position, as shown in Figure 6 below.
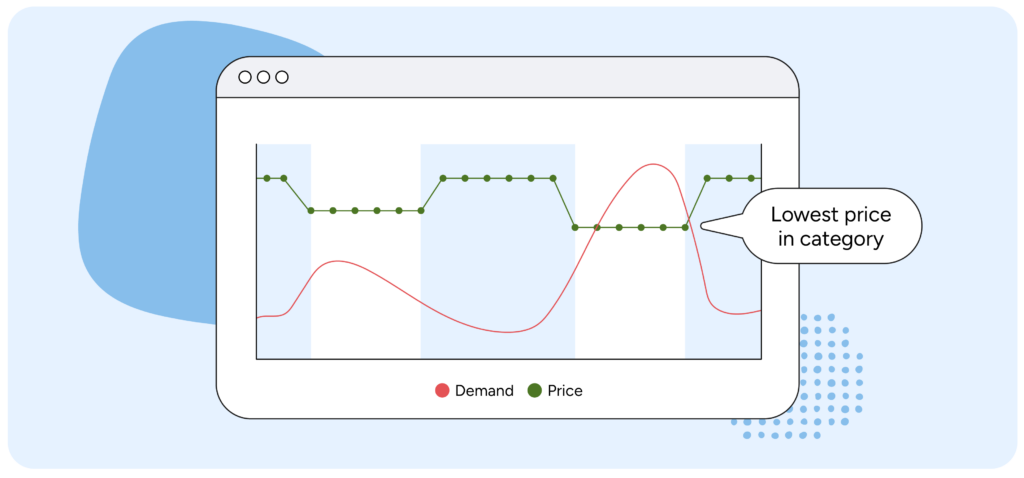
Machine learning does more than leverage price data, though. With machine learning-based forecasting, DIY retailers can accurately predict the impact of promotions by considering factors including, but by no means limited to:
- Promotion types, such as price reduction or multi-buys.
- Marketing activities, such as circular ads or in-store signage.
- Products’ price reductions.
- In-store display, such as presenting the promoted product in an endcap or on a table.
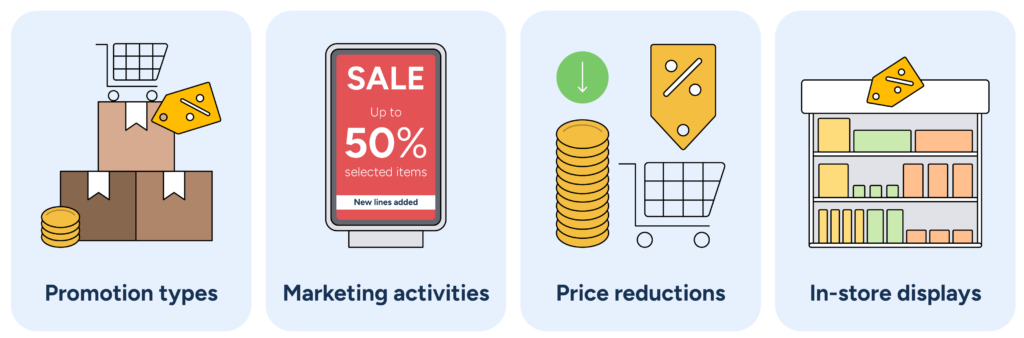
The halo effect is another critical consideration when developing promotions in the DIY and home improvement space. The halo effect is when promoting one product drives sales for related products outside that product’s class. For example, if you promote cordless power tools, you’ll likely see an increase in sales of compatible batteries as well.
Unfortunately, the halo effect’s impact can be so diffused across the assortment that identifying every impacted product becomes essentially impossible, even with machine learning. Think gloves, shovels, lawnmowers, or any number of other items shoppers might associate with gardening. But even if a forecasting system can’t automatically identify all possible halo relationships, it should still make it easy for your expert planners to identify those relationships manually. Then, the system can use that information and past patterns to generate its forecasts automatically.
2.3.4 Managing omnichannel demand in DIY and home improvement retail
As customer demand for online shopping options grows, DIY and home improvement retailers need to manage the complexity of omnichannel demand forecasting and fulfillment. It’s neither cheap nor easy to run omnichannel services, and without careful planning, you risk margin erosion.
Many DIY retailers rely on a single forecast that includes store and online demand. However, reliance on this overly broad forecast to improve accuracy across multiple channels increases the likelihood of inventory issues in both stores and distribution centers, including overstocks and out-of-stocks—particularly when forecasting and ordering long-lead-time products and slow movers. Home improvement retailers seeking to improve online profitability need a supply chain management solution that can accurately forecast each channel’s demand and deliver data-based insights.
It’s imperative to forecast online sales separately from store-based demand. By forecasting online demand separately, DIY retailers can accurately capture the unique demand patterns of online sales and the impact of, for example, promotions prominently featured online. In addition, the ability to predict the volume and timing of online orders enables planning for the right capacity and workforce in picking and dispatching customer orders.
The best omnichannel retailers can make clever use of their different sales channels to minimize risk and maximize revenue for new products and products being phased out. New products can, for example, be launched online first to be included in the physical stores’ assortments if they prove successful. To minimize the risk of customer disappointment while still ensuring stores’ profit margins, down-trending products can be removed from the physical stores’ assortments to make room for better-performing products while remaining available online for their hardcore fans. Without store- and channel-based demand forecasting, transitions like these will be very cumbersome and have a high risk of stockouts or overstock.
Successful forecasting of online demand requires addressing the challenge of backorders and split shipments. Occasionally, online customer orders must be fulfilled using inventory from several distribution centers (“split shipments”). Sometimes, there are delays in fulfillment or customers placing orders for products waiting to be restocked at the DC. To ensure reliable future forecasts, the planning system in use must be able to attribute these orders to the DC from which they would ideally have been fulfilled and for the day they should have been shipped.
A best-in-class system will automate much of this work, relieving the burden on your planners, who otherwise would have to manage multiple channels, suppliers, and delivery patterns manually. A highly transparent, automated system gives DIY retail planners confidence that the right amount of inventory will be sent to all channels at the right time. By increasing forecast accuracy by channel, your company can improve online and in-store operations, customer experience across all channels, and profitability for the overall business.
JYSK shares how RELEX is helping them drive forecast accuracy and streamline planning processes through agile, AI-enabled solutions to better serve their customers
2.4 Data transparency maximizes planner expertise in DIY demand forecasting
DIY and home improvement retailers who hope to remain competitive must adopt machine learning, but you must also understand its limitations. Automating most of your demand forecasting isn’t just desirable—it’s quite feasible with machine learning. However, retailers will always face circumstances –demonstrated by crises such as COVID-19 – where system-generated forecasts are off (although some systems can recover faster).
In a dynamic business like home improvement retail, there is always a risk that forecasts based on how things used to be will fail to accurately capture how things are now or how they will be in the future. No demand planning solution can completely escape forecasting errors, no matter how advanced. That’s why it’s so important that the experts on your demand planning team are able to fully understand forecast errors. If your system provides transparency into how it forms the forecast, your retail experts can quickly understand and correct any errors they see in it.
Too many DIY retailers rely on “black box” forecasting systems that can take in all sorts of data to produce accurate forecasts but lack transparency. Black box systems may kill your business—or at least your planning efficiency—for several reasons.
Occasional extreme forecast errors can inflict far more damage on performance than smaller, more frequent errors. Consider driving on an uneven, bumpy road. Regular, small bumps can be managed with care, but a deep pothole will cause significant damage to the vehicle if you don’t see it coming.
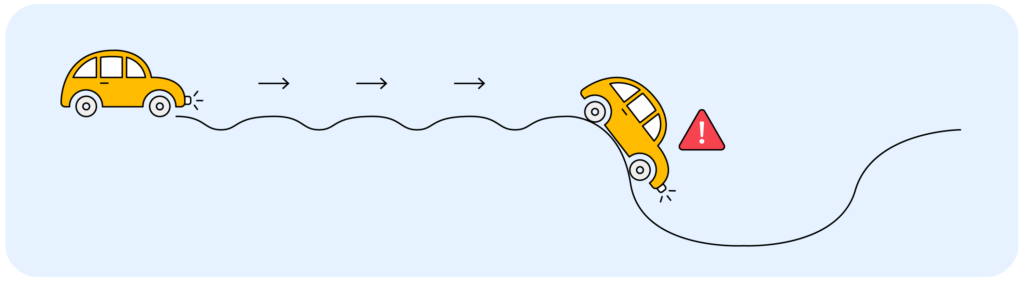
When demand planners can’t make sense of forecast errors, their confidence in all forecast calculations erodes. This leads to increased double-checking and manual forecasting, undermining the entire goal of using computer power to automate forecasting.
That’s why best-practice retailers understand the value of transparency. Even when the system does the heavy lifting, human planners must always be able to understand and control how their forecasts are generated.
3. Automated replenishment improves efficiency and availability throughout the DIY supply chain
3.1 Forecast-based replenishment replaces manual processes with automation
Too many DIY retailers today still rely on their store associates to keep track of availability and demand for thousands of SKUs and manually place orders to keep shelves adequately stocked. This approach is extremely time-consuming, and one of the great benefits of centralizing and automating the process is that it frees your store personnel to focus on delivering high-quality customer service.
Selco Builders Warehouse shares how they have seen significant enhancements in the efficiency of their branches and customer service following their deployment with RELEX Solutions.
However, a manual approach also fails to take advantage of the wealth of supply chain data that could inform and improve those ordering decisions. Home improvement retailers need to take advantage of an automated supply chain management system that uses machine learning-based forecasting to guide replenishment. The system should be able to automatically generate store-level order proposals based on detailed supply chain information on available inventory, incoming goods, expected sales until the order arrives, safety stock requirements, and visual minimums.
High-quality, forecast-driven replenishment delivers consistent benefits:
- Increased revenue from better on-shelf availability, boosting sales by up to 17%.
- Up to 30% reduction in inventory value and 20-day reduction in days of supply with automated and centralized store replenishment.
- Improved capacity management, including more efficient store and DC capacity utilization and up to a 30% reduction in peak deliveries to stores.
3.1.1 Leveling goods flows to maximize in-store capacity at peak times
DIY retailers commonly see significant delivery peaks at certain times of the week. These peaks pose considerable capacity challenges, from DC picking and transportation to store receiving and shelving.
However, Figure 8 (below) shows how a best-in-class planning system autonomously smooths out the flow of goods in your distribution centers and stores, reducing the risk of bottlenecks and ensuring efficient capacity utilization.
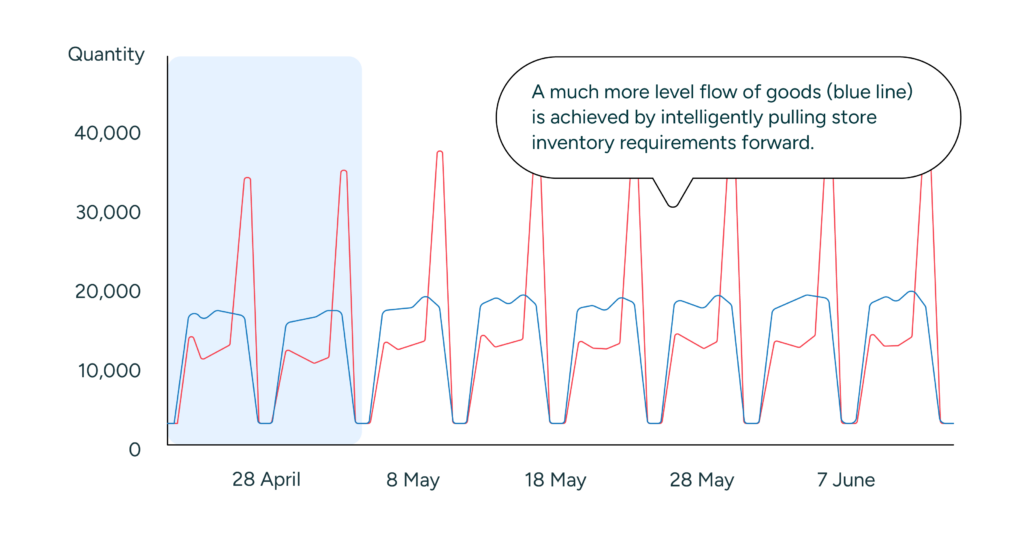
Granngården, one of Sweden’s largest retailers of pet and gardening products, has driven significant improvements to their goods flow and overall capacity management processes using a system that manages their delivery flow smoothing. With proactive visibility into capacity issues throughout their supply chain, they can automatically smooth resource needs across the week, allowing their store staff to focus on customer service on peak days. The stores with the most significant capacity challenges were able to reduce weekend deliveries from 70% to only 48% while maintaining both availability and safety stock.
3.1.2 Optimizing orders to fill up trucks and meet supplier order limits
Along with leveling the flow of goods, you must also be able to consolidate your ordering for maximum efficiency. It may seem simple, but pooling orders for multiple products to fill load carriers or meet supplier order limits can test your planning system’s flexibility.
DIY retailers need several capabilities to meet supplier requirements and benefit from lower transportation costs or supplier discounts without accumulating excess stock:
Flexibly define which products should be pooled when planning an order. Products from the same supplier are often pooled together. Still, sometimes it makes sense to consider the same supplier’s different manufacturing sites separately or to consider all products sourced from the same region, regardless of supplier, as one group.
Set targets and limits for the consolidated order in multiple units, such as value, volume, number of pallets, weight, or combinations of these dimensions. For example, when filling trucks, the order should fill the available space efficiently without you paying to transport air or exceeding the maximum legal weight.
Let the planning system decide which kind of load carrier it should aim to fill up with the order. With some suppliers, it may make sense to order a truck with a trailer or one with a trailer and one without, depending on the forecasted demand.
Set the right order trigger level. When supplier order restrictions are difficult to meet, it may make sense to require enough demand for at least 30% of a truckload, for example, before the planning system starts building an order that fills up the entire load carrier.
Building and home improvement products company K Group took advantage of their planning system to significantly reduce the time it takes to calculate orders despite typical small-volume orders and complicated supplier ordering restrictions. This new way of ordering requires less time and fewer personnel to make purchasing decisions.
3.1.3 Managing inventory levels for slow movers
Slow-moving items are essential to DIY retail and make up a considerable portion of most product ranges, but they can be challenging to manage due to their unpredictable nature. In many cases, you may want to keep a certain amount of a slow-moving item in stock to maintain the brand image, even if demand doesn’t necessarily justify the quantity. These items generate the least sales data across the longest periods but must be replenished to meet your visual minimums.
Some slow movers are typically purchased in large quantities rather than as single units. For example, a consumer rarely purchases a single light switch or just one tile. However, a builder may need a few dozen light switches or boxes of tiles to complete a project. Further, in both examples, all the items need to be identical. Other items, such as table legs, must be kept in specific quantities because they are typically purchased as a set.
For such items’ inventory levels to be successfully managed, safety stocks need to move up and down in step with expected unit sales per transaction at the product-store level. By analyzing how a specific item has typically sold in the past and in what quantities, you can determine the ideal level of availability and trigger distribution centers to fulfill inventory to maintain that minimum without risking overstocked or obsolete inventory.
3.1.4 Sync replenishment and space management for cost-efficient operations
Traditionally, retailers have operated in a very siloed manner, with very little communication between the merchandising teams responsible for store planograms, the supply chain teams responsible for store replenishment, and the store operations teams responsible for in-store work processes. Keeping these teams in sync in the large-format stores typical for DIY retail is essential to ensure inefficient activities aren’t wasting time and money.
The space allocated to each product in a store has a significant impact on both the results and costs of the store replenishment process:
- If the allocated space is larger than demand, the inventory needed to ensure optimal availability won’t be enough to maintain full, appealing displays. In that case, you need to define additional visual minimums that indicate how many units should be on shelves to ensure appealing displays. For slow sellers, visual minimums will always be higher than what’s strictly needed for high availability.
- If the allocated space is smaller than demand, incoming deliveries won’t fit on your shelves, and you’ll have to store at least part of the delivered quantity in a backroom. This significantly increases the cost of shelf stacking because store personnel will need to move goods back and forth between the sales floor and the backroom. Backroom storage also significantly increases the risk of empty shelves, as timely replenishment from the backroom depends on the vigilance of store personnel.
Full integration between space and replenishment planning is an essential best practice for increased operational efficiency. Retailers with this level of integration can:
- Easily automate the maintenance of visual minimums on the product-store level based on the number of facings or total shelf space allocated to each product in each store.
- Automatically trim replenishment orders that would cause incoming deliveries not to fit on the shelf. This, of course, must be balanced with the risk of stockouts if the space allocated to some products is very small compared to demand.
- Plan replenishment so that shelves are fully filled upon each store delivery, minimizing shelving work. Rather than getting two batches in one go, if there is space for a third one to be delivered next week, the order is calculated to fill the assigned shelf space upon arrival.
- Assign main replenishment days based on where products are displayed in the store to create more focused deliveries that minimize the need for store personnel to unnecessarily move around the store when stacking shelves.
The space assigned to each product is vitally important to the efficiency of your replenishment process. Good analytics tools can identify products and stores with space and sales mismatches.
3.1.5 Managing green life items
For many DIY retailers, plants and flowers are a large part of their offerings, and 62% of retailer respondents in a recent survey reported garden center sales of more than $1M in 2022 (up from 53% in 2020) and 29% reported sales over $5M. With substantial revenue at stake, DIY and home improvement retailers must consider a few key elements to manage their plants and garden center products.
For example, availability and quality depend on growers’ output. For manufactured goods, suppliers hold stock and fulfill orders as they come in. With grown, live products, though, supplier availability can be unpredictable and driven by weather to a high degree. The actual quality and volume of available product inform what can be sent to stores. DIY retailers can turn to a pick-to-zero strategy: supplier orders are based on stores’ replenishment needs, but rather than fixing the amounts to be delivered to each store, you reallocate the supplier delivery to stores upon receipt based on the latest inventory and forecast information.
Due to uncertain supply, DIY retailers often have to split replenishment orders between multiple vendors to ensure availability. In that case, the planning system also needs to allocate the order need to several vendors. For example, 65% to GreenGrowers Co. and 35% to OrganicFarmers Co. Though green life product management can be highly laborious to manage manually, it can be automated effectively. The critical prerequisite is clear guidelines for which products should be included in stores’ assortments and which vendors should be used for sourcing at any given time. As in any automation process, high-quality master data is essential.
Plantagen shares their success with their recent expansion with RELEX to streamline their ordering and replenishment and drive efficiency for store teams.
Furthermore, green life products have a high level of substitutability. For shoppers, a tulip is simply a tulip, but your supply chain system may have tens of different product codes for “tulip,” each associated with a different vendor. A system capable of switching between planning levels is crucial for products with high substitutability. Your planning system must be able to forecast overall tulip demand and keep track of on-hand inventory at the product level to determine replenishment quantity accurately. However, the actual replenishment orders need to be on the SKU level—for example, “Tulip supplied by GreenGrowers Co.”
Finally, efficiency depends on understanding the capacity for orders. DIY and garden centers don’t typically use traditional pallets or roll cages for transporting plants and green life products. Instead, they use unique Danish trolleys, which have their own dimensions and optimal capacity requirements. Efficient store replenishment requires understanding not only the specific trolley but also the sizes and volumes of the plants going to each store to maximize each delivery. A system that can intelligently calculate that fill helps your planners make accurate purchasing decisions while reducing costs.
3.2 Centralized and responsive replenishment drives seasonal efficiency
Many variables can impact your seasonal inventory needs, so DIY retailers need a replenishment solution that can optimize seasonal products’ availability while reducing residual stock at season’s end. This strategy results in increased sales, reduced costs, and higher profitability, transforming seasonal replenishment from a problem into an opportunity.
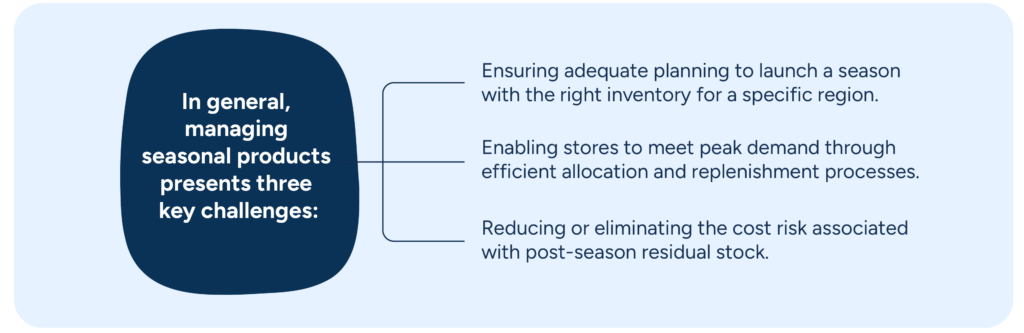
Using an automated replenishment solution, DIY retailers can seamlessly combine pre-season ordering, initial allocations, automatic in-season replenishment, and targeted end-of-season allocations and markdowns to drive maximum value from seasonal stock.
An innovative, responsive replenishment strategy uses store-specific sales data to decide how to allocate seasonal products to stores. A good system can automatically consider the seasonal pattern for a product or product group at each store in your network, teasing out regional and store-level variations and basing distribution on either product- and store-specific forecasts or, if those aren’t available, on the store-specific historical sales for the given product segment.
But you also need a centralized approach. If you ship the entire season’s stock for an item to all stores at once, then the only way you can respond to actual sales is to transfer goods between stores—an inefficient, labor-intensive, and expensive approach. The best solution for handling large amounts of seasonal items is what is sometimes called a push-pull-push strategy.
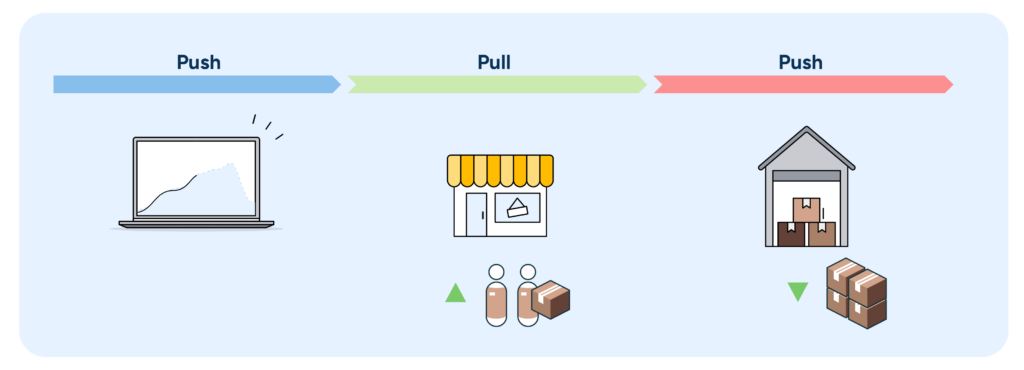
PUSH: Using the forecast and historical sales data, retailers can estimate when the season will start for their various regions. While local conditions such as weather fluctuations can be unpredictable, effective use of data enables an agile response when needed. You can then determine a deadline for when the stock needs to be received in time to prepare for your first sales push. Product is allocated— “pushed”—to stores in sufficient quantities to build displays and meet the initial demand when the season begins. The rest of the products for the season are kept in a central location. After that initial sales spike, you switch to the “pull” stage to replenish stores as sales occur.
PULL: As sales pick up, replenish stock levels at your individual stores through the “pull” stage based on the latest store-specific sales data and inventory levels. This enables you to respond far more effectively to local conditions, such as variations in the weather or a change in competition for an individual store. Effective use of the “pull” control ensures that supply is channeled to the right stores at the right time to meet demand, preventing a scenario in which some stores face shortages while others struggle with excessive amounts. This also prevents the need for costly store-to-store inventory transfers.
PUSH: When sales begin to slow, the next “push” phase goes into effect. This is the time to start clearing the remaining product out of your central warehouses. You should determine when and where to allocate residual stock using continued analysis of store-level sales data, ensuring product goes to stores with the highest sales and no excess inventory. An effective system will also help you plan markdowns to optimize profits, especially for items that may fall out of trend and sell poorly next season. You should base this stage’s execution on your team’s analysis of location-specific conditions, so remaining stock is pushed to stores with the highest likelihood of sales.
The push-pull-push strategy is very effective when used with historical sales analysis and an accurate forecast. While weather can be unpredictable, the forecast will reflect typical seasonal characteristics for each region and location, providing the basis to plan purchasing timing and allocation decisions.
Product lead times are also critical for seasonal purchasing and allocation planning. When lead times are long, limiting you to a fixed amount of available stock for the entire season, the value of that inventory needs to be maximized. However, suppose lead times are short, and availability is high throughout the season. In that case, it’s sufficient to push only the amount of product needed for stores to build displays and meet the initial sales peak, then replenish in accordance with actual sales.
4. Customer demand drives the end-to-end DIY supply chain
4.1 In the integrated DIY supply chain, distribution centers are driven by forecasts across all channels
Traditionally, DIY retailers have treated store replenishment and inventory management at regional distribution centers or central warehouses as separate processes driven by separate demand forecasts. However, this approach risks visibility gaps that often lead to costly errors, especially when dealing with bulky and seasonal items.
The best practice is to base distribution center forecasting on stores’ projected orders, reflecting both pull-based demand and planned, push-based stock movements.
There are, however, incremental challenges to consider when using store demand forecasts to drive planning at your distribution centers:
Before you can sell your goods, they must be delivered to stores and managed efficiently there. You must sync distribution center and store demand forecasts to account for peak sales and delivery times. The difference in timing depends on your stores’ sell-through rates and replenishment schedules, which can vary between stores, products, and days. Rapid changes at the store level mean bad news for DC forecast accuracy. However, complete visibility across the supply chain combined with goods flow smoothing increases accuracy and combats capacity issues with peak-time deliveries.
When you push rather than pull goods through the supply chain, DC delivery peaks aren’t visible in store forecasts. This is most evident with seasonal and promotional items, where you must push inventory uplift to stores in advance, creating a significant DC demand peak. You control this process, but DC supply planners face a lot of pressure to anticipate when and in what quantities stores will take in products. Effective automation in your planning process optimizes allocation efforts, reduces manual planning, and mitigates the risk inherent in disconnected systems.
For your system to achieve seamless integration of store and distribution planning, it must be able to calculate projected orders per product, store, and day several months—up to a year—in advance. It should then process those calculations, considering current and known future replenishment parameters as well as the demand forecast.
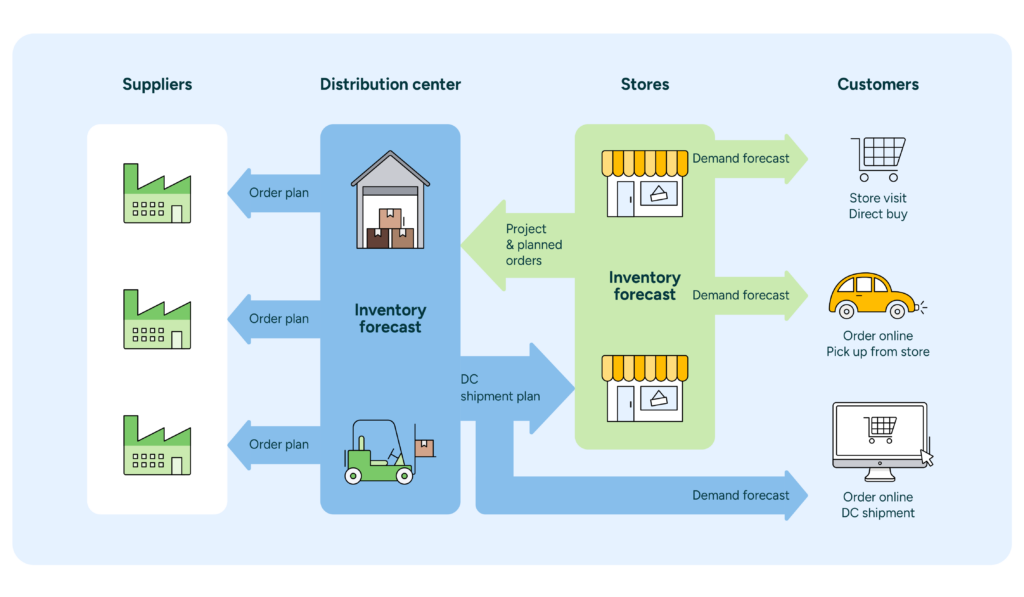
In practice, the stores’ order projections should consolidate a range of data on:
- Current inventory.
- Safety stocks and visual minimums.
- Delivery schedules.
Planned inventory movements, including stocking up to build promotional displays and shifting orders to level out the capacity requirements in distribution.
So, what are some examples of situations where projected store orders, rather than stores’ demand forecasts, enable more accurate DC planning?
Product introductions: When a new product is introduced into the range, the system pushes enough product to at least meet the set visual minimums to each store, creating inventory buffers that can take stores days or weeks to digest. As long as you have surplus inventory in stores, the projected store orders (as well as the actual outflow from your distribution centers) will be lower than forecasted consumer demand.
Product terminations: When your distribution center forecast is based on projected store orders, it automatically considers stores’ existing inventory buffers and accurately estimates how long each store will take to clear the remaining stock. If you’re planning a product termination, the distribution center forecast will reduce automatically as the termination date draws closer, supporting a controlled inventory ramp-down.
Promotions: Typically, retailers push anywhere from 30–100% of their expected promotional uplift to stores in advance. Because these planned inventory movements are entirely within the retailer’s control, they are predictable and will automatically be included in the projected store orders. Further, if the stores are left under or overstocked following the promotion, the system will accurately reflect their fulfillment needs in distribution center forecasts.
Seasons: Distribution centers almost always send some buffer stock to stores before the start of a major season. This helps support the need to build nice seasonal displays in stores, level out seasonal peak volumes, or plan proactively for weather impacts so you’re prepared even if a season starts early. As with promotions, these planned stock movements should be automatically visible in stores’ projected orders and used as the forecast for distribution centers. Because factors like local weather drive seasonal demand variation between stores, stores consume their inventory buffers at different paces. These store-to-store variations should also be automatically visible in distribution center forecasts.
Changes in replenishment schedules: Retailers frequently change stores’ replenishment schedules either temporarily (possibly to match increased demand in the high season) or permanently (for example, after implementing new transportation routes). Of course, changes in the replenishment schedule won’t impact consumer demand, but they’ll undoubtedly impact the goods flow into stores. When it’s based on projected store orders, your distribution center forecast should automatically capture the resulting changes to the timing and size of store deliveries.
4.1.1 Applying the best model for effective slow mover planning
When you aggregate projected orders across stores, your distribution centers can form a very accurate, customer-driven forecast for supplying those stores. However, this approach may introduce systematic bias to the upstream forecast for very slow-moving products.
For example, a slow mover sells an average of 0.1 units per week. When a store sells an additional product unit, the inventory balance will drop low enough to trigger a replenishment order that, based on the forecast, is projected to happen in ten weeks. But because demand for this slow mover is essentially random, the store is equally likely to sell that order-triggering unit next week, three weeks from now, or ten weeks from now. This means the order projections systematically underestimate near-term demand for slow movers, especially when aggregated across several stores with similar demand patterns.
To distribute the projected replenishment orders for your slow-moving products more realistically over time, you can apply a modeling approach called “Monte Carlo sampling.” From an individual store’s perspective, there’s no difference; the store still needs to carry the same amount of safety stock to ensure availability. However, at the distribution center level, this approach significantly improves the forecast accuracy of slow movers.
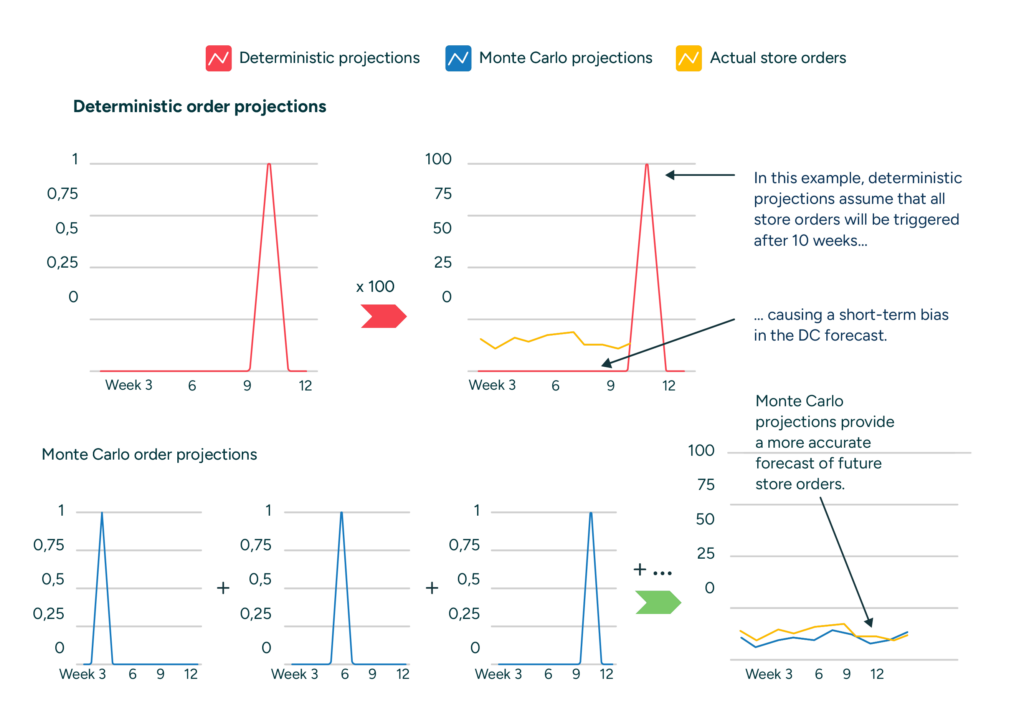
4.2 Integration drives end-to-end DIY supply chain execution from a single plan
Large-scale retailers need to be able to instantly see and measure the impact of decisions made in one function across all other functions. This is particularly important for DIY and home improvement retailers, who must manage extensive ranges while considering diverse regional and seasonal demands.
Leroy Merlin Italy explains how a centralized planning approach using RELEX is helping his team improve forecasting in the short term and in the medium-long term.
When you base distribution center planning on stores’ projected orders, you can immediately see the impact of planned activities like promotions or preseason allocations throughout the entire supply chain. For the system to reap the full benefits of this transparency, it must have access to all planning data as soon as the appropriate team has made a seasonal plan, range change, or other relevant decisions.
Time-dependent master data is a critical enabler of proactive planning. We’ve touched on several reasons why accurate timing is vital for DIY retailers. Below are examples illustrating how systems that support time-dependent master data enable your teams to share valuable information immediately as it becomes available. This, in turn, allows replenishment planners to reduce manual work by relying on the system to trigger necessary actions automatically.
- Time-dependent replenishment schedules: When the planning system can manage several replenishment schedules linked to specific dates, it can automatically consider future changes in replenishment schedules in its supply chain projections.
- Assortment activation and termination dates: Product ramp-ups and ramp-downs are much easier to manage when start and end dates for the active product range have been defined. You can automate routine planning tasks, such as pipeline fills for new products or inventory ramp-downs for discontinued products, reducing manual work while ensuring optimal inventory levels in all product lifecycle phases.
- Seasonal push-pull-push: Seasons naturally have start and end dates, but you must be able to specify beforehand how stores should be stocked during those seasons. Rules and templates make it possible to build accurate replenishment plans for each store and product without extensive manual work.
- Temporary supplier delivery restrictions: Chinese manufacturers, for example, often won’t dispatch shipments during the Lunar New Year. If you make information about restrictions like this available to your planning system, it can automatically adjust orders early enough to ensure high availability during the affected period.
An integrated supply chain setup removes the need for double-planning work. The impacts of planned changes to store replenishment are automatically reflected in the projected store orders that inform distribution center demand forecasts.
For example, if you’re planning a new promotion, your promotional forecast will impact the amount of product that should be sent to each store. Additionally, you may have a strategy in place outlining that distribution centers should push 50% of the forecasted demand to stores a couple of days before the promotion launches to ensure store associates have time to build promotional displays. As soon as the promotion plan is made within the system, the relevant information is immediately visible in the distribution center forecast as well, allowing them to deliver goods to stores on the right dates and in the right quantities.
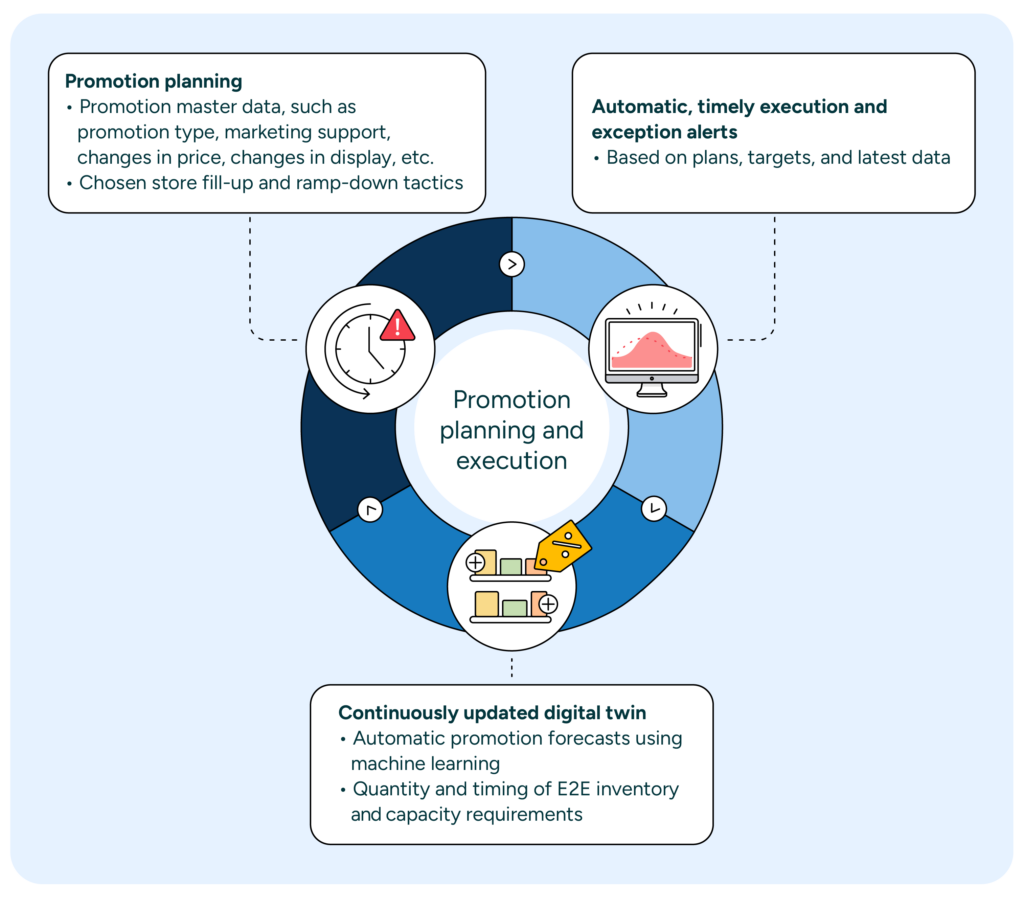
Norwegian gardening retailer Felleskjøpet Agri, who had been relying on manual processes executed by store managers, automated and centralized their supply chain processes, providing demand forecasting, automatic replenishment, allocations, and integrated supply chain planning for their 96 stores and one distribution center. After implementation, Felleskjøpet Agri saw a 7.8% improvement in on-shelf availability, a 33% inventory reduction, and a 20-day reduction in days of supply.
4.3 Multi-echelon optimization of goods flows
For DIY retailers who manage a wide product range from various suppliers, optimizing your multi-echelon inventory flows with a high level of automation is vital for cost control. Increased supply chain control and visibility enable DIY retailers to automate several important distribution tactics, such as virtual ringfencing, cross-docking, and scarcity allocations.
The most efficient way to meet both store and online demand is to fulfill orders from the same centrally held pool of stock in your distribution centers. The best practice to ensure availability across all channels is to create a virtual ringfence around part of the stock in your distribution center that’s earmarked for online sales.
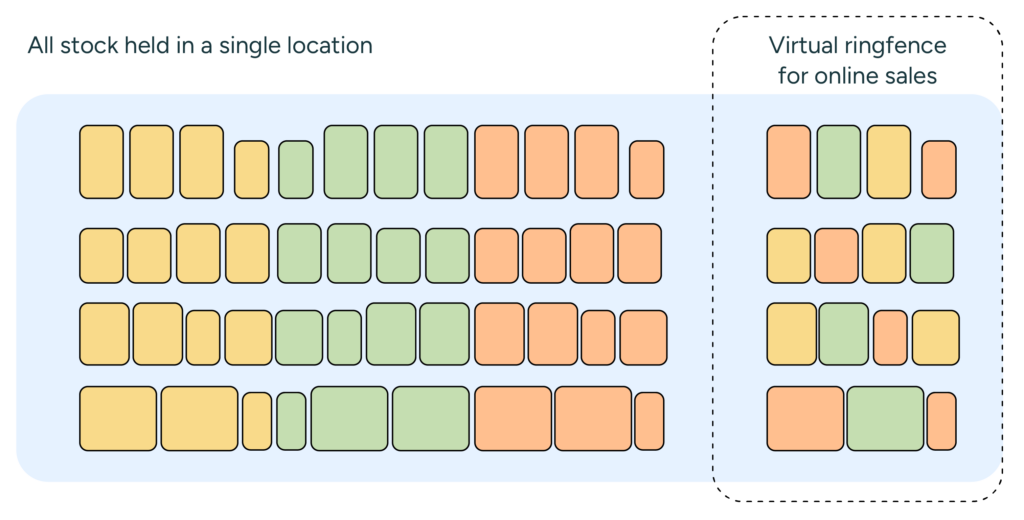
With virtual ringfencing, the system “reserves” the portion of your centrally held stock allocated to online demand, ensuring stores can’t draw from it to fulfill store demand. It’s easy and efficient to send more stock to stores as the season progresses, but recalling goods from stores to distribution centers is always expensive and best avoided whenever possible.
This approach to allocation is executed best when based on continuously updated channel-level forecasts. The starting point for online inventory before or at the start of a season may be crude since you don’t know how the item will sell in each channel yet. However, as you gather sales data, the system will update the forecast and drive the split between channels. As the season’s end nears, you can slowly ramp down the ringfenced amount to minimize excess end-of-season stock.
Ringfencing also enables your planners to deploy different strategies in different situations. For example, you can prioritize channels differently depending on the available supply—some retailers might want to maintain 100% online availability even if that means stores experience stockouts. To manage the process well, your system needs a good exception management process to ensure that all changes in demand are measured and accurately drive the actions necessary to meet your business’s goals.
Whereas ringfencing helps streamline omnichannel distribution, cross-docking can help DIY retailers streamline the distribution of bulky products. This approach is effective when managing items so large that storage and subsequent redistribution are capacity-restrictive, or items that need to be directly allocated to stores rather than held centrally. This strategy could be effective for managing a number of DIY and home improvement products, including building supplies, garden and lawn equipment, and appliances.
There are some requirements for cross-docking to work efficiently:
- Suppliers need to be able to deliver full truckloads to the cross-docking facilities.
- Delivery units, such as pallets or roll cages, must be ready for immediate movement to outbound trucks without additional handling.
- Outbound trucks must maintain high fill rates to keep transportation costs down.
To support cross-docking, your inventory management system needs to understand both inbound lead time to the cross-docking facility as well as outbound lead time to trigger replenishment orders at the right time.
When supply chain planning is fully integrated, your planners can resolve exceptions optimally and efficiently. For example, an integrated supply chain planning system can automatically trigger optimized scarcity allocations if an incoming shipment is delayed.
Instead of fulfilling store orders on a “first come, first served” basis, the right system can automatically allocate available inventory to stores to maximize overall on-shelf availability or in accordance with strategic store prioritization. In the best-case scenario, on-shelf availability is not even affected. Similarly, you can proactively push inventory approaching the end of a season or promotion to the stores the system identifies as having the best chance of selling the products at full price.
5. Efficient distribution center buying in DIY retail
Replenishing central warehouses and distribution centers is sometimes seen as more of an art than a science. It’s true that longer lead times (especially for overseas orders), a lack of control over external suppliers, and global systemic disruptions can introduce complexities. But at least in principle, replenishing central warehouses or distribution centers is not that different from replenishing stores.
Home improvement retailers can optimise order fulfilment when replenishing stores from their own distribution centers as they find the best. When ordering goods from suppliers, though, there may be complex restrictions regarding minimum order values or quantities, or volume-based discounts or other rebates that can significantly impact margins when utilized efficiently. Many retailers cannot enter this kind of supplier contract or price information into their planning systems, forcing their operative buyers to invest considerable time double-checking orders.
Active goods flows (combinations of products and stores) for larger DIY retailers replenishing their physical stores are typically measured in millions or tens of millions, which means that automation is crucial. For central and regional warehouses, the number of order lines is much smaller, and the value per order line is much higher, making the economic impact of each order line more pronounced. This has enabled and encouraged a lower degree of automation in operative buying compared to store replenishment.
We have found that setting up operative buying processes in a structured way with good system support can also result in very high levels of automation at the DC level. However, this does not necessarily mean that best-practice DIY retailers have a significantly leaner buying team. A key result of automating routine tasks is that operative buyers have more time to:
- Proactively deal with potential capacity, delivery, or quality issues.
- Analyze the performance of the current assortment, suppliers, and supplier agreements for continuous improvements.
5.1 Managing long lead-time supply and using alternative suppliers
When dealing with international suppliers and long lead times, there is always a risk of supply chain disruptions caused by, for example, political events, container shortages, natural disasters, or port congestion. Identifying a secondary (typically local) supplier for long lead-time products that might experience delays or disruptions alleviates some risks of working with international lead times.
A DIY retailer can plan for the regular replenishment to come from the usual international supplier—typically the lowest cost option—with an alternate supplier established to guard against delays. In this instance, it can be more cost-effective to place a small order from the secondary supplier, which may have a higher cost, than to empty your safety stock.
There are also some disruptions, like the annual Lunar New Year celebrations throughout much of Asia, that are entirely predictable. DIY retailers should have a proactive strategy for managing any supply chain disruption you can predict.
The Chinese New Year celebration causes patterned, annual factory shutdowns in China that affect the availability of long-lead products in the first quarter of every year. Shutdowns for the Lunar New Year begin in January and continue through February, with factories not returning to full working capacity until weeks after the holiday.
To accommodate these closures, you should begin planning for the holiday as early as June/July. Many Chinese suppliers cut off buying opportunities around September/October. Capacity smoothing can help alleviate the need to buy all of your long lead products at once by slowly building up stock over multiple shipments before the shutdowns.
5.2 Smart buying takes advantage of good prices
The cost of goods sold dominates retail costs. The operative buying team must be responsible for efficiently exploiting rebates to improve gross margins.
In theory, it’s pretty straightforward to make smart buying decisions when prices are changing:
- When you know a product’s price will increase, stock up just before the price increase.
- When you know a product’s price will decrease, only order the quantity you absolutely need before the price change. Then, stock up after the new price has come into effect.
- When a price is lowered temporarily (due to a supplier campaign, for example), order less just before the price reduction and stock up when the price is low.
To truly benefit from price changes, DIY retailers must factor in inventory carrying cost and time orders correctly and potentially split the investment buy—the additional quantity being purchased above what is needed to meet demand—into several orders.
Furthermore, in situations where storage space is scarce, the inventory cost may suddenly jump to a whole new level if you exceed the capacity limits of your current warehouses. Suppose you fill or exceed your storage capacity. In that case, you’ll need to find and pay for additional space outside your current warehouses for additional goods, quickly turning your investment buy into a highly unprofitable move.
The best practice is to feed your planning system time-dependent price data so it can optimize when and in what quantities to make purchases relative to price changes. This allows you to effectively take advantage of even minor price changes, as operative buyers won’t have to spend time manually figuring out optimal order quantities. With this strategy, it’s critical to consider restrictions, such as shelf life for perishable items or capacity limits on storage space. If your planning system cannot deal with such restrictions automatically, your buying team will have to double-check your suggested investment buys.
It’s not unusual for supplier contracts to include a rebate that’s triggered when the buyer’s annual order value exceeds a set quota. Again, keeping track of supplier quotas, placed orders, and forecasted orders is very hard to do manually. Intelligent planning systems support smart buying decisions by suggesting additional orders to earn rebates when feasible without adding orders that would result in counterproductive stockpiling.
6. Planning for optimal capacity and resource utilization throughout the DIY and home improvement supply chain
In a dynamic business like retail, capacity bottlenecks can emerge in almost any part of the supply chain in response to a range of events, from holidays or unusual weather to promotions or significant assortment updates in stores.
Home improvement retailers need to understand how the forecasted demand will impact inventory, capacity, and resource requirements throughout their supply chains to identify and proactively resolve these bottlenecks.
6.1 Retail Sales & Operations Execution (S&OE)
The S&OE process aims to ensure that retailers can fulfill short-term demand for the upcoming 0–3 months as cost-effectively as possible. The starting point is a very granular demand forecast at the SKU-channel-day level. From there, your planners can use supply chain projections to get a detailed understanding of inventory, capacity, and resource requirements throughout your supply chain.
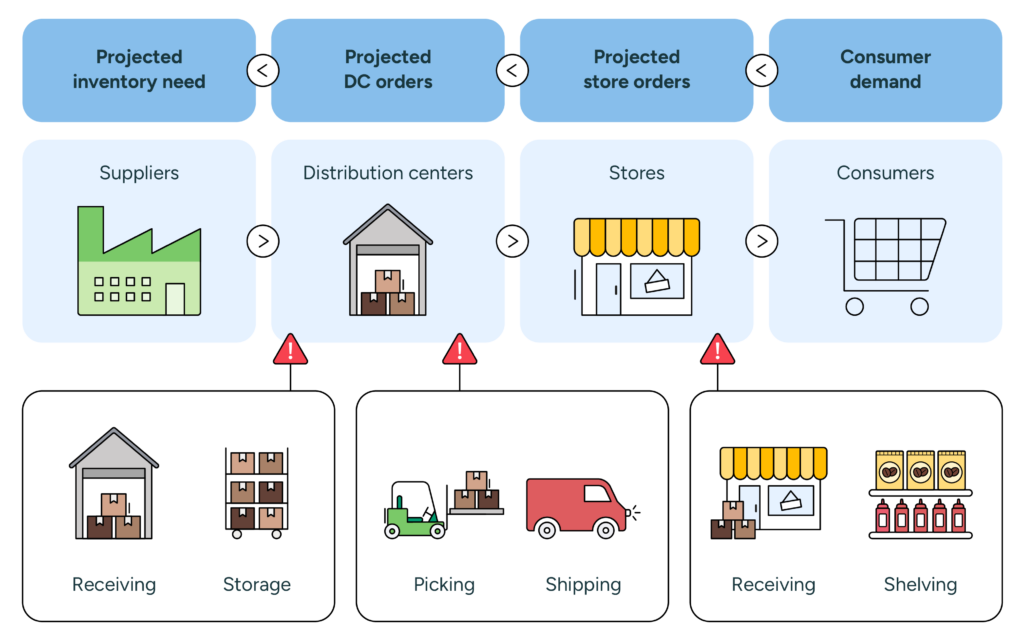
This end-to-end visibility into retail operations drives many S&OE benefits, including:
- Cross-functional alignment: Visibility makes the impact of business decisions made by one function instantly available across all functions to be accounted for in their planning. For example, the expected impact of a planned promotion is immediately reflected in all local demand forecasts as well as inventory and resource projections throughout the supply chain. This means business decisions like promotions only need to be planned once for automatic execution.
- Proactive exception management: With visibility into current and future inventory, capacity, and resource requirements throughout the supply chain, the system can automatically detect potential bottlenecks and help your planners prevent or quickly resolve them. For example, suppose the system alerts planners that an upcoming promotional stock-up combined with seasonal allocations will create a substantial delivery peak. In that case, they can proactively manage the problem before it starts congesting store backrooms or exhausting DC picking capacity.
- Effective contingency planning: With digital twin modeling, retailers can compare and better understand how different planning scenarios will impact their supply chain. For example, suppose one of your sales regions is outgrowing its fulfillment capacity. In that case, your distribution planning team can easily model when and how to shift fulfillment for some of this regional demand to another fulfillment center, effectively balancing capacity requirements across your distribution network.
When dealing with millions of goods flows, tens of thousands of employees, hundreds of vendors, frequent promotions, and regular price and assortment changes, there are bound to be exceptions to any retailer’s plans. Many of these exceptions require immediate attention and quick adjustments to avoid or minimize any negative impact on S&OE.
However, by turning to AI and advanced optimization, DIY retailers can resolve most of these deviations without any human intervention. Best-in-class, autonomous issue resolution increases the speed and accuracy with which you can manage exceptions by:
- Autonomously rebalancing inventory in the supply chain: Trigger optimized scarcity allocations and force-outs in line with your business priorities; re-optimize store orders for plans on the fly in accordance with a pick-to-zero fulfillment approach; or automatically build up inventory levels before periods when a supplier won’t be able to fulfill orders, such as around the Chinese New Year.
- Ensuring throughput and efficient use of capacity: Proactively smoothing out fluctuations in goods flows; continuously optimizing replenishment and space to reduce in-store goods handling; and optimizing workforce deployment using continuously updated workload forecasts.
- Recommending corrective or “next best” actions: Automatically suggesting optimal markdowns to clear excess stock from the supply chain; suggesting supplementary order placements with domestic suppliers to avoid stockouts from higher-than-planned demand when international suppliers’ lead times are long.
The best-performing retailers are able to combine human expertise with technology, quickly adapting to new situations and implementing new business priorities at scale. Consider managing extreme weather events like hurricanes or even the COVID-19 pandemic, which are examples of events so unique and unpredictable that not even the most advanced forecasting systems could predict their impact in advance. In situations like this, your planning team should be able to leverage the system to enable smart decisions based on their invaluable human expertise.
6.2 Retail Sales & Operations Planning (S&OP)
If the goal of retail S&OE is to resolve unforeseen capacity and resource challenges in the short term, then retail S&OP, by contrast, looks further into the future. The goal of retail S&OP is to ensure sufficient capacity and resources to support future growth targets, planned changes in the distribution network, major seasons, and more.
But the retail S&OP process is about more than supply chain efficiency alone—it’s about maximizing profitability. S&OP should result in:
- Goods flows that match, rather than exceed, capacity throughout the supply chain secure reliable supply, which in turn prevents lost sales from delivery problems.
- Cost-effective operations, with costly overtime minimized in all parts of the supply chain.
- Informed decisions that still support your profitability when capacity restrictions prevent guaranteed availability for all products.
- Full transparency into resource requirements, making it possible to ensure that all resources, including store workforce availability, are based on the same operational plan.
Preparing for major seasons provides an important use case for retail S&OP. For example, the critical (and complicated) seasonal shift from winter to spring increases consumer focus on yard care and outdoor spaces. This requires retailers to source related goods well ahead of the season itself to ensure that their stores and distribution centers will be stocked in preparation for a prominent sales peak, the timing of which is often weather-driven and, therefore, unpredictable.
Preparations for a major season usually start 9–15 months before the season begins, when retailers set sales goals and define their seasonal assortment accordingly. You must then determine the number of goods you have to source to match that sales plan and when to order them and deliver them to stores and distribution centers to stock up before the season starts. You also need to be able to adapt flexibly if a season starts earlier than usual.
Because purchase volumes are high and bulkier goods require a lot of storage space, home improvement retailers must carefully plan when goods should arrive in their distribution centers. You should also consider whether a portion of your seasonal goods can bypass distribution centers entirely through vendor-to-store delivery and plan how much additional warehousing space you may need to rent to deal with peak inventory.
It would simply be impossible to use spreadsheets to model the supply chain—with all its complexities—accurately enough to proactively identify bottlenecks in storage space or goods handling capacity. Even building a simplified model would be enormously time-consuming and prone to error. Using supply chain projections is the only way to detect the types of moving capacity bottlenecks described above with any degree of certainty. To learn more about effective S&OP in DIY retail, watch Leroy Merlin Italy’s success story.
Once you’ve identified potential bottlenecks, DIY retailers should use “what-if” scenario planning to examine and eliminate them. Bottom-up scenario planning allows you to see exactly how changes in inbound deliveries, store allocations, and forecasted sales volumes will impact the flow of goods, storage requirements, and resources needed for goods handling.
Use your software’s scenario planning capability to identify the scenario that best meets your goals and come to an agreement with your suppliers on it. This enables you to lock your plan down well in advance of the season so your planners can focus on execution and corrective actions.
6.3 Efficient supplier collaboration
Supplier collaboration has been discussed for decades, but surprisingly, few retailers have implemented it extensively. To establish fruitful collaboration, both parties need to a) put in the effort and b) receive measurable benefits from the process. Unfortunately, because this has rarely been the case, many collaboration initiatives fail.
While technology doesn’t solve the challenge of supplier collaboration, it can ease the pain. For example, most collaboration projects spend most of their effort collecting data from various sources, but the right planning system can minimize that work. Rather than trying to fix everything in one go, we recommend building your supplier collaboration processes bit by bit.
A good starting point is sharing order forecasts with your suppliers because it’s a lean way to collaborate. Suppose your planning system can calculate supply chain projections. In that case, the purchase order forecast, which tells your supplier what you plan to buy from them in the coming weeks and months, is already readily available. A good system can send automated reports that share that information with your suppliers.
You can also share relevant information on planned promotions, upcoming events, or other changes, helping your suppliers understand the reasoning behind your purchase order forecast. DIY retailers may also share demand forecasts or point-of-sale (POS) data with their suppliers, but the most essential information to share is what you expect the supplier to deliver and when.
A more collaborative way of working requires both parties to recognize the value that investing their time and effort will bring. Whereas simply sharing a forecast is one-way communication, Collaborative Planning, Forecasting, and Replenishment (CPFR) is true two-way communication. A good planning system helps by providing reliable projections of future purchase orders, analytical tools to understand potential changes and issues, as well as a platform or portal for the collaboration itself.
Ideally, retailers can give their suppliers access to their projected demand, plans for purchase order placements, and data on promotions, seasons, events, etc., to which suppliers can then add their own views. Combining a supplier’s holistic view of their categories and products with a retailer’s understanding of your business and marketing activities like this ultimately results in a more accurate overall plan.
Best-in-class planning systems can support this kind of collaboration by providing a platform that can take in multiple forecast types, alert users to any differences, allow users to edit plans, and finally, disaggregate the agreed plan to whatever level of detail is needed—whether stores, products, or days—to support operational execution.
7. Team up with the machines to win
Retail is in turmoil, and it is unclear what the impact of different sales and delivery channels, store formats, or even retail players will be. In 10–15 years, we will probably look back at this time in amazement and wonder, “How did we not see this coming?”
Some predictions about the future of DIY retail are still easy to make, though:
- Supply chains will grow more complex. As DIY retailers implement new channels to meet consumer expectations—for example, rapid delivery of even the largest products, direct-to-site deliveries for professional end-customers, or BOPIS options for individual shoppers—their supply chains will only increase in complexity. Retailers must master their retail data to understand where inventory should be stocked and what capacity and resources are needed for fulfillment.
- The DIY retail supply chain will become more effective. Having grown quite price-conscious, consumers no longer accept premium prices to keep inefficient supply chains in business. No one benefits from wildly fluctuating workloads or capacity requirements caused by poor planning and management, so neither retail employees nor management should be sad to see old, inefficient practices go.
- Technology and automation will play a big part in retail’s transformation. We have already seen this in other sectors that once relied heavily on manual routine work. There’s no reason why retail wouldn’t follow suit.
Home improvement supply chains need to become more responsive and finely controlled than ever to meet end-customer demand. At the same time, retail supply chains need to become more efficient by optimizing inventory flows from multiple perspectives—store operations, distribution, picking, and warehousing—to meet the price pressure. This is only possible by teaming up with intelligent machines.
The world of DIY retail is too complex to be managed with notepads and intuition—this has been true for a long time. The breaking news is that not only are the simplest jobs being automated, but significantly more advanced planner roles are being filled by machines, too. More importantly, intelligent automation will replace manual work and take planning to a level of granularity never seen.
Will there then be any role for humans in this brave new world? Yes! There will be plenty! Three essential roles are:
Masters of the machines: We are making significant progress in specialized AI, the kind of machine intelligence useful for solving very specific tasks. However, we still need talented people to design the systems and determine when and how the available machine intelligence is best used.
Colleagues to the machines: Machine learning algorithms are very dependent on access to data. They have difficulty applying common sense or developing innovative solutions in new scenarios with insufficient data. This is where their human colleagues can provide invaluable insight.
Innovators think beyond the machines: Especially in businesses experiencing creative destruction, there’s a great need for novel thinking, new business models, and new ways of delivering goods to consumers. Retail innovation is still far beyond AI’s capabilities.
So please don’t hold your breath waiting for AI to revitalize your retail business or even solve your DIY supply chain challenges. But please do start phasing in machine intelligence where it is most feasible and impactful. This collection of best practices is a good place to start.