Machine learning in CPG demand forecasting: Considering retailer data drives results
Sep 9, 2021 • 4 min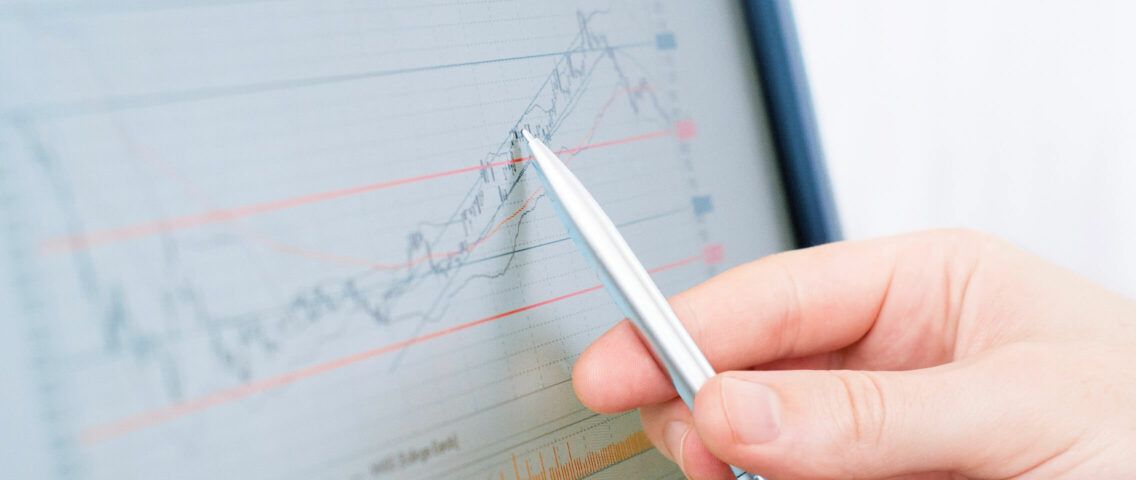
For most consumer packaged goods (CPG) manufacturers, the supply chain—from sourcing through production and finally distribution—is quite long. When sourcing raw materials and packaging, for example, your lead times may range anywhere from a few weeks to several months. CPG companies targeting high-yield production efficiency and low time investment on set-ups need production batches that can cover several days or weeks of demand. And before any of those end-products can reach the consumer, they need to pass through regional distribution centers and retail stores, tacking on additional days or weeks.
To effectively manage a value chain this long and complex, your company must excel at demand planning. CPG manufacturers can’t afford the luxury of responding to consumer demand; they must anticipate it. Poor demand forecasting poses two significant risks:
- Losing sales and customers due to poor product availability
- Eroding your cost-effectiveness through obsolete stock or inefficient production
So, how can CPG manufacturers improve their demand forecasting?
Retailer Data Is Critical to CPG Demand Forecasting
CPG companies actively shape demand for their products through pricing decisions, brand promotions, product ranges refreshes, and the introduction of product innovations. Because they hold these pieces of the demand puzzle in their own hands, they can usually account for them in their demand forecasts.
However, some important pieces of the demand puzzle—namely retailers’ business decisions—are outside of their control, so CPG manufacturers often can’t account for them in their forecasts. Even with direct-to-consumer channels, the majority of CPG sales still come from retail orders. Forecasts that don’t incorporate any information from retail customers simply cannot be accurate enough for effective planning.
Retailers’ commercial decisions on store assortments, product displays, promotions, and in-store events impact their own demand, which then impacts your demand as a CPG manufacturer trying to fulfill their purchase orders. Retailers’ supply chain strategies—for example, how early to stock up before a product launch, promotion start, or major holiday—also directly impact the retail orders you fulfill.
At the end of the day, CPG manufacturers must be able to leverage retailer data in their own demand planning and collaborate effectively with their retail partners to attain high forecast accuracy and meet demand.
The good news is that many retailers already make point-of-sale (POS) data available to their CPG suppliers, and they’re increasingly sharing inventory data and order forecasts as well. The bad news is that this data is still grossly under-utilized by CPG manufacturers. But why?
In our experience, the primary inhibitors are:
- Relying on aggregate forecasts of multiple banners’ demand per distribution center or region, which makes it difficult to understand the impact of a single banner’s decisions on total demand
- Demand planning systems that aren’t designed to take in, clean, and leverage external data that comes in many different shapes and forms, impeding automated forecast calculations based on the latest retail data
Fortunately, best-in-class demand planning tools are designed specifically to support the use of external data in more granular, banner-level demand forecasting.
Machine Learning and Retail Data Improve CPG Manufacturers’ Forecast Accuracy
Machine learning enables CPG companies to achieve more granular forecasting with less effort. Many CPG companies rely on aggregate forecasting only because their manual forecasting processes can’t scale well enough to forecast at a banner or store level. Machine learning-based forecasting solutions, on the other hand, introduce high levels of automation that enable far more granular forecasting without any additional planning effort, in fact reducing hours of manual review on a daily basis through exception alerts.
A demand planning system that uses machine learning can automatically consider enormous amounts—more than any team of human planners could ever manage—of the retailer data that ultimately impacts your demand calculations. The more banner-level data that you, as a CPG manufacturer, can bring into your demand planning system—for example, the number of stores selling the product both now and after the next assortment review—the more quickly machine learning technology proves its value. And because machine learning algorithms are continuously self-learning, forecasts only improve over time as the system takes in more and more data. The result is a more accurate, granular forecast.
Machine learning-based forecasting consistently performs better than time-series based forecasting, especially in complex situations like promotions or moving holidays such as Christmas. However, we’ve found that further enriching that machine learning-based forecast with additional retail data (such as the number of banners or stores carrying a specific product) enables CPG manufacturers to react automatically to retailers’ assortments changes.
In our experience, using retailer data increases overall forecast accuracy across all products in your range by several percentage points, with notably bigger forecast accuracy improvements for products impacted by retailers’ assortment changes or promotions.
Retail Data Drives Immediate Results and Long-Term Opportunities through Collaboration
The ability to process banner-level data from retail customers has an enormous impact on forecast accuracy. With a more accurate demand forecast, your business can drive a range of performance improvements throughout the CPG supply chain, such as:
- Improved service levels
- The ability to execute on-time, in-full deliveries to your retail customers
- Reduced inventory holding needs and costs
- Lower spoilage levels
And this, of course, is only the start. As CPG manufacturers and retailers begin to see the tangible results of a collaborative relationship, it will only whet the appetite for more—comparing forecasts and collaboratively resolving discrepancies to further enhance all parties’ demand planning accuracy, operational efficiency, and service levels. Once a basic foundation of granular, machine learning-based demand forecasting is in place, your CPG company will be ready to take the next step: true two-way collaboration with your retail partners.