The function of gen AI and the future of work in supply chain
Aug 2, 2024 • 5 min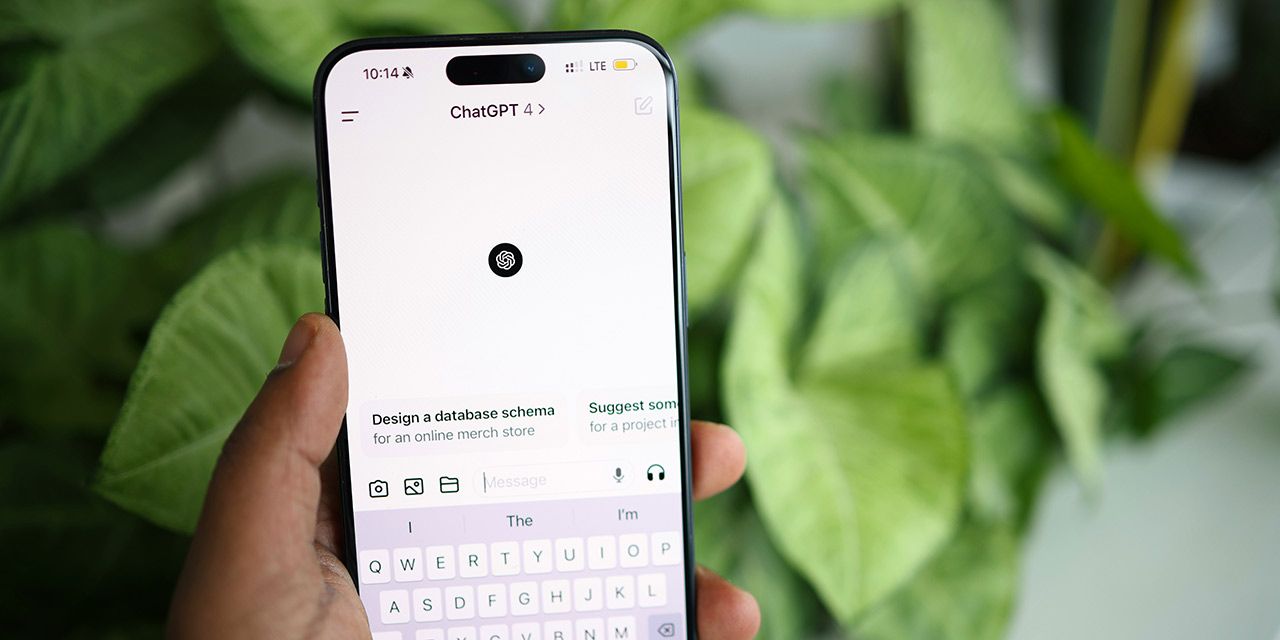
As systems like ChatGPT rattle established tech stacks and business processes, some are hailing “generative AI” as the magic pill that will cure all work-related ills. Others see it as a precedent to an insidious puppet master that will render humanity redundant.
Neither scenario is accurate.
Both viewpoints assume that gen AI is something that it’s not – an all-knowing, all-powerful technology capable of accomplishing any task at superhuman speed.
But that just isn’t the case. Simply put, gen AI is one tool among many. There are tasks at which it excels and tasks at which it fails miserably.
This begs a few questions:
- What exactly is gen AI? What can it do (and not do)?
- What are the best use cases for gen AI in supply chain planning?
- How will integrating gen AI shape the future of work in the supply chain world?
With a balanced approach and a clear understanding of gen AI’s strengths and limitations, there are some exciting possibilities to explore.
What is gen AI and how does it work?
Gen AI (derived from “generative AI”) usually serves more general-purpose use cases. In most business settings, the phrase refers to a subset within gen AI of large language models (LLMs) popularized through the likes of ChatGPT.
The foundational LLMs (a.k.a foundation models) such as OpenAI’s GPT, Anthropic’s Claude, or Meta’s Llama are trained on enormous amounts of publicly available data. Essentially, they function as large prediction machines, answering the question: “What’s the best response to your query based on having read the whole internet?” As anyone who has used them knows, LLMs do have a tendency to “hallucinate,” and they do not perform complex mathematical calculations too well.
They excel, however, at reasoning, processing, synthesizing, and delivering information in a clear, conversational way. So how can we apply these strengths to supply chain management to make human lives better?
Use case #1: Vertical copilots
Eventually, AI copilots will become ubiquitous. Sometimes referred to as “assistants,” copilots are AI-powered systems, often built on foundation models, enriched with proprietary data, and optimized to serve a specific business domain.
Think of how we’re already using our phones to organize our daily lives. We’re moving toward a future in which a copilot on your phone could, for example, send you an alert: “Your flight leaves at 10am, but traffic is getting worse. Would you like to call you an Uber now?”
To be clear, a highly specialized machine learning model on the backend (not gen AI) is doing complex calculations to recognize that a 15-minute drive just turned into an hour. Machine learning is a subset of AI that analyzes swaths of data to identify patterns and generate predictions, continuously refining its calculations based on outcomes. (Supply chain already leverages machine learning to improve plans and forecasts.) But in the case of your ride to the airport, gen AI would enable easy, conversational task management to ensure you make your flight.
In the business world, some companies are already using vertical copilots, which are assistants trained to support a particular industry (e.g., coders who use GitHub Copilot to speed up more mundane tasks or identify coding issues).
So what might a vertical copilot look like for the supply chain industry?
First, let’s tackle the foundation of a good copilot – its knowledge base. A supply chain copilot should be equipped with industry-wide best practices, allowing it to answer more general questions, such as: “What factors should I consider for preseason planning?” It should also make information about its complementary planning solution easily accessible so users can onboard quickly, clarify UX questions, and deepen their general understanding of their planning tool. This knowledge base must be continually updated with the latest information and best practice developments.
Underpinned by this wealth of knowledge, the copilot should seamlessly integrate across solution suites and daily workflows. It might be trained to support supply chain in general or instead support specific job functions like demand planning or supply planning.
Beyond best practice recommendations, the copilot will be able to generate context-aware answers specific to a company’s supply chain, providing more advantages to users, such as:
- Answering network-specific questions, such as: “Where am I most at risk of not meeting expected customer demand, and how can I address the problem?”
- Proactively posing what-if questions to backend machine learning, alerting users to anomalies, and recommending actions.
- Organizing business functions, such as S&OP, by synthesizing documents and conversations to summarize meeting notes, assign action items, and determine deadlines.
Human-to-human collaboration will remain a hallmark of supply chain planning; after all, supply chain is a people business. However, gen AI provides the gateway to both human-to-machine (which is currently the dominant use of gen AI) and machine-to-machine collaboration. This will alleviate grunt work and elevate productivity for more efficient supply chains and business processes.
Use case #2: Conversational commerce
Conversational commerce is the concept of using gen AI to power chatbots that interact with consumers and make shopping recommendations based on consumer behaviors.
This is merely the next development in social commerce. Retailers are no strangers to omnichannel ecosystems, and even manufacturers are casting their eyes on direct-to-consumer business models. These days, consumers expect items to be available through multiple buying channels, and fierce competition necessitates smart channel-level planning.
That’s why, as conversational commerce develops, it’s important these bots incorporate near real-time data and integrate with the rest of the supply chain planning process.
How can supply chain benefit from such an agent? Suppose someone likes or comments on a social media post about a particular pair of shoes. A chatbot would reach out to that person, showing them the shoe in all its available colors and sizes. Supply chain visibility into current inventory levels or future projected inventories would ensure the bot never recommends the item in colors or sizes that are unavailable or anticipated to be out of stock.
In this way, companies can provide a more personalized shopping experience while also protecting retail inventory and preventing stockouts as new channels open up.
The future of work: The human element
Gen AI is a great way to train and equip users, and it has its place among the many technologies companies use to better manage their supply chains. For instance, machine learning is very much the most relevant technology for forecasting, but gen AI can easily communicate demand information and insights to users for better decision-making.
Gen AI is now another tool in the AI toolkit for business decision-making. It offers significant potential by complementing existing algorithmic approaches such as optimization, machine learning, and heuristics, rather than replacing them. By leveraging AI, human planners and decision-makers acquire superpowers that equip them with great insights and recommendations so they can put the best of human ingenuity to work. After all, as the saying goes, “the role of AI is not to turn a human into robot, but to take the robot out of the human.”
At the end of the day, gen AI applications are about empowering humans and enriching their daily lives. The right tools provide the best insights but will always need someone guiding the strategy.