Demand sensing: How to conquer CPG supply chain chaos
Jul 31, 2024 • 9 min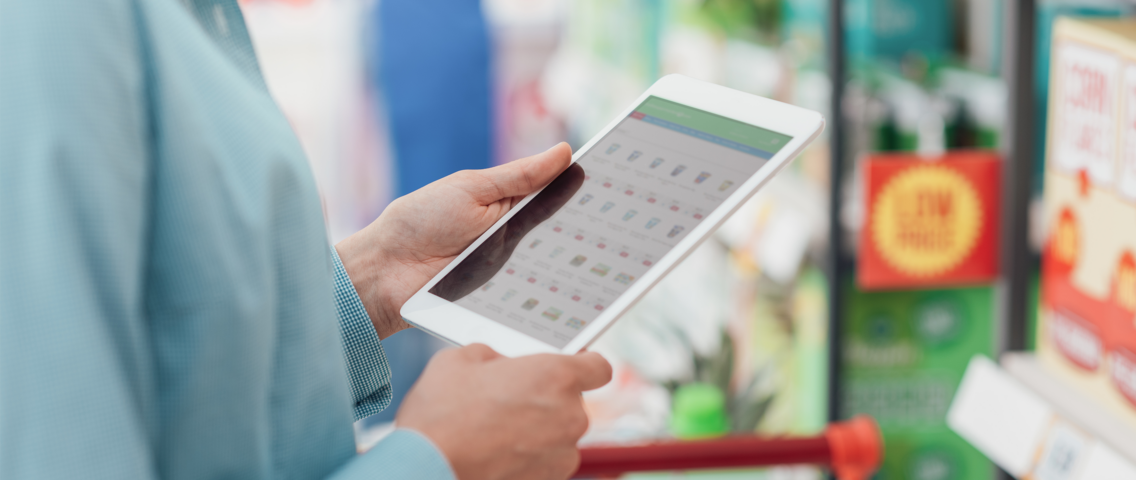
Consumer packaged goods (CPG) companies remain under pressure to maintain some semblance of control over their supply chain, even after years of intense disruption. The manual forecasting processes many companies use to stay centered through the chaos have been pushed past their limits. Consumer demand is constantly shifting based on the ever-changing, uncertain economic landscapes. Companies that primarily rely on historical order data are finding out they struggle to accurately forecast even the immediate future.
To get out from under the immense strain of these disruptions, planning teams have increasingly turned to demand sensing. The high accuracy and cost reduction achieved through demand sensing come at a time when consumer goods companies sorely need both. And the benefits extend beyond uncertain economic climates.
Seeking relief from economic uncertainty, CPG companies and their close cousins, fast-moving consumer goods (FMCG), should improve their supply chain processes with a demand sensing solution that pays off quickly and saves businesses time and money well into the future.
What is demand sensing?
Demand sensing refers to integrating internal data with retailer point-of-sale (POS) data and other external data sources to create and adjust short-term forecasts. Including external data and using machine learning software helps companies “sense” what’s happening regarding consumer demand and make changes accordingly. Forecasts built using demand sensing solutions achieve better accuracy and adjustment times than ones created using traditional forecasting processes.
Demand sensing vs demand forecasting
Unlike traditional forecasting methods relying on historical sales data, demand sensing and machine learning-based demand forecasting can process real-time data and demand signals. The difference lies in how they use these inputs.
Demand sensing is built for speed. Its hyperfocus on immediately integrating data for rapid responses to market changes makes it a heavy hitter in short-term demand planning, a boon to FMCG companies.
While machine learning-based demand forecasting can also quickly incorporate and analyze new demand data, it focuses more on identifying trends and patterns, delivering better insights, and providing a rock-solid foundation for long-term planning.
Why is demand sensing important?
The argument for using a demand sensing and machine learning-driven forecasting solution over traditional (often more manual) forecasting comes down primarily to data—namely, the ability to quickly and accurately process vast amounts of it from numerous sources, including external and POS retail data.
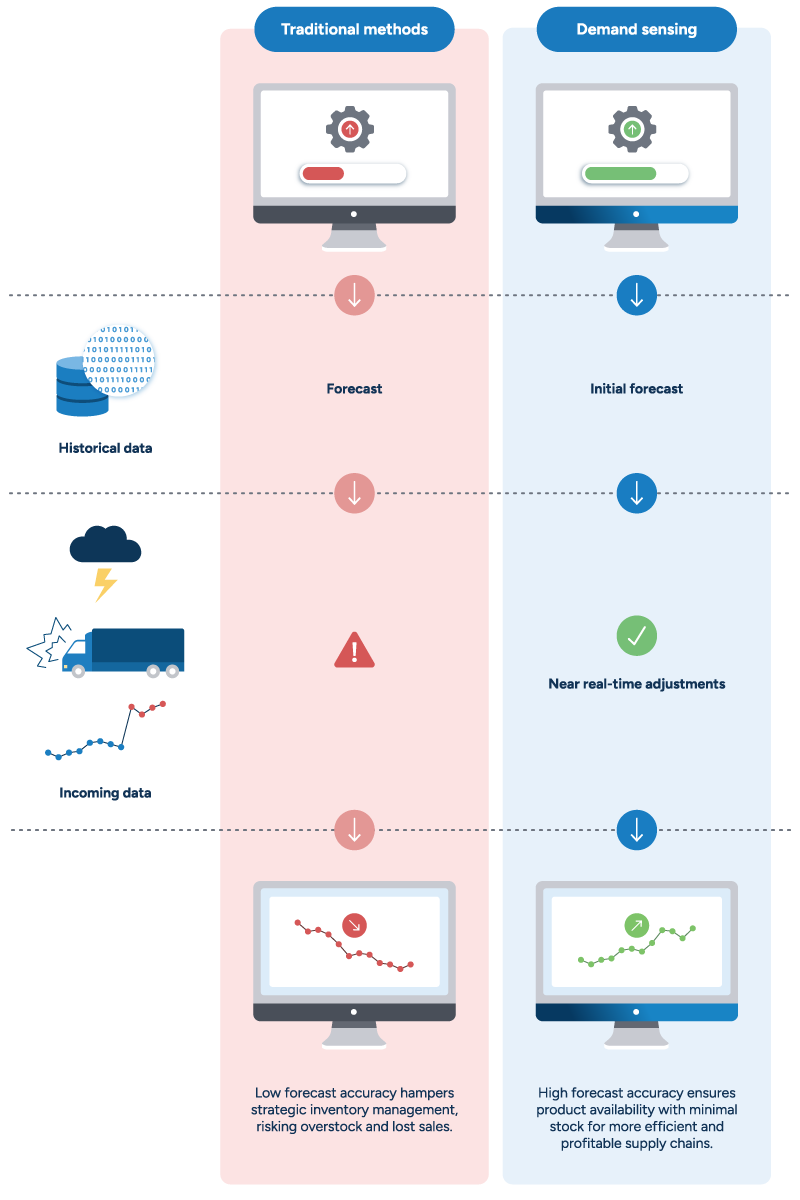
Demand sensing embraces daily forecasting to improve accuracy and reduce cost and waste in supply chain planning. Current, relevant data from retailers and other external sources informs these forecasts, which update automatically in response to new information.
For instance, a forecast based on distribution center (DC) reorders and strengthened with granular POS retail data will reflect current demand better than one based solely on DC reorders. Reorders reflect what was happening recently, whereas POS data is closer to how consumers are acting now.
Constant data updates and automated adjustments prevent a strict adherence to dated forecasts, and the machine learning function promotes continuous performance improvements. Demand sensing software also considers factors like current trends, retailer decisions, market conditions, and even external events like inclement weather to improve forecast accuracy.
This way, demand sensing software reduces inventories, optimizes processes to produce long-term cost savings, and decreases lost sales through increased availability.
Read more: Demand forecasting for retail and consumer goods: The complete guide
Challenges limiting forecasting potential
Supply chain decision-makers and planners have traditionally developed forecasts based on internal and historical order data. The logic is sound to a degree. However, a reliance on the manual processing of internal and historical order data has a few significant shortcomings.
Traditional methods cannot:
- Account for unprecedented external disruptions that fundamentally alter consumer demand year-over-year. (For example, demand in April 2020 was certainly different than that of April 2019.)
- Detect shifts in consumer demand that occur within days — shifts caused by unforeseen events like social media trends or unexpected retailer promotions and pricing changes.
- Anticipate retailer orders that occur after initial pipeline fills for planned events like product introductions and terminations, seasonal changes, and assortment changes.
Consumer goods companies should avoid these pitfalls even in sunnier economic situations, but these issues are especially costly in the current market. Manual forecasts require the wrangling of massive amounts of data on the part of planners. And demand can change dramatically in a matter of days, creating a situation where planners constantly react to changes but never quite catch up to them. These inaccuracies in forecasting ultimately lead to waste, especially for businesses dealing with fresh products or other goods with extremely short shelf lives.
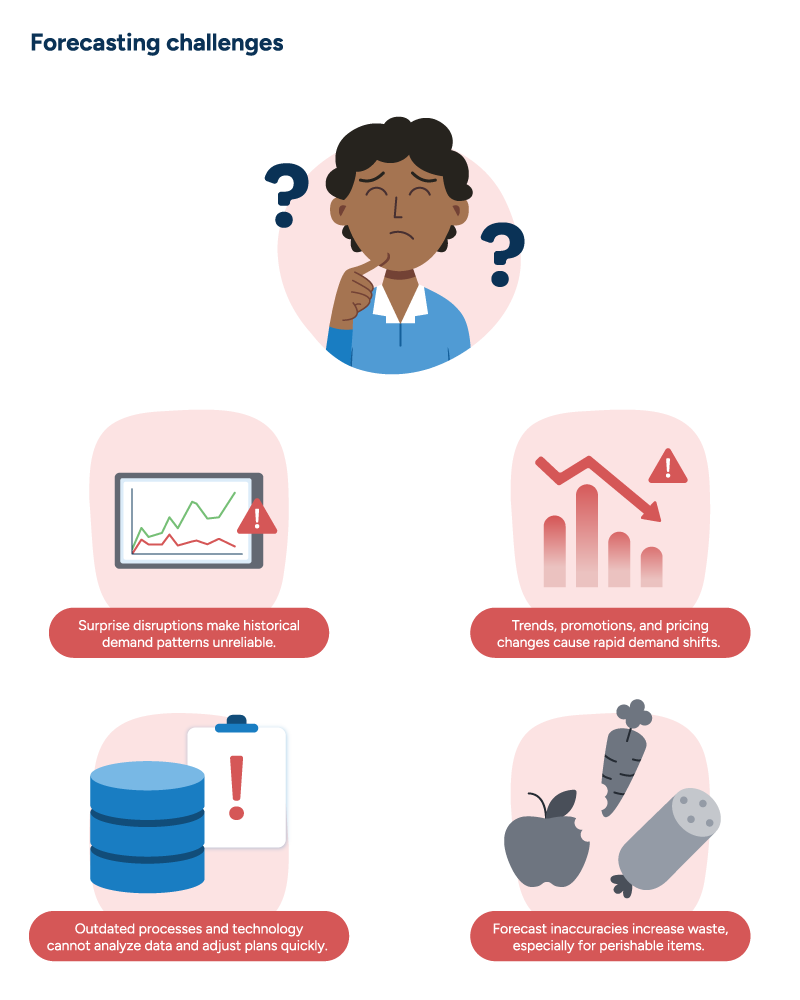
3 ways demand sensing capabilities reduce costs with better forecasts and responses
AI- and machine learning-powered demand sensing software may seem like a wish list item to businesses beholden to their spreadsheet-based supply chain systems. Yet, an increasing number of companies already use such tools to optimize their supply chain planning processes.
Companies still weighing the adoption of demand sensing software should consider the three major ways demand sensing positively impacts revenue.
1. Detects immediate changes in demand and consumer behavior
Demand sensing solutions process streams of current external data instead of relying solely on historical trends. This allows consumer goods companies to see demand changes as they happen versus making assumptions based on previous years.
Demand sensing in supply chain combines three sources of data to build and adjust forecasts:
- Internal company data. This category includes price changes, assortment plans, product introductions and terminations, promotions offered to retail and wholesale customers, and any other data generated from within the organization.
- Retailer data. These sources include POS data, pricing incentives, retailer promotions, assortment decisions, marketing campaigns, current and projected store and DC network inventory levels, open sales orders, and any other retailer data.
- External data. Comprehensive demand sensing solutions also factor in competitor actions, weather, sporting or entertainment events, and other complex or random events that are likely to impact consumer demand.
Read more: Machine learning in CPG demand forecasting: Considering retail data drives results
2. Automates forecasting adjustments
Robust demand sensing solutions minimize the amount of manual work required to create and adjust forecasts. AI- and machine learning-capable programs can detect trends and demand changes faster than any team of humans could, significantly reducing the time between trend detection and forecast adjustment. This lightning-fast reaction time helps limit costly over- and understocking,
Machine learning also trumps manual forecasting in another big way: the ability to capture and build from insights. It takes workers time and effort to perform a manual analysis – work that must be repeated each time a forecast is created. A machine learning solution instead automatically retains the information gleaned from these analyses and learns from each event, preventing planners from continuously repeating the work.
Automation allows flesh-and-blood planners at consumer goods companies to focus on high-value tasks outside of the solution’s capabilities—namely, exception evaluation. A demand sensing solution identifies forecasting outliers and brings them to the attention of the planner, who, in turn, determines whether the forecast is valid or not. The machine uses external data sources to contextualize these exceptions and help the planner make the correct judgement regarding forecasting accuracy.
3. Provides a comprehensive picture of demand needs
Reliance on any one source of data hinders forecast accuracy and handcuffs businesses to rigid forecasts. A forecast based exclusively on POS and consumer demand drivers like promotions could lead to an inventory that doesn’t align with the actual order needs of the retail customer.
This issue presents itself particularly with new items delivered in relatively large packs. Creating a forecast based only on POS data may lead to the supplier building up a large amount of stock too quickly. Companies then house stock that won’t be needed until several weeks or even months later, creating obsolescence issues for items with shorter lifecycles or limited shelf life.
A business can instead combine retailer POS data with historical order data to quickly adjust inventory, distribution, production, purchasing, and sales plans with an up-to-date forecast. Companies must also consider inventory buffers, lead times, delivery schedules, and order batch sizes in each stage of the supply chain to accurately determine future supply chain needs.
This consolidation of up-to-date data from multiple sources also allows businesses to plan distribution and inventories better, especially when the flow of goods is unstable. Consider a company that creates a baseline forecast before running a promotional campaign. Even slight inaccuracies in the forecast can result in costly stockouts. However, demand sensing software updates daily and tracks the realization of the forecast in real-time, allowing companies to take immediate action if units are moving quicker or slower than anticipated.
Demand sensing sheds light on other situations involving an unstable flow of goods as well, including:
- New product launches
- Product ramp-downs
- Marketing campaigns and promotions
- Seasonal goods
- Holiday-related fluctuations
Read more: Supply chain optimization for manufacturers
Benefits of demand sensing
As the intricacy of the global supply chain increases alongside consumer demand, investment in a new or improved demand sensing solution will help companies better withstand current and future supply chain disruptions and frustrations.
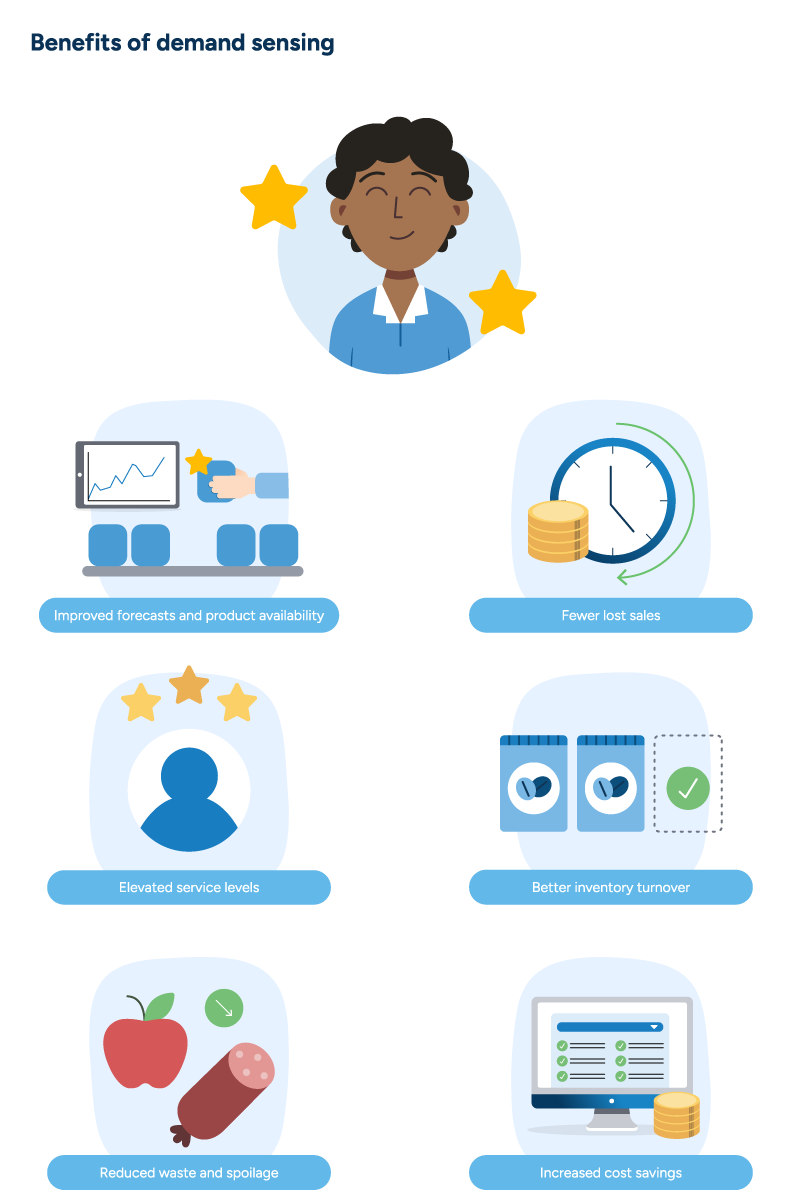
A robust demand-sensing solution helps businesses gain certainty in an uncertain market by detecting immediate changes in demand and consumer behavior. Powerful software equipped with machine learning capabilities parses through this massive volume of data to determine how demand is shifting daily. Three significant cost-saving benefits of immediate demand change detection include:
- Fewer lost sales through improved availability.
- More accurate product supply compared to actual demand during step-changes in promotions.
- Reduced waste and spoilage, especially for fresh products with short shelf lives.
Demand sensing in action
For over a decade, RELEX has helped consumer goods companies improve their forecasting and decision alignment.
Consider Atria, a RELEX customer and one of the leading food suppliers in Northern Europe with €1.5 billion in net sales as of 2021. The company needed to improve its forecast accuracy for highly seasonal goods and meat products with exceptionally short shelf lives. Atria used RELEX’s demand sensing capabilities to achieve an incredible 98.1 % weekly forecast accuracy while reducing manual forecasting changes by 13%.
“We continue to reap the benefits of that relationship while expanding our use of their solution’s capabilities,” said Pekka Korpeinen, Director, Steering & Planning for Atria. “It’s apparent to me that RELEX and Atria share the same level of ambition when it comes to exploring how consumer packaged goods companies can derive maximum value from modern planning technologies. The addition of RELEX’s machine learning-based forecasting has greatly contributed to the continued success of our business.”
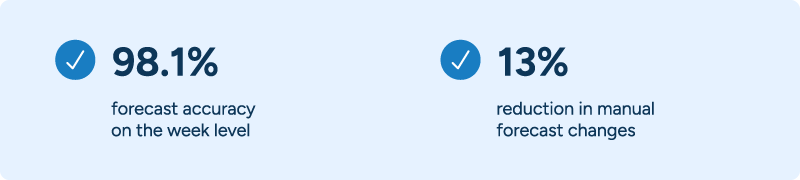
Outpace a rapidly changing market
From tackling disruptions to being inundated with data, CPG and FMCG companies face serious challenges. Demand sensing is how they even — and overcome — the odds. By leveling up their planning solutions and equipping their teams with the AI-driven insights and capabilities they need to thwart uncertainty, companies achieve efficiency gains that translate into better service levels and long-lasting profitability.
At RELEX, our long history of working with retailers worldwide means that no one in the world knows better what and how retailers buy from consumer goods companies. The RELEX solution can be custom fit to solve your unique pain points and optimize even the most complex supply chains, equipping your team with the tools and insights needed to deliver measurable business value – now and in the future.
Don’t get caught clinging to outdated manual systems during the next big disruption.