Artificial intelligence (AI) is attracting a lot of attention in retail. The reason is obvious: today’s retailers can collect massive amounts of data on transactions and interactions with consumers both online and in brick-and-mortar stores. At the same time, they have to make billions of decisions to align their inventories, capacity, and staffing with ever-changing consumer demand. In short, retail is the perfect match for AI.
The hype around artificial intelligence in retail has led to something of a gold rush. Application vendors are quick to slap an “AI inside” sticker on their products, portraying their solutions as more intelligent than they really are. In such a business environment, it’s no wonder many retailers are confused. Is AI all hype, or are the retailers who have yet to implement it laggards in danger of going out of business?
This paper won’t provide any vision statements or roadmaps about the future of AI. Our goal here is to provide an accurate overview of how retailers are applying artificial intelligence to optimize their businesses today. First, let’s look at what AI is and what it isn’t.
1. Artificial Intelligence Is Algorithms, Not a Person
Technology companies have been eager to position their AI algorithms as “intelligent” by making them seem as human as possible, even giving them human names such as Siri, Alexa, Einstein, or Watson. It’s essential to keep in mind, though, that AI is not a person. AI is not even a singular “it.”
We are still far from general artificial intelligence that would be able to creatively solve ill-defined problems. We are, however, making great progress in specialized AI that solves well-defined problems (such as algorithms for image recognition) and combinations of several types of specialized AI (such as self-driving cars).
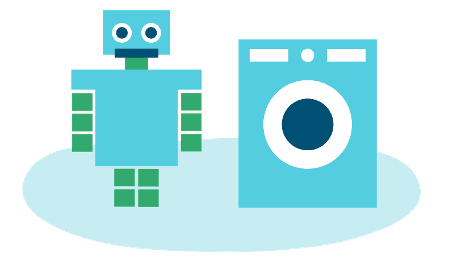
In short, what we call artificial intelligence today is a collection of algorithms, nothing more and nothing less. Some of these algorithms consist of simple components ingeniously combined to produce reasoning so complex that data scientists struggle to explain it (think deep learning). Others are so simple that anyone two weeks into a computer science class could code them into a computer program that beats you every time in zero-sum games like mancala (think minimax algorithms).
Different AI algorithms are not better or worse. Instead, they simply use different types of data and are applied to solve different kinds of problems.
This means retailers do not need “AI”—they need to employ several AI algorithms in their analytics toolbox to supplement “old hat” technologies like statistical analysis and rules-based heuristics. Furthermore, retailers must still leverage human expertise to define business priorities and resolve ill-defined or unprecedented problems.
So, if we look behind the hype, how does the pragmatic application of AI help retailers optimize their business?
2. AI Delivers More Automated, Granular, Accurate Retail Decisions
AI makes it possible for retailers to produce faster, more accurate decisions—an invaluable asset in an industry with millions of goods flows to control. Machine learning algorithms, for example, consider hundreds of potentially demand-influencing factors when forecasting retail sales, something a human demand planner could never achieve. The application of AI in retail is not limited to demand forecasting, though. Retailers can reap even greater benefits by leveraging AI to optimize the full range of their operations.
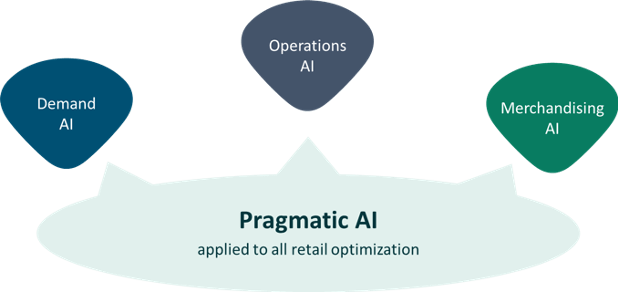
2.1 Artificial Intelligence for More Accurate Retail Demand Forecasts
What started with a few forerunners (like RELEX) has become mainstream over the years: today, many retailers apply machine learning in demand forecasting to predict the impact of factors like weather, for example, on sales. Currently, retail technology vendors either apply machine learning or are rushing to update their legacy systems to offer machine learning for retail demand forecasting.
Machine learning gives a forecasting system the ability to learn automatically and improve its predictions using data alone, with no additional programming needed. Because retailers generate enormous amounts of data, machine learning technology quickly proves its value. When a machine learning system is fed data—the more, the better—it searches for patterns. Going forward, it can use the patterns it identifies within the data to make better decisions.
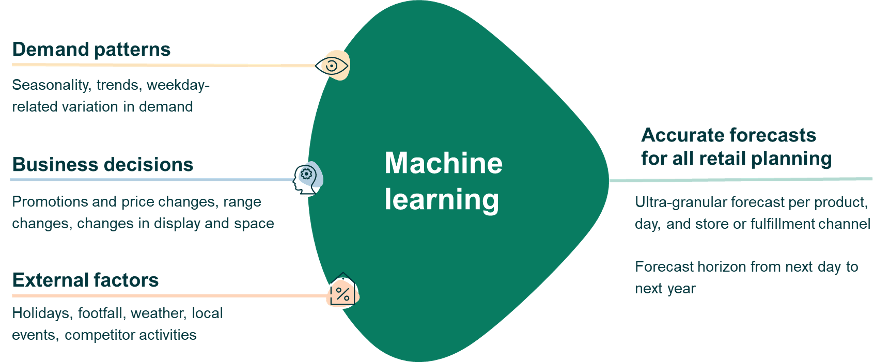
Machine learning makes it possible to incorporate a wide range of demand-influencing factors and relationships into your retail sales forecasts. This is enormously valuable, as weather data alone can consist of hundreds of different factors that can potentially impact demand.
When machine learning is implemented in the right way, effectively addressing common challenges with retail data, it delivers benefits across all facets of retail demand forecasting:
- Capturing recurring demand patterns caused by weekdays and seasons
- Forecasting the impact of promotions, price changes, and other internal business decisions
- Predicting the impact of local footfall, local events, weather, and other external factors
In simple situations, such as dealing with recurring variations in demand, machine learning delivers equivalent or slightly better forecast accuracy than traditional demand forecasting methods. When dealing with complex situations, though, such as overlapping promotions or sales cannibalization, machine learning-based forecasting clearly outperforms traditional forecasting approaches.
2.2 Artificial Intelligence for More Cost-Effective Retail Operations
Despite its importance, improved demand forecasting does not deliver much value unless the forecasts are put to good use. Forecast-based optimization of retail operations is an area where a pragmatic approach to AI provides incredible results. Often, the return on investment for forecast-based workforce and supply chain optimization is even higher than that for improved retail demand forecasting. For a large retailer, the attainable annual cost savings amount to tens of millions of dollars.
2.2.1 AI in Workforce Optimization
Personnel is one of the biggest assets in retail, but also one of the biggest costs, amounting to some 10–20% of revenue. Consequently, more accurate workforce planning can generate millions in annual savings through reduced over-staffing. At the same time, more accurate workforce planning drives employee satisfaction and revenue by ensuring that store staff are not overwhelmed by the amount of work assigned to them and are available to provide a high level of service to shoppers.
Automated workforce management, or workforce optimization, is a multi-faceted problem too complex to solve without the use of AI. Many moving parts need to be simultaneously considered to reach optimal outcomes. First, retailers must use several forecasts to predict future workload accurately:
- Predicting customer service workload, such as staffing a cash register, requires customer footfall forecasts
- Estimating in-store replenishment workload, such as shelving, demands visibility into inbound delivery volumes
- Preparing for fulfillment work, such as order picking, requires online order and order line forecasts
This total workload must then be optimized so that time-critical work like customer service can be performed when there is demand for it, and less time-critical tasks are scheduled around that to enable reasonable work shifts.
Finally, retailers must be able to optimize work shifts to match workload, contractual restrictions (such as minimum and maximum hours), and regulatory restrictions like mandated breaks and time between shifts. The optimization must also consider that not all employees will necessarily have sufficient training to perform all tasks, and recommend the best-qualified resources.
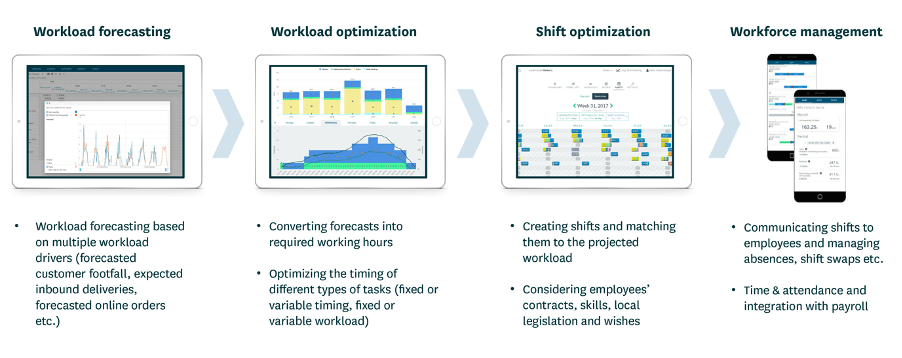
By applying AI pragmatically through a combination of algorithms, this complex shift optimization problem can be solved much more accurately than before—and in mere seconds. AI makes it possible to satisfy both the short-term need to create well-balanced, effective work shifts and the long-term need to ensure the business meets contractual and regulatory requirements.
The financial impact of retail workforce management and optimization is enormous: up to a 10% decrease in personnel costs, which translates to more than $10 million in annual savings for a retailer with $1 billion in revenue. At the same time, employees benefit from more level workloads and better visibility into future work shifts. Workforce optimization also lets store managers spend far less time executing manual planning on their computers and phones, and a lot more time proactively managing their staff. (Read more in this case study.)
2.2.2. AI in Supply Chain Optimization
Mathematical approaches and operations research have been applied in supply chain optimization for quite some time now, but there are novel opportunities in this space as well.
Stochastic supply chain planning, for example, sounds quite fancy, but ultimately just deals with uncertainty in an intelligent way. A tangible example is replacing static safety stocks for fresh products with probability-based order optimization to capture how demand volume and uncertainty fluctuate throughout the course of a week. This enables fresh product retailers to optimize the trade-off between risk of waste and risk of lost sales.
Optimizing fresh food replenishment drives impressive results. We’ve seen retailers reduce spoilage by up to 40%, for example (see this report for more information on food waste reduction and its impact on sustainability.) Since the cost of food waste amounts to more than 1.5% of revenue for the average grocery retailer, waste reduction has the power to drive a significant increase in profitability.
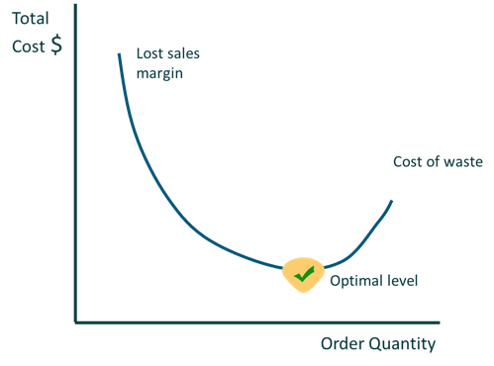
Another valuable use of AI in retail optimization is goods flow smoothing, which ensures more level flow both into distribution centers and from distribution centers to stores. Smoother inventory flow allows for more efficient use of capacity in distribution centers, micro-fulfillment centers, and stores. This is especially valuable for retailers that have invested in automated goods handling; higher capacity utilization drives quicker return on investment in robotics.
The application of AI adds a great deal of value upstream in the supply chain. More importantly, it unlocks completely new opportunities for optimization downstream in the supply chain as well. Optimizing store replenishment to attain a smoother goods flow is inherently complex. The optimization must consider the full range of products carried by each store as well as an array of store- and product-specific data. It must factor in local demand for each product, available shelf space per product, which products are displayed in the same aisle in each store, and product shelf-life. By applying AI—swarm intelligence, in this case—all requirements can be met in a way that increases efficiency both in stores and in the distribution network (read more about the benefits in this case study).
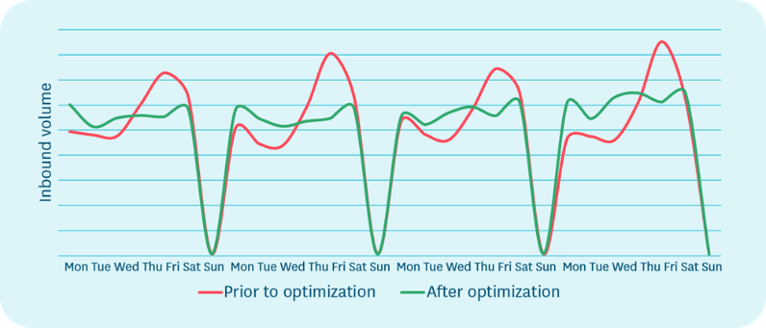
Finally, it’s well known that retail is “another day, another disaster.” When dealing with millions of goods flows, something is bound to go wrong. It is very time-consuming to have human planners manage the consequences of each late delivery, each quality issue, and each unexpected demand fluctuation in the supply chain. Instead, recurring minor disturbances should be dealt with automatically, preferably before they have any notable negative impact on operations.
The good news is that these types of well-defined exceptions lend themselves nicely to autonomous issue resolution. Using pre-defined business priorities and automated rules and workflows, your supply chain planning software can make intelligent decisions on its own—for example, optimally allocating scarce inventory to the right stores or automatically triggering optimized force-outs of stock nearing its expiration date.
2.3 Artificial Intelligence for Better Commercial Decisions in Retail
In merchandising, AI algorithms help retailers gain a comprehensive understanding of consumer shopping patterns, then use that knowledge to cluster products, consumers, and stores. For example, when you shop online, the site often suggests that “you may also be interested in these products.” These suggestions come from a recommendation engine typically based on AI algorithms that analyze other consumers’ shopping carts.
Clustering stores based on local shopping patterns is another application of classification algorithms—the K nearest neighbors algorithm, in this case. Effective store clustering supports retailers’ ability to create assortments tailored to local demand without introducing unnecessary complexity into assortment management.
Optimization algorithms enable more accurate merchandising decisions. Store-specific space optimization, for example, in which each product’s shelf placement and amount of facings is optimized based on local demand forecasts and inbound goods flow. AI-based algorithms like simulated annealing allow space planners to quickly solve complex optimization problems with extremely high accuracy rates. Locally optimized planograms drive tangible business value, reducing goods handling costs and increasing on-shelf availability and sales.
Finally, markdown optimization is a simple but powerful application of AI in merchandising that uses price elasticity models to estimate how price impacts the demand for a product. Price elasticity calculations are, again, not a novel concept. However, today’s planning systems’ ability to perform these calculations on the fly and per product/store, combined with unprecedented inventory visibility and prediction accuracy, has taken a tried-and-true concept to a new level.
Modern, unified retail optimization enables a highly automated markdown process that autonomously identifies the products and stores that will benefit from markdowns, then recommends the optimal markdown prices in accordance with the retailer’s pricing strategy. High levels of automation allow planners to quickly execute markdowns with little effort and accurately unlock new opportunities. Markdown optimization becomes a worthwhile activity in retail verticals where it has not traditionally been used, such as grocery.
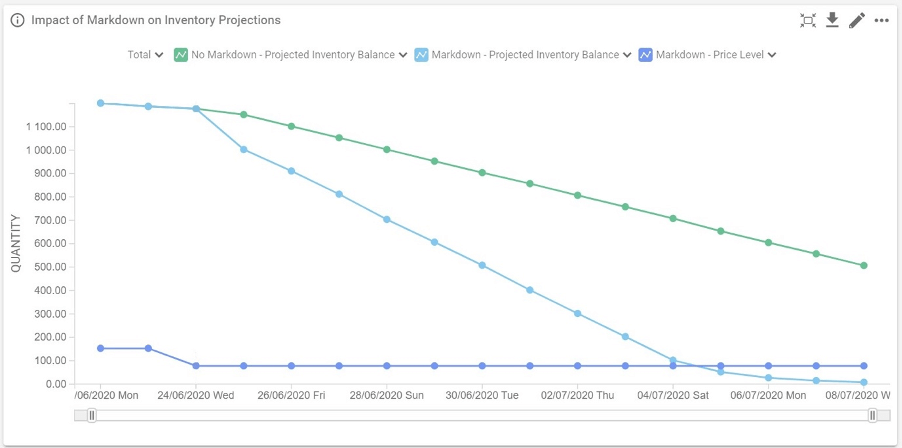
The benefit is clear: our customers have seen 4.9% growth in margin. Markdown optimization also reduces hassle in stores by effectively clearing out stock to make room for new products during assortment changes or season ends (read more in this case study).
3. AI in Retail Optimization Delivers Business Value
When applied to retail optimization, AI delivers astonishing results: improved profit margins, increased sales, reduced waste, and more effective inventory and capacity utilization. The benefits to the retailer, its customers, and even the environment are immense.
The application of AI in your retail operations, however, must be carefully and pragmatically designed. To ensure that you reap the full benefits of AI, your retail optimization software needs to meet these requirements:
- The right algorithms need to be selected based on available data and the type of problem to be solved. The methods must be continuously updated as technology evolves and new data become available.
- The algorithms should operate efficiently and reliably at retail-scale, enabling effective management of retail’s billions of forecasts and tens of millions of goods flows. If data processing takes too long, the recommendations may already be outdated by the time they’re ready.
- All AI approaches must work seamlessly together and support cross-functional planning. The biggest challenge for retailers today is moving from suboptimal functional silos to unified, cross-functional decisions that drive overall profitability.
- The reasoning behind automated decisions and recommendations must be completely transparent, allowing users to fully understand, trust, and sometimes adjust calculations and results without having to be data scientists.
However, while access to more data, better algorithms, and increasing data processing power are making decision-making more granular, accurate, and automated, human planning expertise is still enormously valuable. Novel situations like the recent global pandemic, which had little associated data from which to create models, can surely arise again (learn more about an intelligent response to COVID-19 impacts here). Limited data scenarios also arise naturally as retailers reinvent themselves.
Human expertise is important, for example, when retailers plan new store formats or new services. Similarly, many complex issues are best resolved by human planners discussing available options with category managers or suppliers. Finally, planning expertise is essential for translating each retailer’s unique business strategy into the detailed objectives that control how AI optimizes outcomes.
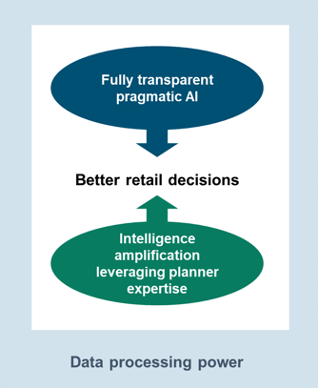
In order to reap the full benefits of planner expertise, retailers need intelligence amplification: the ability to turn data into actionable information and recommendations. This also makes planners’ work more meaningful, allowing them to spend less time on routine data preparation and more time on making decisions. This is why best-in-class planning tools must have powerful analytics built into them. This ensures no time is wasted switching between systems or waiting for reports when decisions need to be made.
4. Ask the Right Questions: How to Evaluate Your AI Options
With clichés like “data is the new oil” flying around, peers trying to impress you with how AI-savvy they are, and technology vendors packaging every calculation beyond spreadsheets as AI, it can be hard to keep track of what is real and what is not when it comes to AI. So how can retailers make sure they’re implementing AI pragmatically to improve the health of their companies?
The only way to make sense of it all is to ask technology vendors the questions everyone in the room is thinking but few have the courage to ask:
- What do you mean when you say this is AI-based?
- What and how much data is required for this to work?
- How is the data used? How does data quality impact the results?
- How is the new method better than the old way of solving this problem?
- Do you have any real-life examples of how this works in practice?
- Can I talk to some of your customers already using this approach?
Each one of us is already engaging with specialized artificial intelligence every day when using navigation applications, browsing product recommendations online, or letting our parking-assistant take over control of our cars. Artificial intelligence is not magic—it’s clever engineering.
As with most technological innovations, you don’t need to be able to program the AI algorithms yourself or replicate the calculations, but you do need to know when it works (and especially when it doesn’t) and why. A pragmatic approach to AI is about solving real business problems to deliver real business value.