Eliminating AI ambiguity: Distinct AI approaches to three top business concerns
Jun 12, 2024 • 11 min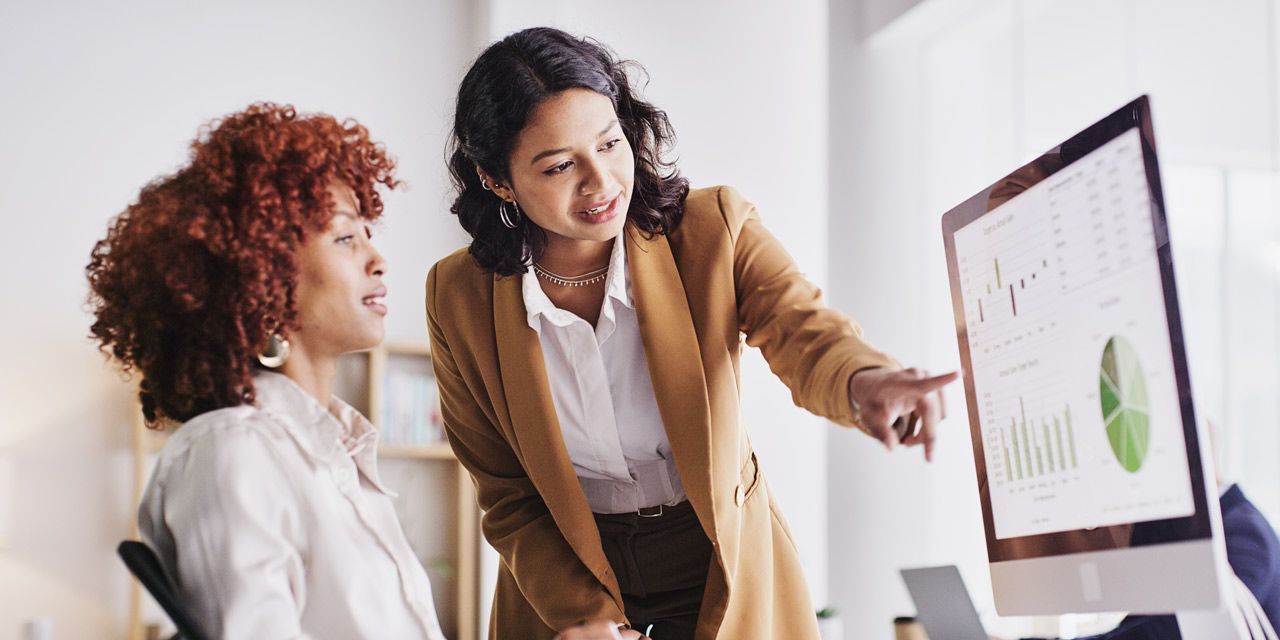
When it comes to retail and supply chain planning, CIOs and IT managers are facing a new, spectacularly vague directive – “implement AI.” This initiative is flanked by the promise of bigger budgets on one side and the risk of failed tech implementations on the other.
So how does one go about “implementing AI” in a methodical way tailored to industry-specific business needs?
There are two common misapprehensions about AI. The first is that AI is its own initiative, a separate investment that is superimposed onto all the other supply chain functions. The second is that all AI is created equal, and any engine will do.
Instead, think of AI as an infusion rather than a superimposition – something woven into the fabric of a planning solution. The more specialized and trained the AI in a specific capacity or sector, the faster the ROI, the more accurate the results, and the more scalable the system.
So what does that look like in practice?
To illustrate, we’ll examine how strategic and tailored AI techniques, native to the RELEX planning solution, help companies meet three of today’s most common business objectives in the retail and supply chain sectors.
Understanding different types of AI
First, let’s define what exactly we’re trying to implement. “AI” encompasses numerous methods and technologies.
“Gen AI” refers to generative systems (like ChatGPT) that serve more general-purpose use cases. In the business setting, the most common type of Gen AI involves large language models (LLMs) that easily collect, summarize, and distribute knowledge.
“Specialized AI” refers to algorithms with a narrower focus than Gen AI. Specialized AI is trained to perform a specific function at a superhuman level. This includes advanced “machine learning” and optimization algorithms, which leverage massive amounts of industry data to perform specific, complex tasks and generate highly accurate output rapidly.
RELEX uses each type of AI defined above to serve a unique function in the supply chain environment and deliver distinct advantages.
Objective #1: Recalibrating forecasts to shifting consumer behaviors
Trends and fads are always coming and going. Some are more consistent, varying with seasons and cyclical patterns. Others are inherently flighty, disappearing as rapidly as they arrived. Both types can leave retailers rushing to fill suddenly empty shelves or watching the dust settle on their rapidly aging inventory.
Now inflation is adding a twist to the challenges of anticipating consumer demand. According to McKinsey, 75% of consumers are trading down in their buying habits (e.g., adjusting how much they buy or holding off on spending for extended periods of time).
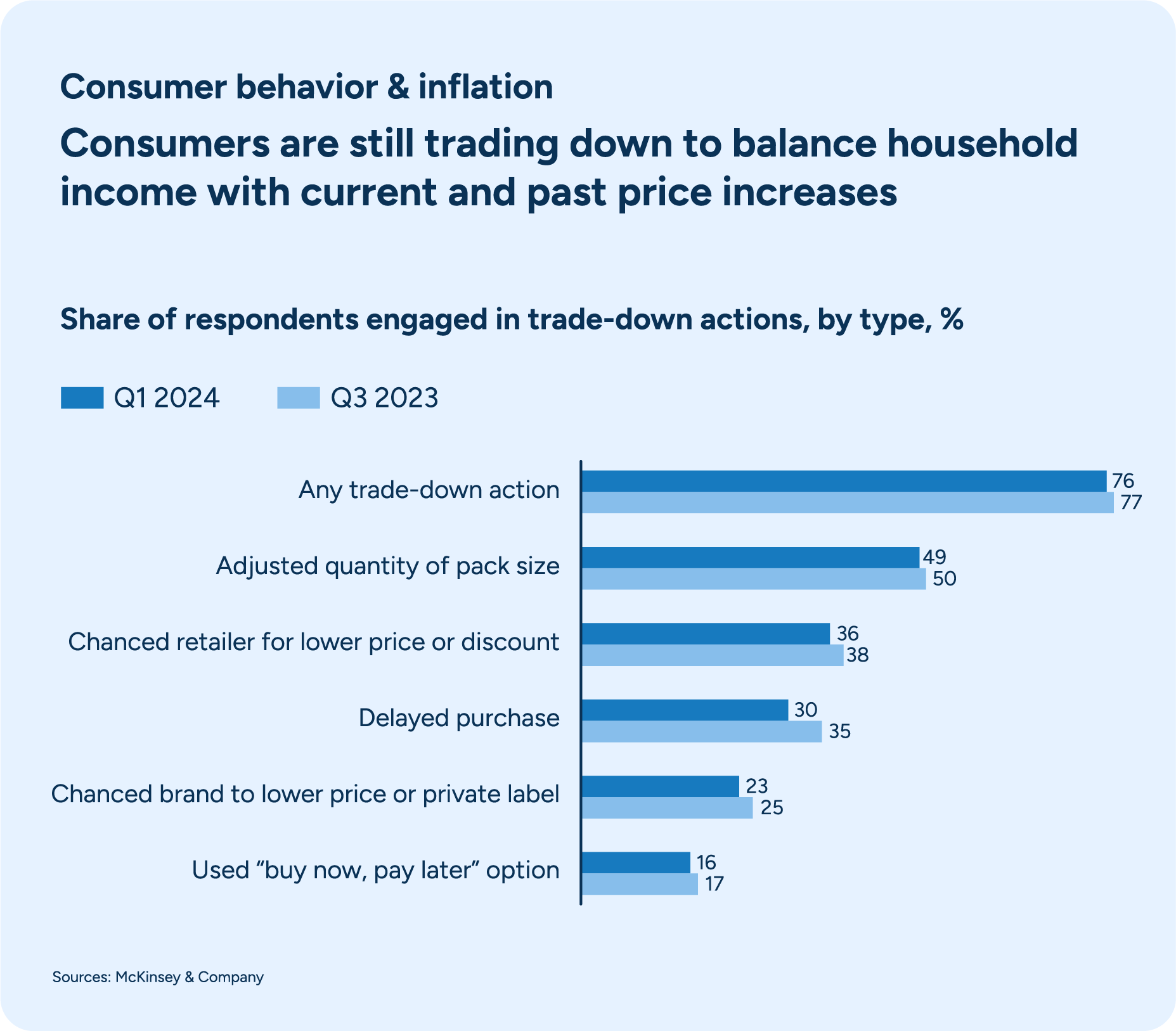
Shoppers are growing more selective about purchases, and retailers cannot afford to hold unproductive inventory.
Planners must quickly absorb consumer demand patterns and trending behaviors into their calculations for accurate forecasts, and category managers need to know which product mixes will best appeal to shoppers.
In this scenario, AI delivers a one-two punch so retailers can accurately shape their forecasts and their assortments.
Machine learning-based forecasts
Over the past five years, we’ve been developing and refining our machine learning forecasting model to help our customers achieve greater planning agility, easily spotting outliers and identifying fast-approaching trends.
This AI-based solution analyzes three factors:
- Demand patterns such as seasonality, trends, and weekday-related demand variations.
- Business decisions like promotional plans and changes in display or space.
- External events including holidays, weather, footfall, and competitor activities.
With a comprehensive picture of demand across all product types, retailers can increase availability and optimize inventory. By quickly incorporating more high-quality demand data, ML-based solutions create forecasts that outperform traditional methods in speed, accuracy, and granularity.
RELEX integrates three types of machine learning algorithms into its forecasting solution so customers can manage the inherent scarcity and volatility typical of data in retail and CPG environments.
- Automatic variable & parameter selection and data cleansing: Our machine learning algorithms identify the most critical demand-influencing factors and remove errors from the data to ensure the solution uses only clean data for highly accurate calculations.
- Change point detection: This capability allows businesses to react swiftly to drastic demand shifts caused by unknown variables and events (e.g., competitor stockouts or store closures), adapting forecasts quickly and accurately.
- Data pooling: Many retailers have a portfolio of slow-moving items with sparse sales data. By pooling data for products with similar demand behaviors and product attributes, retailers can create highly accurate forecasts with limited data.
These ML solutions automate planning drudgery, improve data insights, and help our customers achieve better adaptability and scalability.
For example, WHSmith, one of Britain’s best-known convenience retailers, uses this machine learning solution to forecast its on-the-go sandwiches, salads, and pastries in their airport locations. By incorporating forecasted airport passenger numbers into their sales forecasts, WHSmith can better understand how foot traffic patterns will shift. It aligns its forecasts with these anticipated peaks in demand, significantly reducing spoilage and increasing efficiency.
Read more: WHSmith optimizes food-to-go and weekday profiles
Assortment planning
RELEX Assortment planning is a unified approach to analyzing, planning, and managing assortments through the category review cycle. We’re excited to introduce new AI developments that deliver customer-centric assortments, helping retailers improve efficiency, profitability, and the customer experience.
Assortment rationalization
Assortment rationalization has transformed from an optional capability to table stakes. But it calls for a methodical approach supported by the right data, insights, and technology.
The RELEX solution now contains AI-supported rationalization that spots end-to-end profitability for each item per store, highlighting poor performers.
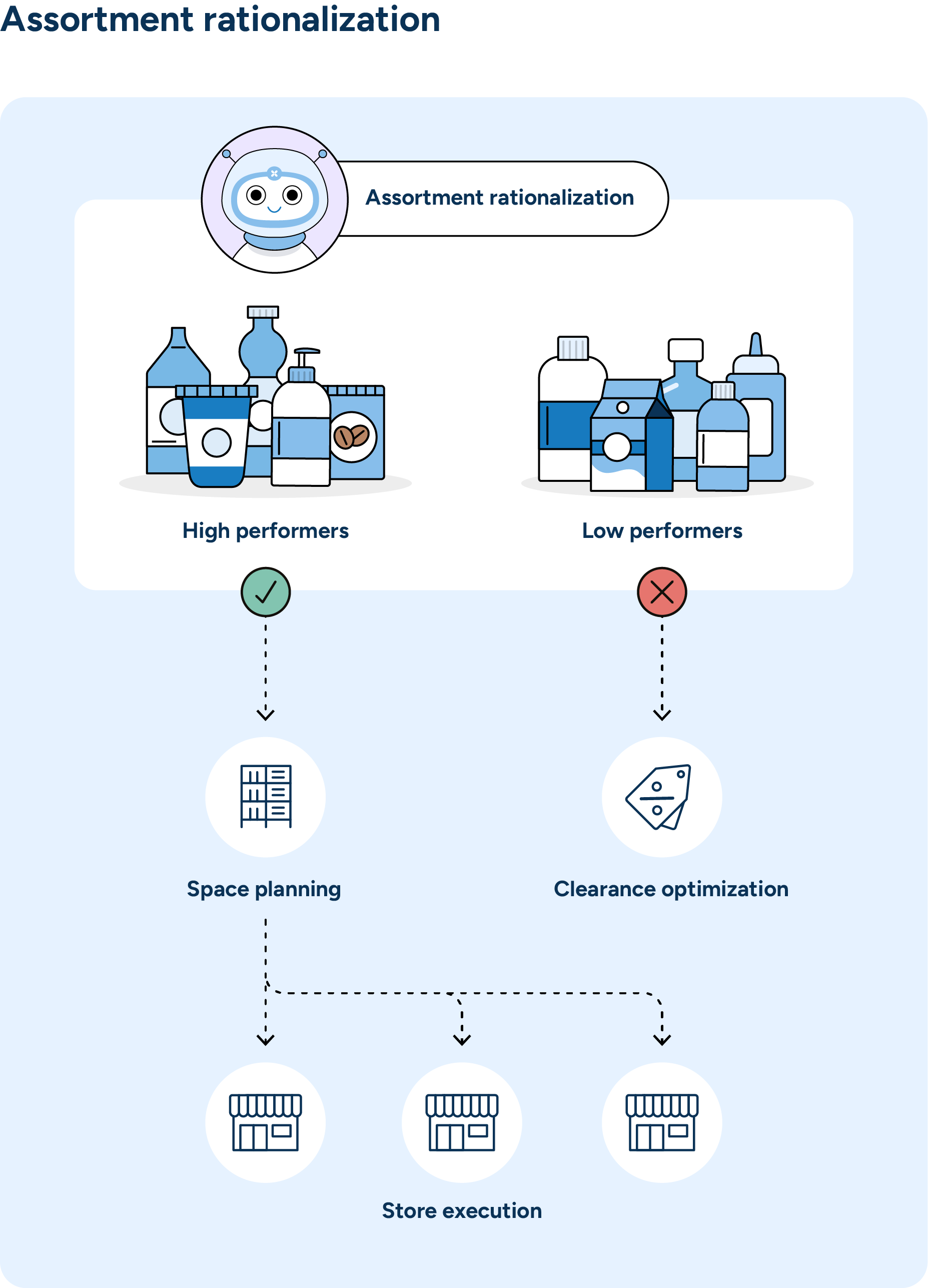
Because all retail functions and data insights are hosted on a single, unified platform, retailers can immediately act on these insights and remove underperforming items from the assortment, executing clearance plans smoothly while moving approved assortments on to space planning. This limits waste and margin erosion while satisfying shopper expectations and enhancing profitability.
Applying new optimization methods
Assortment optimization is as much an art as it is a science. Category managers and assortment planners must ask themselves:
- How much overlap should there be between products?
- Is duplication consuming valuable space better allotted to a different item?
- Are current strategies safeguarding customer satisfaction or risking overstock, obsolescence, and expiration?
A retailer’s business strategy and vertical will very much determine its approach to categories and assortments.
To help our customers refine their categorization strategies further, our data scientists at RELEX Labs are developing a new assortment optimization capability. It applies advanced AI methods to customer behavior patterns to identify product families that traditional methods miss.
The process begins by assessing product sales and basket data without incorporating any category information. RELEX uses neural networks to analyze this POS data, cluster items, and delineate new categories. These networks outline which products are substitutes for each other and which complement each other.
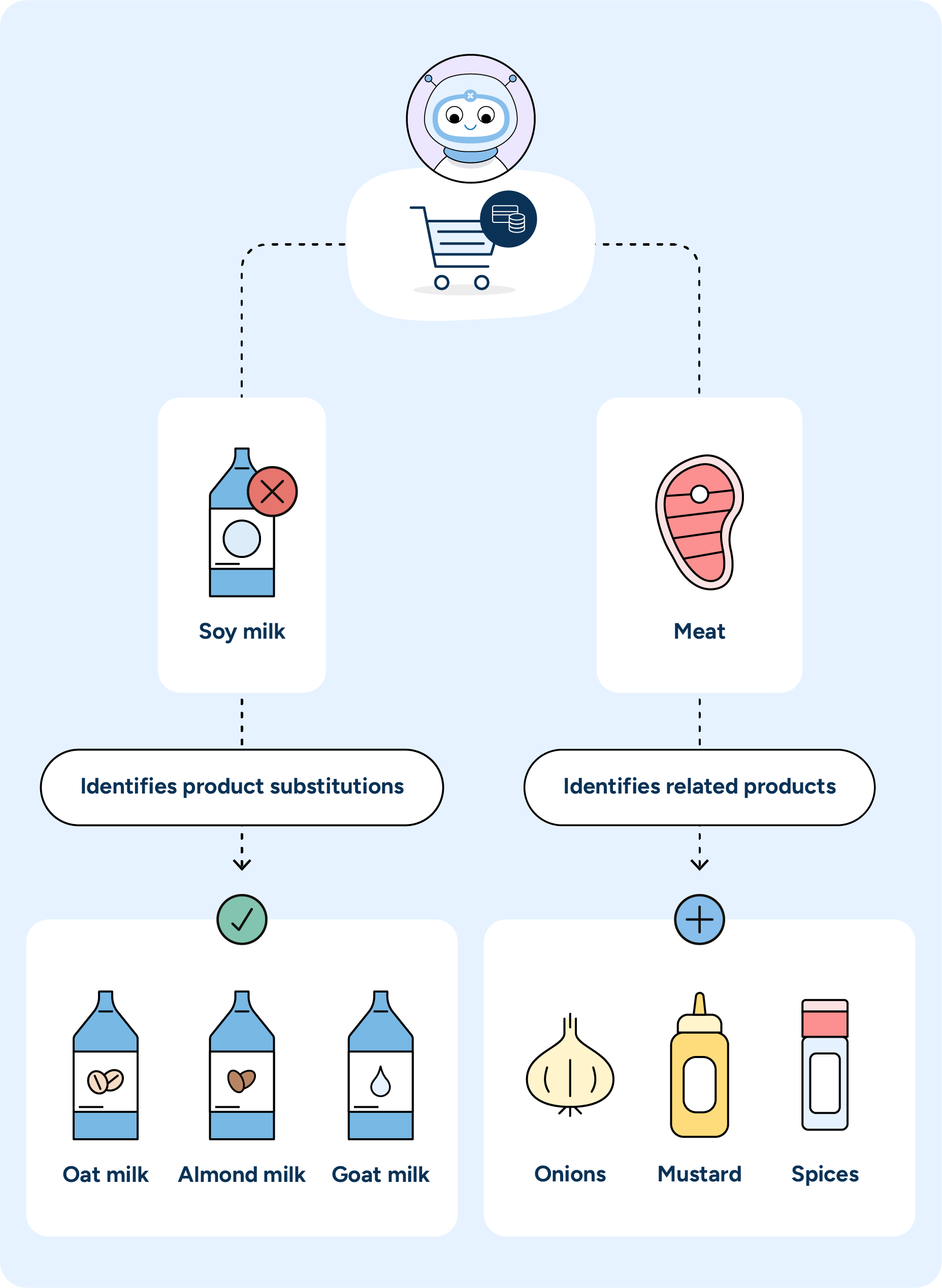
This helps retailers identify products that differentiate them from the competition and items that drive overall profitability (even if they don’t have high margins themselves) by contributing to basket growth.
Deep neural networks and transfer learning techniques automatically identify relationships between products to develop and refine high-profit, customer-centric categories.
Looking ahead, this AI technology will also improve ways to model demand transfer. For instance, if most of the demand for Item B can transfer to Item A, retailers can drive incremental sales by eliminating Item B from the assortment and adding a unique product to the newly available space. They can also better optimize the existing products for improved availability and more efficient replenishment.
These assortment methodologies will also help suggest customer decision trees for space planning and provide more insight into customer shopping missions, cannibalization, and halo effects.
Objective #2: Improving efficiency, availability, and sales
When it comes to in-store inventory counts, traditional cycle-counting just isn’t cutting it anymore – and the numbers don’t lie. Retailers globally lose an estimated $1.1 trillion in lost sales due to hidden stockouts. One study shows that 60% of the products analyzed were affected by inventory record inaccuracies.
At the store level, inventory adjustment can be counted and recounted frequently in very short time spans, wreaking havoc in upstream planning. This often results in phantom inventory, a discrepancy between how much inventory a store has on hand and the (usually higher) inventory levels recorded in the planning solution. This leads to lost sales and inaccurate forecasting calculations, eating away at margins before retailers can recognize and address the stockout.
Trying to capture phantom inventory without the right tools can force store employees into frequent, manual inventory checks, unguided by data insights or strategic task prioritization.
Retailers need more accurate inventory counts so they can enhance forecasts and order proposals while avoiding frequent manual checks in stores. Predictive inventory is the answer.
Predictive inventory
RELEX predictive inventory works across sectors, from grocery to DIY, tackling challenges from fast-moving perishable fresh produce to slow-moving items like refrigerators.
It uses machine learning (trained on data from some of the largest grocery retailers in the world) to identify likely inventory miscounts. Machine learning monitors sales data, looking for sudden drops in sales for items that should have on-hand inventory. It then recommends targeted inventory checks to store associates.
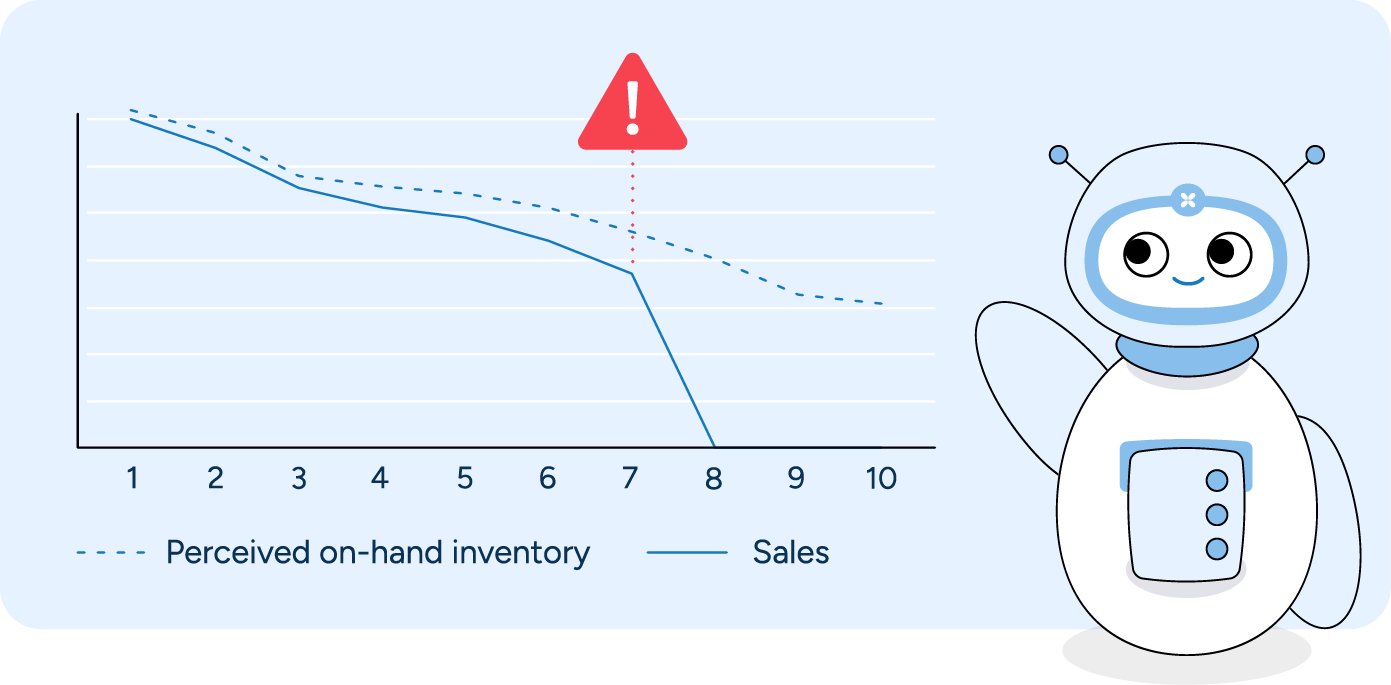
The sooner store associates identify a hidden stockout, the sooner they can correct it, feeding more accurate data into the machine learning algorithms that govern forecasting.
This reduces unstructured, labor-intensive inventory counts, freeing up associates for customer-facing tasks. Plus, more accurate balance data helps continuously refine forecasts to fit consumer demand and increase sales and profitability.
Objective #3: Using Gen AI for daily efficiency improvements
Gen AI – the greatest thing since sliced bread and the biggest eye roller of the last decade. Its usefulness comes down to who has developed the engine you’re using and how you apply it to the daily grind.
Many companies turn to generic options that produce instant answers. But are they the right answers? Do they speak to your particular industry? Your challenges? Your business-specific needs? Do they provide daily guidance and planning insights?
Say hello to Rebot
This is where we’d like to introduce Rebot, our in-house Gen AI copilot.
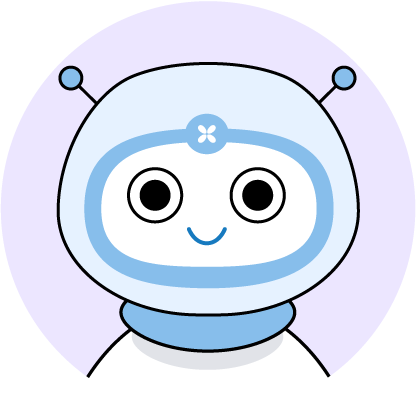
Rebot uses the Azure OpenAI platform but indexes RELEX knowledge separately. After rigorous testing, we’ve made it available to customers, many of whom are already using it daily.
In its current iteration, Rebot answers questions about our products and supply chain strategies for practical guidance in day-to-day operations. Over time, we’ve built a knowledge base of RELEX experience and expertise, recording every best practice development as we go. This continually updated knowledge base feeds Rebot all the information it needs to recommend a plan of action. So if a user asks, “How should I do seasonal planning for a peak period?” they receive an instant answer, based on our best practices.
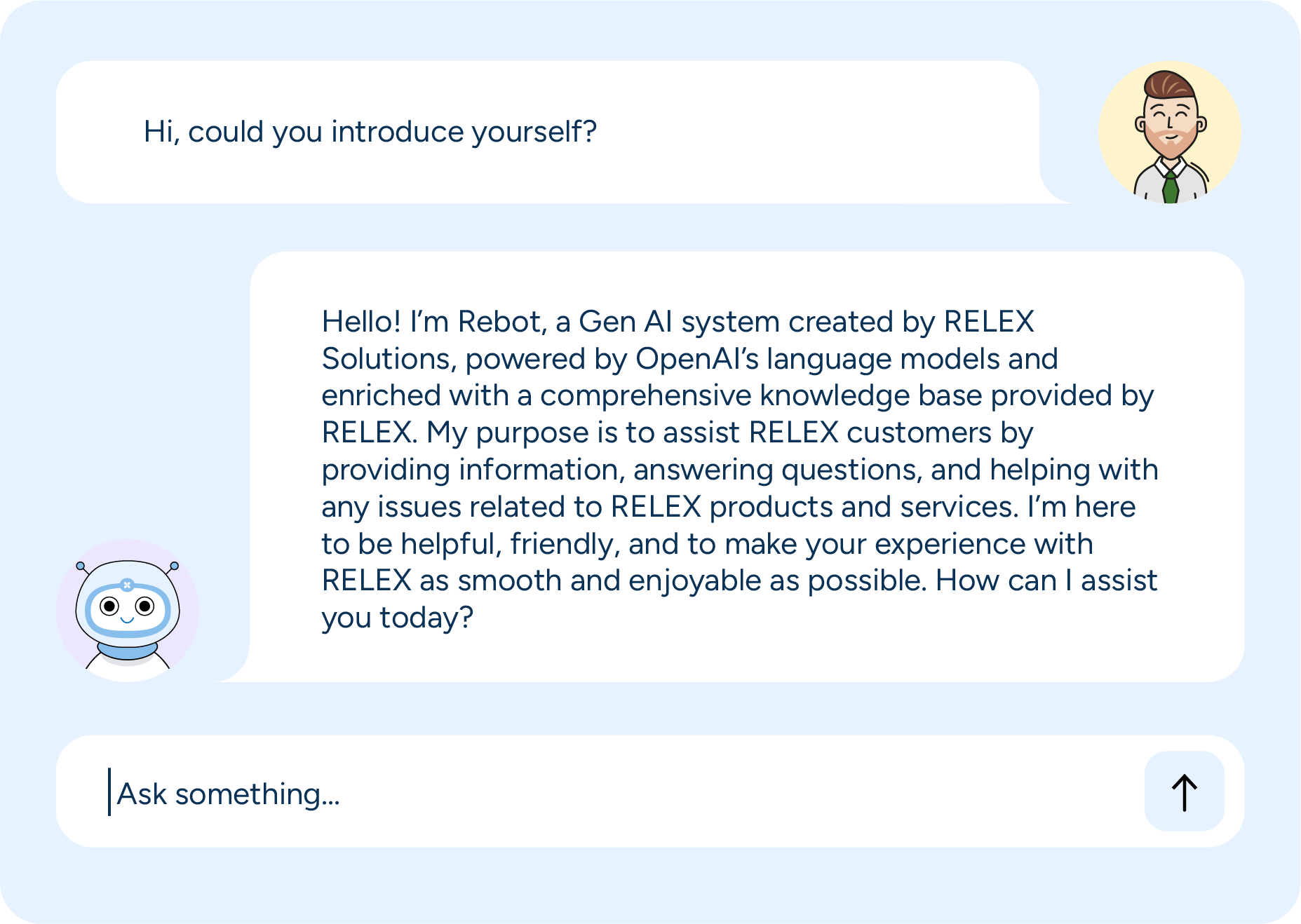
But we’re even more excited about what our developers have coming.
Our next step for Rebot is to blend our internal information sources with a retailer’s operational data to form one integrated knowledge base with which customers can interact. Rebot will not store or train with customer data. Instead, through configurations and a specially adapted API, it will reference company data only while answering queries. In this way, it will be able to analyze that data and recommend action – securely and under customer control.
This will allow users to ask questions specific to their business and receive highly tailored answers. For instance, using live production data, a user can ask Rebot, “For this upcoming seasonal plan, are there any areas projected to have lost sales? How can I improve the plan to mitigate that risk?” And Rebot will start making customer-specific recommendations.
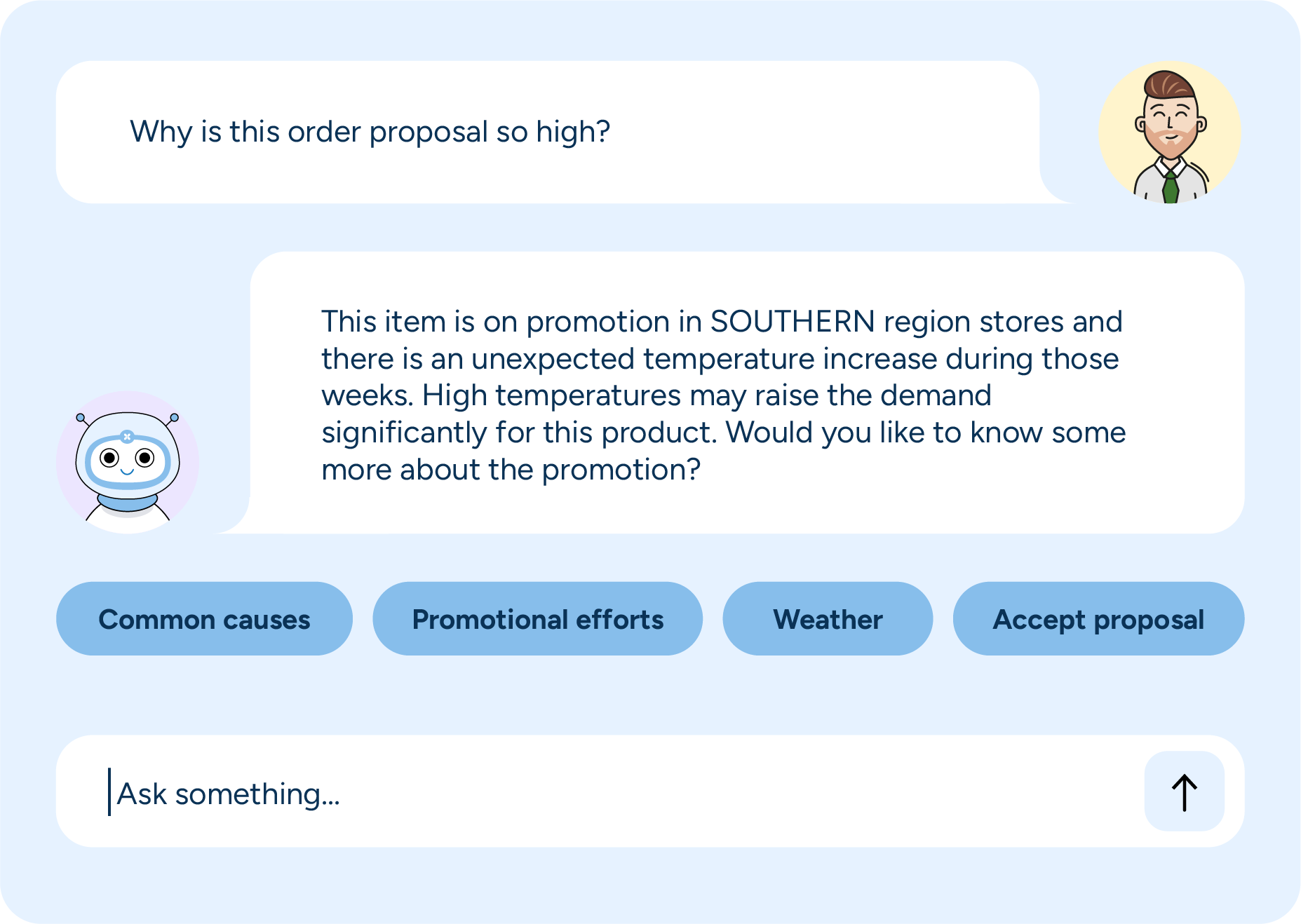
Rebot has fast become a daily go-to for both RELEX customers and employees. It’s a way to grab easily digestible information quickly and continuously deepen personal learning. This also makes it extremely valuable when onboarding new employees and users. We’re continuing to develop the system, advancing its capabilities as a copilot and integrating it into products as part of our development roadmap.
Benefits of integrating different AI functions through a resilient, unified platform
Individually, these AI applications deliver considerable value.
- Automation reduces workload and errors.
- Keener data insights generate better forecasts.
- Better on-shelf availability increases customer satisfaction and sales.
- Optimized inventory reduces waste and increases margins.
- Efficiency improvements streamline planning, operations, and onboarding.
But these AI functions work to the greatest advantage when they work together.
Our unified platform brings all your planning functions under one roof so companies can boost profitability while delivering premium consumer experiences. From forecasting to assortment, pricing to store execution, teams can easily share data, communicate plans, and alert each other to anomalies or disruptions. AI insights, shared data, and better visibility empower users to find opportunities to improve efficiency and make optimal daily decisions.
How to implement AI strategically with RELEX
Returning to the original directive to “implement AI,” the answers do not have to be as vague as the question. A knowledgeable technology partner has the solution, strategy, and experience to help companies identify the specific AI applications that best suit their challenges.
At RELEX, every one of our customers already uses AI because it’s not new to us; we’ve been building it into our solutions from the get-go. By dedicating tremendous resources to our R&D, we ensure that the companies that partner with us never get bogged down in buzzwords but never miss out on the latest advancements. By equipping them with the knowledge, technology, and speed they need to stay efficient and competitive, we’ve helped retailers, wholesalers, and consumer goods companies reap impressive benefits in inventory management, profit margins, and customer satisfaction.