Machine learning in the context of retail demand forecasting
May 29, 2019 • 5 min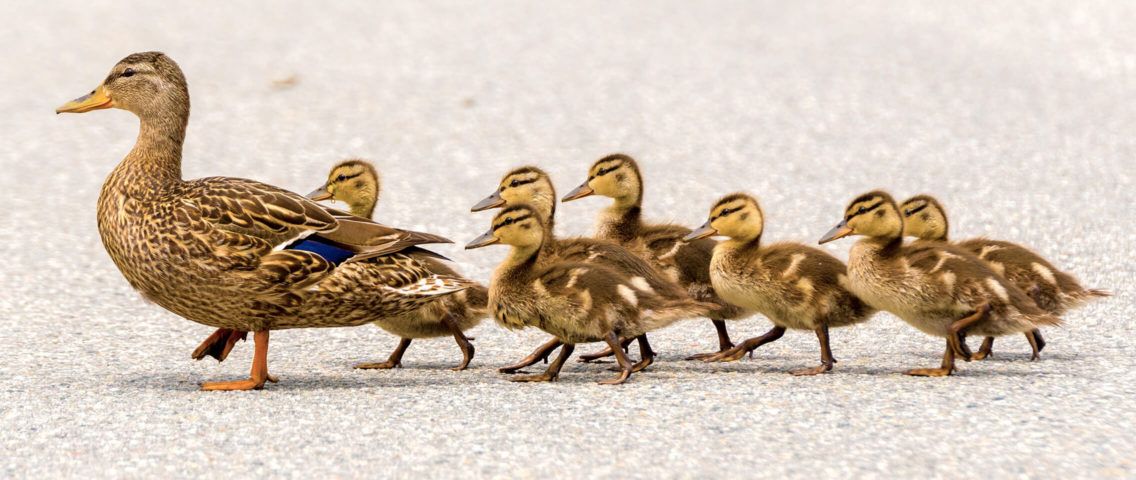
The tech industry has been buzzing about machine learning for a few years now — it seems every week, companies introduce new ways to exploit it to transform their businesses. It comes as no surprise, then, that leading retailers who recognize the competitive advantage have turned to machine learning to transform their planning as well. But what exactly is machine learning, and how do we utilize it in retail planning? Let me start with an example.
Imagine a retailer that operates at airports, providing fresh food products. Their demand fluctuates not only from day to day, but also throughout the day depending on footfall numbers at the airport. Manual entry for this sort of data would be wildly time consuming — not to mention that it would almost guarantee an error-prone forecast.
A machine learning algorithm, though, with access to airport data could automatically recognize the relevant footfall patterns and apply those trends toward the retailer’s demand forecasting, all without the need for any human programming. This is exactly how RELEX helped WHSmith, one of Britain’s leading retailers, drive significant improvement in its forecast accuracy. It’s a straightforward solution, but a powerful one.
There’s a lot of hype around the topic at the moment, but it’s important to remember that machine learning is by no means a fix-all solution. At the end of the day, machine learning is just math, and often quite simple math at that. That’s why it’s so important to take the time to fully understand the challenge you’re trying to solve — because different challenges require different methods. A smart retailer will recognize that real-life problems can never be solved with just one solution, not even one as powerful as machine learning.
Machine Learning Is Not Programmed, but Taught with Data
Machine learning gives a system the ability to automatically learn and improve from data without any programming. The system is fed data, such as examples, direct experiences or instructions, in which to look for patterns. Going forward, it can use the patterns it identifies in the data to make better decisions. In short, the learning algorithm eliminates the need for human programming by automatically generating a program/model based on the data it’s provided (Expert System).
The primary benefit of machine learning is that the system can process large amounts of data from a variety of sources without the need for human labor. The value you get from machine learning, though, will be a function of the quality and quantity of the data you feed it.
Practical Examples of Machine Learning in Retail
In the airport retailer scenario highlighted above, machine learning was used to identify the relationship between an external factor (footfall) and local demand per store and product. But machine learning can take a variety of external factors into consideration in a retail environment.
Weather
Weather, for example, is also a significant source of fluctuations in consumer demand. While predicting weather may be difficult, it’s actually quite simple to create rules or models based on the relationship between demand for a product and weather elements such as rain, hours of sunshine, temperature and so on.
However, when looking at your entire assortment, this simple task grows quite laborious. You need an automated way to process data in such vast quantities. This is especially true when you realize that the process must look both at the future and the past. An accurate and robust baseline forecast will have filtered out the effect of weather from historical sales data as well.
When these processes are automated, retailers can proactively plan for increases or decreases in local demand, assuming they have sufficient product availability. In grocery retail, taking weather effects into consideration can reduce forecast errors by between 5% and 15% for weather sensitive products and by up to 40% on the product group and store level. When a supermarket in Britain used RELEX’s weather corrections during an unusually cold and rainy summer, they were able to increase forecast accuracy for their weather-sensitive products to over 90%.
Cannibalization
Whereas weather can have a variable effect, product promotion always has a direct positive impact on the sales volume for the promoted product. Too often, though, retailers neglect to take into consideration the impact promotion of one product can have on sales volumes for other, non-promoted products. When the increased demand for one product results in the decreased demand of another, it is called cannibalization. Cannibalization can, for instance, occur between two types of ground beef: When one is on promotion, increased consumer preference for the promoted ground beef results in a decrease in demand for the other. Taking relationships such as this into account significantly improves forecast accuracy during promotions that have cannibalization effects, decreasing the risk of spoilage for cannibalized products.
It may seem easy at first to predict how sales promotions affect shopping behavior, but what happens when you’re sifting through data for thousands of products? How do you recognize the relevant relationships out of millions of possibilities? The most practical solution is to use machine learning techniques that automatically recognize these relationships based on historical sales and promotional data.
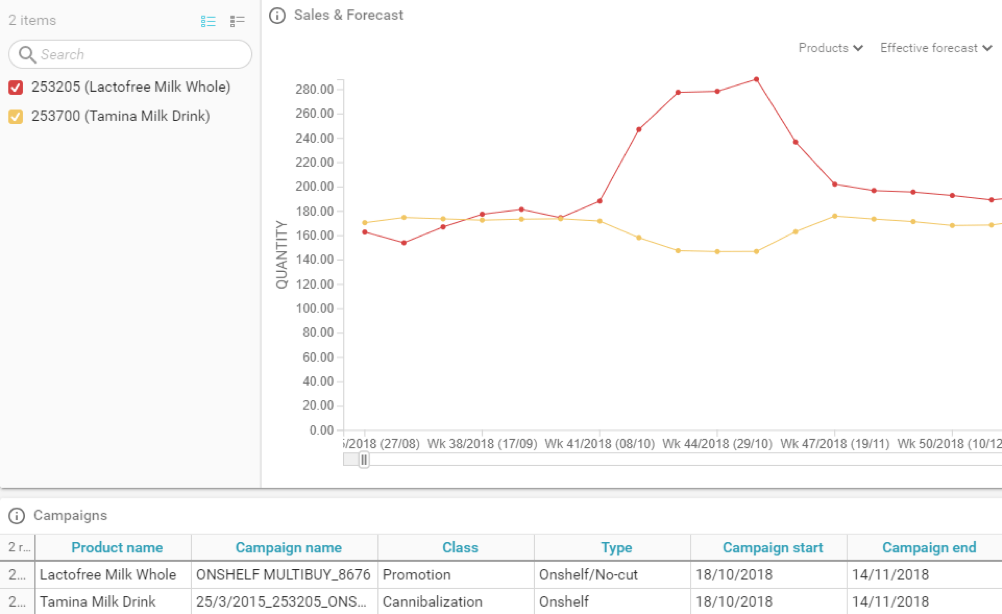
Use a Combination of Tools for the Best Results
In retail planning, demand forecasting is an obvious application area for machine learning. Because we serve all planning horizons with the same forecast, we employ a layered forecasting approach:
- Time-series forecasting for reliable baseline forecasting that supports long-term planning
- A combination of causal modelling and machine learning to capture the medium-term impact of, for example, promotions and cannibalization
- Machine learning to model the impact of external factors (such as weather) typically not known much in advance
Different forecasting methods have their own strengths and weaknesses in terms of stability vs. reactiveness, demands on access to data, planning horizons and computational efficiency. It makes sense, then, that we attain the best results by using a combination of methods.
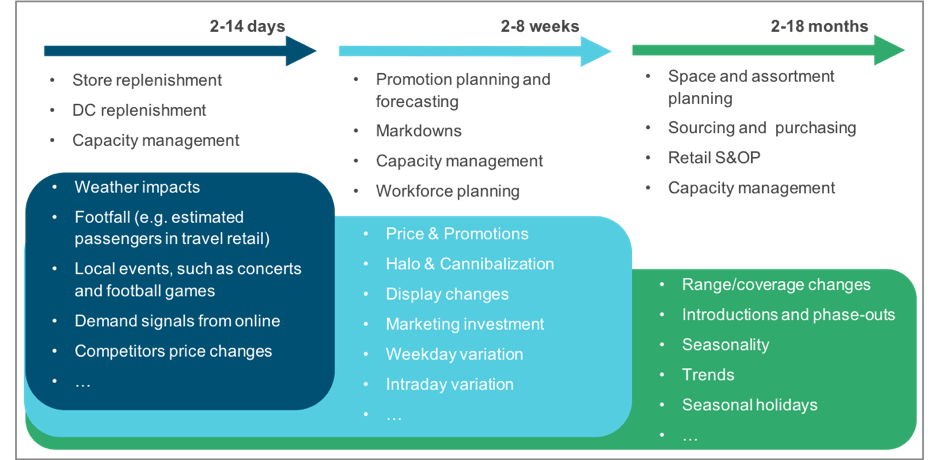
The Keys to Developing Machine Learning Capabilities
To truly benefit from machine learning techniques, you need exceptionally large datasets — but large datasets require outsize processing power. At RELEX, we have a mutually beneficial relationship with our customers in that retailers can always provide us with a lot of data and we can provide our customers with the solid technological foundation of our in-memory data platform and data integration capabilities. Working together, we’re in a position to develop excellent machine learning techniques. The RELEX platform provides our retail customers with the superior speed and performance necessary for running machine learning calculations, even with their exceptionally large datasets.
We’re also constantly examining and experimenting with new methods at RELEX, and we currently have a team dedicated to working full-time on machine learning and analytics-related research. At the moment, our R&D team is researching how neural networks with multiple data sets can benefit our customers, developing Bayesian regression modeling of multi-level sales patterns, and exploring the use of classification algorithms to further improve forecasting of new products.
Machine learning is clearly here to stay. But instead of focusing on just one method that seems on-trend, it’s important to consider what the challenge in front of you really is and what tools will best help solve it. We believe that once the hype dies down, machine learning will be business as usual, applied in combination with complementary methods to provide the best possible solutions to the most complex problems in retail planning.