How to turn local teams into highly accurate fresh replenishment experts: using technology to augment local knowledge
Aug 9, 2022 • 5 min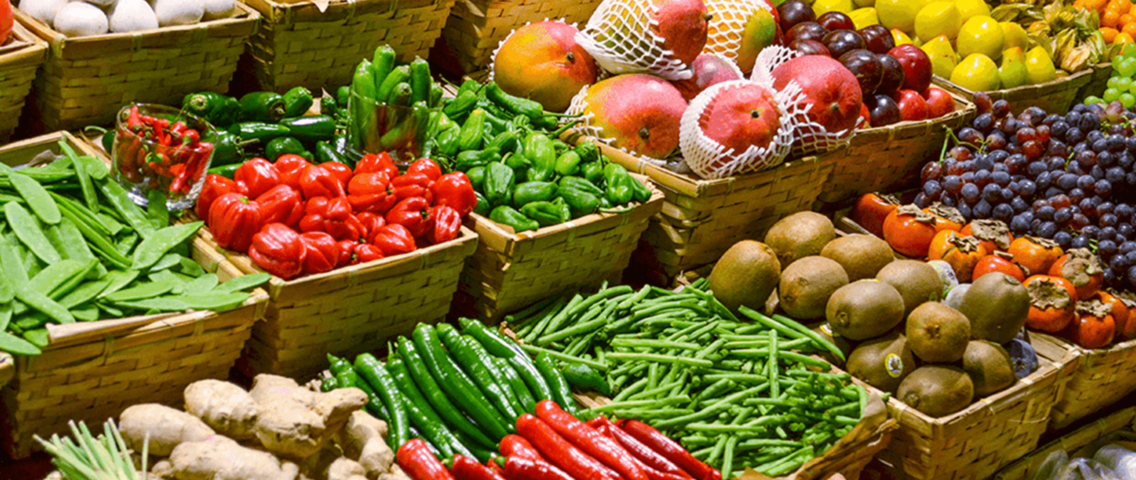
For many grocery and convenience retailers in the United States, a local team makes local decisions about the management, ordering, and replenishment of fresh items—including produce, dairy, and bakery—based on their local knowledge. We often assume that because local teams know their communities, they’re best positioned to place orders based on external factors like events and local demand for specific products. They’re also physically able to inspect each shelf to determine what needs to be ordered and when.
Replenishment is one of the most important tasks for any fresh department, but it’s also the most difficult. Furthermore, many store-level teams are assessed and incentivized based on their location’s revenue and margin, so teams are motivated to maximize sales.
Of course, there are benefits to ordering at a local level, including the ability to take advantage of staff members’ expert knowledge. But there are drawbacks as well — it’s a heavily manual, tedious process that requires a great deal of time to review inventory, plan replenishment, and write orders.
Modern Challenges Require Modern Solutions
Fresh replenishment is a skilled position that requires a high level of training and experience in order to successfully – and profitably – manage the category. However, with the current worldwide labor shortage, fewer people are available to handle in-store operational tasks and fresh replenishment planners must balance their workday across these and other critical work.
With staff spread thin, grocery management tasks simply can’t receive an appropriate level of attention, and it’s more likely that there will be errors or miscalculations. Considering these challenges, retailers need AI-driven technology to augment their local, human expertise.
Errors related to the management of fresh items can be extremely costly—a major concern as prices rise and customers review their grocery spending closely. If a local team orders too little of a fresh product, the store risks losing sales, which results in dissatisfied or even lost customers.
However, if a local team orders too much of a fresh product, the store risks spoilage, which is both a financial loss and an increase in waste at a time when sustainability is a major initiative for many retailers. There’s no buffer with fresh items—unlike with center store products, fresh overstock can’t be stored in a back room or distribution center until it’s needed because it will spoil.
While the local team can mark down some fresh products that are nearing the end of their shelf life, this can only be done if there is a best-by date on which to base this decision. Most produce, however, does not have a specific indicator but spoils piece by piece, with multiple parts of a batch spoiling at different times. Manual stock rotations, uneven spoilage, shrinkage, and lack of quality inventory data bring a large amount of complexity to fresh replenishment.
AI + Human Intelligence = Fully Optimized Fresh Assortments
To improve the management of fresh products and cut down on manual, repetitive, and low-value tasks, local teams need access to forecasts that accurately capture demand variation while ensuring a high level of visibility of on-hand fresh inventory.
A technologically advanced system can use an enormous amount of information—far more than any individual or even local store team could process—to create these forecasts automatically.
With access to this broader data pool, which can include critical details like supplier availability and regional demand trends, store teams can improve and automate their fresh orders while also ensuring the quality of the items on their shelves. Retailers who take advantage of AI and machine learning technology to process their data can automatically access:
- Highly accurate demand forecasts that take demand-influencing factors like local weather forecasts and upcoming events into account
- Inventory level predictions that take into account spoilage, shrinkage, and consumption
- Smart order recommendations of the right amount of stock at the right time
- The ability to review and amend order recommendations at the local level as needed
AI and machine learning technology allow retailers to augment their store teams’ local expertise. It should be noted that while human insight will never be replaced by AI, humans can make good use of AI to improve the data needed for an automated process.
Beyond Local Fresh Management: How Technology Can Reduce Risks in Your Supply Chain
A solution that considers not only the store orders but also the end-to-end supply chain, allows retailers to dynamically collaborate with suppliers. This enables stores to make simultaneous orders from multiple suppliers which can reduce supply chain disruptions, ensure availability, and provide opportunities to make economically priced bulk orders.
Supply shortages in fresh assortments are a common problem. However, with smart allocations, scarce supply is automatically distributed to stores based on their demand forecasts. In this way, stores with the greatest sales potential and demand will get more products.
For example, if a store’s demand for Gala apples is larger than what the supplier or grower is able to deliver, a smart allocation system can automatically determine alternate suppliers and the proper quantity of Gala apples to be delivered to the distribution center, which will then deliver apples to individual stores based on their demand forecast. A best-in-class allocation system will also consider batch dates, ensuring stores can maximize the shelf life of their fresh assortments.
Above all, it’s important to work with an end-to-end system that does more than simply manage fresh products in the store. Fresh management should be one part of a robust system that handles many other tasks, such as the management of shelf-stable items alongside fresh items, capacity flows, and even store operations workloads. An AI-driven solution takes full advantage of all the data it has access to, providing improved delivery patterns and optimized store operations.
Technology Builds Collaboration, Efficiency, and Job Satisfaction
Retailers that rely on local ordering processes don’t need to dramatically change how they do business. They simply need access to a system that provides local stores with data in a format they can use to better plan their fresh orders. This will allow each store to ensure product availability and reduce spoilage while saving both time and money.
When store personnel have the tools to be successful, once-dreaded daily tasks become enjoyable and employees with varying levels of experience and skills are able to perform tasks with expert precision. Even in unexpected circumstances, an AI-driven system ensures local teams can remain nimble and make additional orders on the fly to ensure their customers receive optimal service levels and access to the freshest foods possible.
Technology will never replace human insight and experience. What it can do, however, is augment your local team’s knowledge and handle your store’s complex data, providing visibility and transparency that allow for real-time corrections and improvements if needed. It’s a win-win-win situation for your customers, employees, and the business itself.