Best practices in weather-based sales forecasting
Feb 15, 2018 • 3 min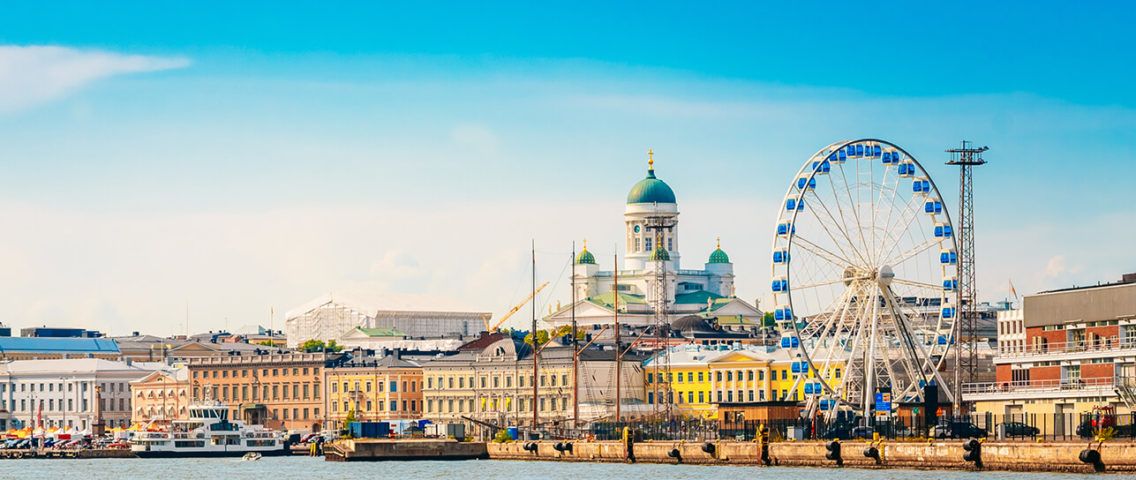
Weather is a source of significant fluctuations in consumer demand. One day might be hot and sunny while the next is grey and filled with rainclouds. Predicting weather is not easy and rapid changes in weather can cause consumers to suddenly shop or cease to shop for specific products, which can quickly become costly for a retailer.
Because of the bullwhip effect, changes in weather can also produce unnecessary high fluctuations on the supply side: If you prepare too extensively you might end up breaching the capacity limitations at every level of you supply chain and increase your fresh goods spoilage but if you fail to prepare sufficiently it can also lead to significant lost sales.
Because of the bullwhip effect, changes in weather can also produce unnecessary high fluctuations on the supply side.
It’s important to know that the lost sales don’t only apply to products that go out of stock – especially during extreme weather conditions when customers are more likely to make their decisions on which store to visit based on the availability of some key product, for example bottled water during hot temperatures.
While predicting weather is difficult, it’s relatively easy to come up with rules or models when thinking about typical examples of relationships between demand and some weather elements: high temperatures impact ice cream sales, rainfall increases umbrella sales and so on. However, when looking at the entire assortment it becomes more complicated. How can products that react to weather be separated from products that don’t? How do you measure which products react to which weather element (e.g. temperature, sunshine, cloudiness, rain, snow) and how much does it react?
In my recently written whitepaper you’ll find out how retailers can optimally prepare for weather-related fluctuations, but in order to understand the basics, I’ve gathered some best practices in weather-based sales forecasting:
1. Build weather-based sales forecasts on top of baseline forecast
By building weather-based sales forecasts on top of stable baseline forecasts, you allow the weather model to concentrate on weather effects and also give visibility on what part of the forecast is coming from which forecast model.
This leads to several benefits in the actual use of weather models: different models and their outputs can be separated to for example quantify whether the weather model really improves forecast accuracy. On the other hand, different models and their outputs can be compared transparently so that e.g. in the case of upcoming large weather-based forecast uplift, the uplift can be put into context to verify if it makes sense or not, and whether the retailer should act on it.
2. Look at weather models at different levels
We recommend building a weather-based sales forecasting process that looks iteratively at models at different levels, so that each product in each store gets the model that fits its data best.
The SKU-store combination is the lowest level and usually most accurate as well. So, in theory each product in each store can get a separate model, because each product and each store can have different responses towards weather. Especially, store location can make a big difference. For example, when comparing the weather reactivity in a store in some central location with lots of tourists to that of a hypermarket in a location only reachable by car, usually the central city location is much more weather reactive than the hypermarket.
3. Consider sophisticated weather effects
In addition to raw weather data, best results are achieved when some of the more sophisticated weather effects are considered. Weekday variation is something worth considering. On top of normal weekday variation, weather reactivity can differ from weekdays to weekends.
Another thing to consider is past and future weather conditions. Responsiveness towards weather is usually stronger for example on the first sunny weekend of the spring than a sunny weekend in the middle of the summer when there has already been plenty of sunny weekends. Consumers also tend to plan ahead based on forecasted weather, and thus shift their purchases to earlier in the week in the case of an upcoming nice weekend. It goes without saying that nonlinearities in the weather effects need to be considered. For example, it’s possible that high temperatures increase demand, but extremely high temperatures start to decrease the demand, because consumers stay home.
With sophisticated solutions, the whole process from fetching weather data to weather-corrected forecast calculation can be completely automated.
With sophisticated solutions, the whole process from fetching weather data to weather-corrected forecast calculation can be completely automated. RELEX has built-in integration to a global weather data provider where data is fetched based on store coordinates and it can also be easily integrated to any other weather data provider, should it be necessary.
If you want to learn more about how retailers can optimally prepare for weather-related fluctuations, read my whitepaper ‘Towards a weather-proactive supply chain‘.