Improve demand forecasting accuracy by factoring in weather impacts
Nov 3, 2020 • 7 min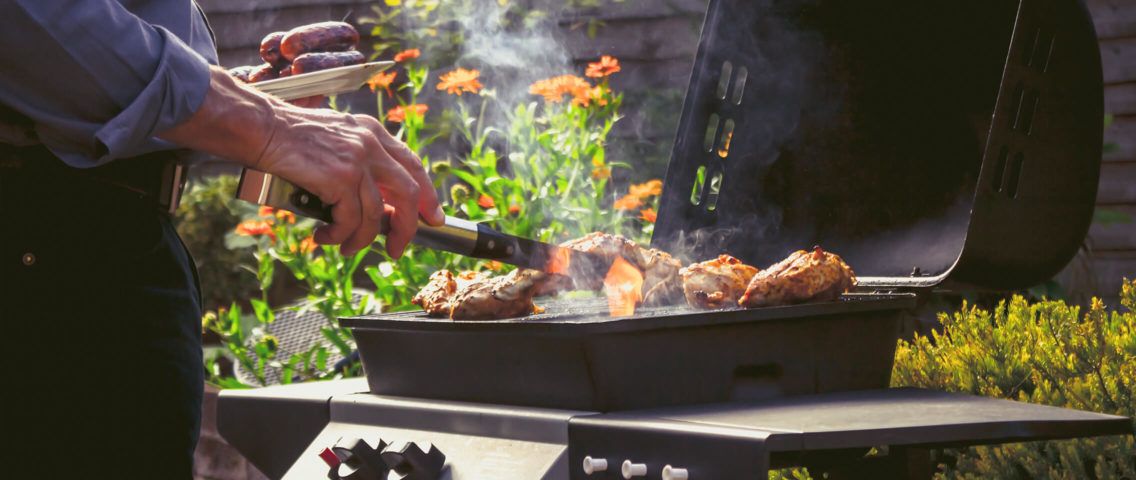
Not all retailers can accurately predict the impact of weather in their demand forecasts, but all retailers recognize how much weather impacts their demand. Consider this: the first heat wave of the year is due to hit a regional cluster of your stores this weekend. You expect local shoppers to stream in to pick up beer, hamburger fixings, and bags of charcoal to kick off the first proper cookout of the season. While your customers look forward to a relaxing weekend with family and friends, your supply chain planners kick into overdrive.
Your inventory team’s ability to accurately predict and prepare for the impact of this weather forecast will mean the difference between capitalizing on an increased sales opportunity or losing money. If you let the nice weather catch you by surprise and fail to order enough stock to meet the demand increase, you’re going to lose sales.
On the other hand, if you overestimate the weather’s impact and see less of a demand increase than you’d planned for, you’ll have excess stock on your hands. For shelf stable products like the beer and charcoal, you face capacity challenges—but for perishable products like hamburger meat, you run the risk of suffering considerable financial loss from spoilage.
Weather Has a Complex Impact on Retail Demand
The “cookout weather” example is a good starting point to demonstrate the impact weather forecasts can have—but now consider all the different types of weather events that might impact retail demand. Think not just about bright and sunny cookout weather, but also unbearable heatwave weather, unbreaking drizzle weather, torrential downpour weather, crisp autumnal weather, snow flurry weather, ice storm weather, and every other type of weather that could possibly impact your stores’ demand.
Then consider each product across your entire assortment. Will demand for your rain boots be as reactive to the rainy season as it will be for your umbrellas? What about raincoats? Light-weight ponchos? Waterproof hats vs normal ball caps? Socks? Frozen mixed vegetables?
Of course, it may seem intuitive that frozen mixed vegetables aren’t particularly reactive to rainy weather. The point is simply that the math gets extremely complicated, extremely quickly. There are an enormous number of different weather factors that will affect different stores across your network on any given day, creating different impacts on different items across your assortment.
It becomes clear that not all weather is actually impactful. In grocery retail, for example, day-to-variation in temperature, cloud cover, or rainfall are unlikely to impact sales forecasts because people have to eat no matter what. The challenge, then, is to automatically identify the specific combinations of factors that will actually impact demand—like that first cookout day of the summer.
This challenge is ultimately too data-intensive for any planning team to manage manually. Retailers must turn to AI-driven demand forecasting software to process enormous data sets and run enormous numbers of calculations. Only then can they automatically define the relationships between all potentially demand-influencing weather factors and actual demand at a granular, product-store level.
Machine learning algorithms drive significant improvements to sales forecast accuracy. With the right technology solution and strategy in place, retailers can accurately prepare for weather-related demand fluctuations and ensure they’re capitalizing on opportunities, not suffering losses.
Machine Learning Considers the Weather in Demand Forecasts
Traditionally, retailers have used time-series models to build baseline forecasts. This approach uses the recurring patterns in historical data—for example seasonality and weekday variation—to make predictions about future demand. Machine learning is necessary, though, if you wish to accurately identify and model the impact of weather. Furthermore, a fully machine learning-based forecasting system is able to automatically calculate how that weather impact will interact with a range of other factors to impact overall demand.
Machine Learning Considers A Broad Range of Demand-Influencing Factors
Demand forecasting software equipped with machine learning algorithms can consider a wide range of potentially demand-impacting variables within a single forecast model. These variables fall broadly into three categories:
- Recurring variations in baseline demand, such as weekday-related and seasonal variations.
- Internal business decisions designed to capture consumer attention and provide a competitive edge, such as promotions, price adjustments, or changes to in-store displays.
- External factors, such as local events, changes in a store’s neighborhood or competitive situation, and this paper’s subject—the weather.
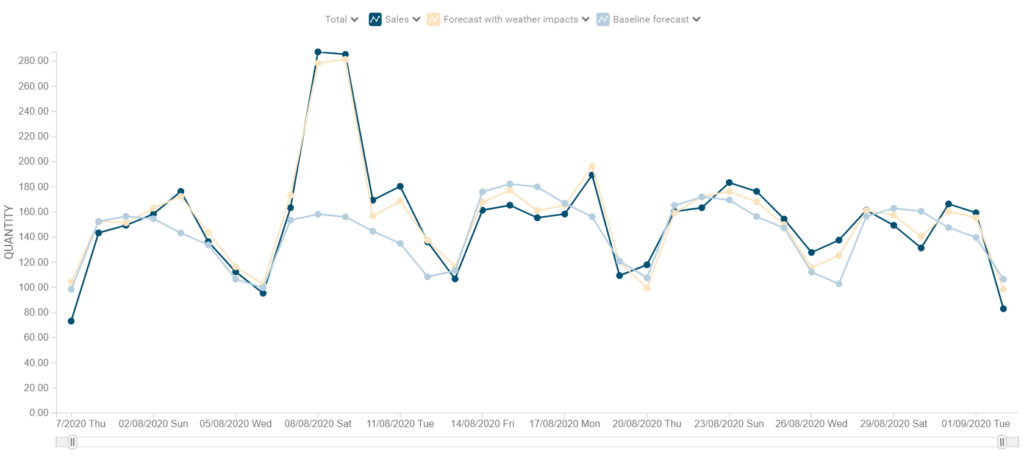
No planning team, no matter how large or talented, could humanly process such an immense amount of data on a continuous basis. However, machine learning-driven software can make millions of these forecast calculations within a single minute’s time, with advancements in large-scale data processing and in-memory technology.
How Machine Learning Designed for Retail Identifies Demand-Impacting Weather
Accurately capturing the impact of weather on demand is more complicated than merely linking temperatures to outcomes. Most of the data processed in such a manner would be largely useless for forecasting because the vast majority of days in a given year have temperatures within a normal, expected range, and therefore do not actually impact demand. Furthermore, these daily temperatures have a strong correlation with seasonal patterns already captured in existing historical data—it normally gets warmer in summer and it normally gets colder in winter.
A successful approach calls for clever feature engineering, or the application of specialized industry knowledge to help the solution extract usable information from raw data in a form that can be put to work in a retail setting. When a solution is equipped with intelligent feature engineering designed for retail, machine learning can explain otherwise uncapturable demand variations that are actually attributable to weather observations and forecasts. These models can predict how a local weather forecast will interact with every other demand-influencing factor for which you have available data, creating a full image of actual demand at a granular, product-store level.
Machine Learning Calculates the Demand Impact from All Influencing Factors
The “first cookout weekend of summer” is a perfect example of truly demand-impacting weather: it’s warmer than average for that time of year, encouraging the masses to take advantage. So what does it look like when a demand forecasting solution is put to work to calculate how weather interacts with other factors to impact demand?
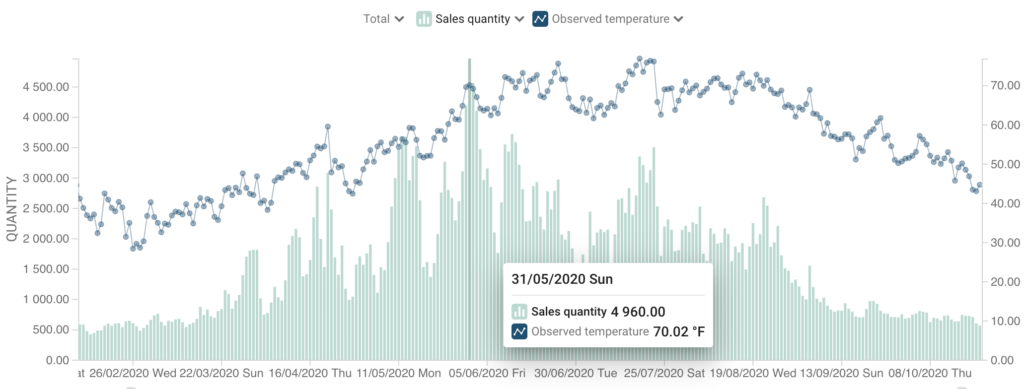
As a starting point, your demand planning software must be able to integrate with a provider of accurate global weather and weather forecast data and automatically fetch relevant data based on store location. Rather than having to manually check and consider weather forecasts for every store in their network, central demand planners can simply review automated store-level forecasts that have already taken local weather into consideration.
Let’s build on that starting point by considering how demand might change when two factors interact: whether or not it’s sunny (a local, external weather factor) and the day of the week (a recurring variation). A bright, sunny day might drive ice cream sales on a Saturday, when many consumers are relaxing on a work-free weekend. The impact of a bright sunny Tuesday might be significantly smaller, though, with tired workers more interested in going home than in taking a detour to buy ice cream. A good planning system must be able to consider how weekday variation and weather factors interact to change demand.
Now, let’s add another factor to sunniness and day of the week: temperature (also an external weather factor). The brightest, sunniest January Saturday that Boston has ever seen will still be extremely cold, and it’s unlikely shoppers will be in the mood for ice cream.
A sunny January Saturday in Miami, with its warmer temperatures, though, just may drive some additional sales. Now what happens when you put your highest-selling brand of ice cream on promotion (a business decision) to capitalize on this anticipated, weather-driven demand increase? Will demand for unpromoted ice cream products decrease as a result of sales cannibalization?
Because an AI-driven demand forecasting software analyzes all demand factors within the same model—including seasonality or weekday variation, business decisions like promotions, and weather data—it can answer such questions easily.
Demand Forecasting Automation and Accuracy Lead to Opportunities
It would simply be too time consuming and complicated to manually calculate the relationship between each product, product category, weather variable, and store location—and the resulting forecasts would likely be inaccurate. Retailers whose planning software automatically considers weather in their demand forecast calculations stand to reap substantial benefits.
AI-driven processes automate the millions of calculations required to model the intricate relationship between weather, other external factors, your business decisions, and the normally recurring variations in your demand patterns. They also automatically identify which products in the assortment are most reactive to different weather variables and what the magnitude of those relationships is.
The result is highly automated, accurate, localized demand forecasts that enable your inventory team to proactively prepare for significant weather-related demand changes. In grocery retail, we’ve found that the ability to capture the effect of weather in demand forecasts can reduce forecast errors by up to 75% for weather-sensitive products in times of unusual weather, such as heat waves.
Of course, forecast accuracy alone is never the end-goal in retail. More important is how a weather-proactive forecast can be leveraged across retail operations to improve outcomes. Retailers stand to capitalize on weather-driven sales opportunities through improved replenishment and availability, and can also significantly reduce fresh spoilage by ensuring the right amount of inventory is being delivered to each store. Ultimately, a demand forecast that reflects the impact of local weather on sales adds continuous value throughout your retail operations.