How can AI enhance sustainability in supply chain?
Nov 28, 2024 • 16 min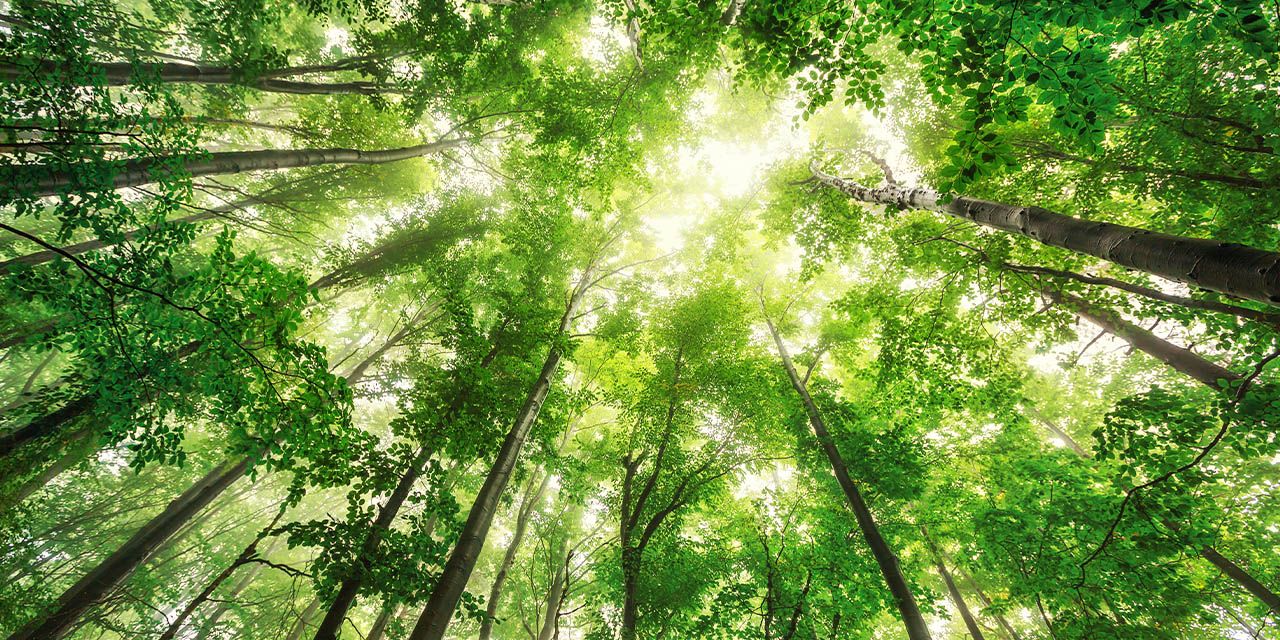
At times, it seems like artificial intelligence (AI) and sustainability are at odds. On one side, AI holds out the promise of greater efficiency, speed, and accuracy. On the other, sustainability decries the additional strain of these technologies on power grids and resources.
There is credence to these concerns, but businesses should maintain an even-handed approach. A wide array of AI capabilities can be added to a supply chain piecemeal, allowing companies to test and select AI strategies that support critical initiatives like sustainability.
How can AI enhance sustainability in supply chain?
The strategic use of AI enhances sustainability in supply chain processes by limiting power consumption on the back end and by reducing waste throughout the supply chain. Many of these capabilities have the added benefit of increasing efficiency, resulting in wider margins and better customer satisfaction.
Plus, with the advent of stricter regulations, AI can play a role in sustainability reporting so companies can measure and track emissions, as will soon be required by law in many regions.
The stakes: Why sustainability needs AI
As supply chains wrap around the globe, their environmental impacts are becoming harder to ignore. Let’s take food supply chains as an example.
One-third of our food is wasted, contributing to deforestation and loss of biodiversity and emitting 8-10% of Earth’s total greenhouse gases. Meanwhile, one in three people cannot afford a healthy diet, and one in 11 don’t have enough to eat every day.
To address this, the UN has some ambitious goals for 2030, including cutting food waste by half and eliminating hunger globally. Progress towards these goals has been slower than expected.
Food waste emits 8-10% of Earth’s total greenhouse gases.
So where does AI come in?
About 13% of food produced is wasted upstream in the supply chain between the harvest and retail stages, and about 19% is wasted in retail, food service, and homes. AI-driven planning systems are all about increasing efficiency and accuracy, helping companies drastically reduce food waste and avoid overstocks that lead to spoilage.
By weaving AI into their planning processes, companies could catch up to that 2030 timeline, lessening the very real impact of supply chain waste on the environment and vulnerable communities, while also getting ahead of pending regulation and eliminating costly inefficiencies.
Sizing up the challenges
A successful AI-driven sustainability initiative has some hurdles to jump first. Some of these may seem merely technical issues at first, but they have ripple effects for the planet as well.
Excessive power consumption
On a global scale, the elephant in the room is the amount of energy AI eats up. Many are looking to gen AI as the silver bullet for planning challenges. One study, however, found that a gen AI system consumes “around 33 times more energy than machines running task-specific software.”
This leaves business leaders with two questions:
- What’s the point of leveraging the latest AI to reduce waste if the amount of energy required renders the result null?
- How can they stay on the cusp of innovations while reducing the risk of getting slapped by incoming regulations?
Speaking of regulations…
Increased regulatory requirements
Environmental regulation is coming. Pressure is mounting for companies to report all Scope 3 emissions; Europe and California are already scheduling laws to come into effect in the next year or two.
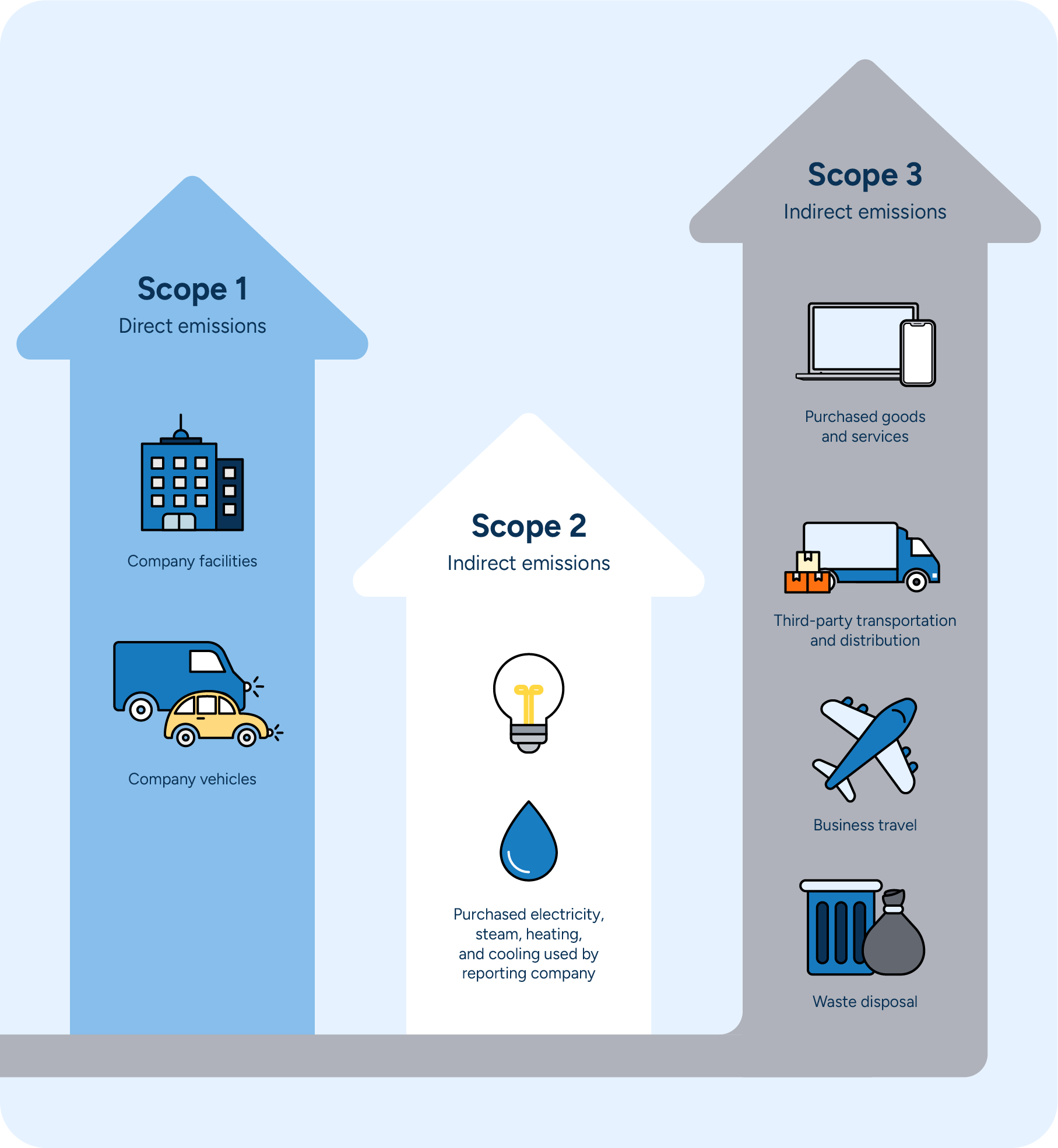
Beginning in 2025, companies in Europe – including those headquartered elsewhere but with European operations – will be required to report indirect emissions across their supply chains. Companies will need to collect, organize, analyze, and report more data than ever. This complicates things because many companies already have to contend with…
Poor data management and low forecast accuracy
Supply chains are swirling vortices of data, brimming with demand fluctuations, vendor information, weather events, and other factors that affect planning decisions. Many companies lack the storage, processing, and analytical capabilities to uncoil all this information and extract actionable insights.
Without those insights, forecasts – especially those based on historical data – can be rendered useless in a matter of minutes. Large supply chains, hampered by technical debt, can’t exactly turn on a dime when plans need sudden adjustments. One delayed delivery can require forecast recalculations that simply cannot be done in time with the resources available.
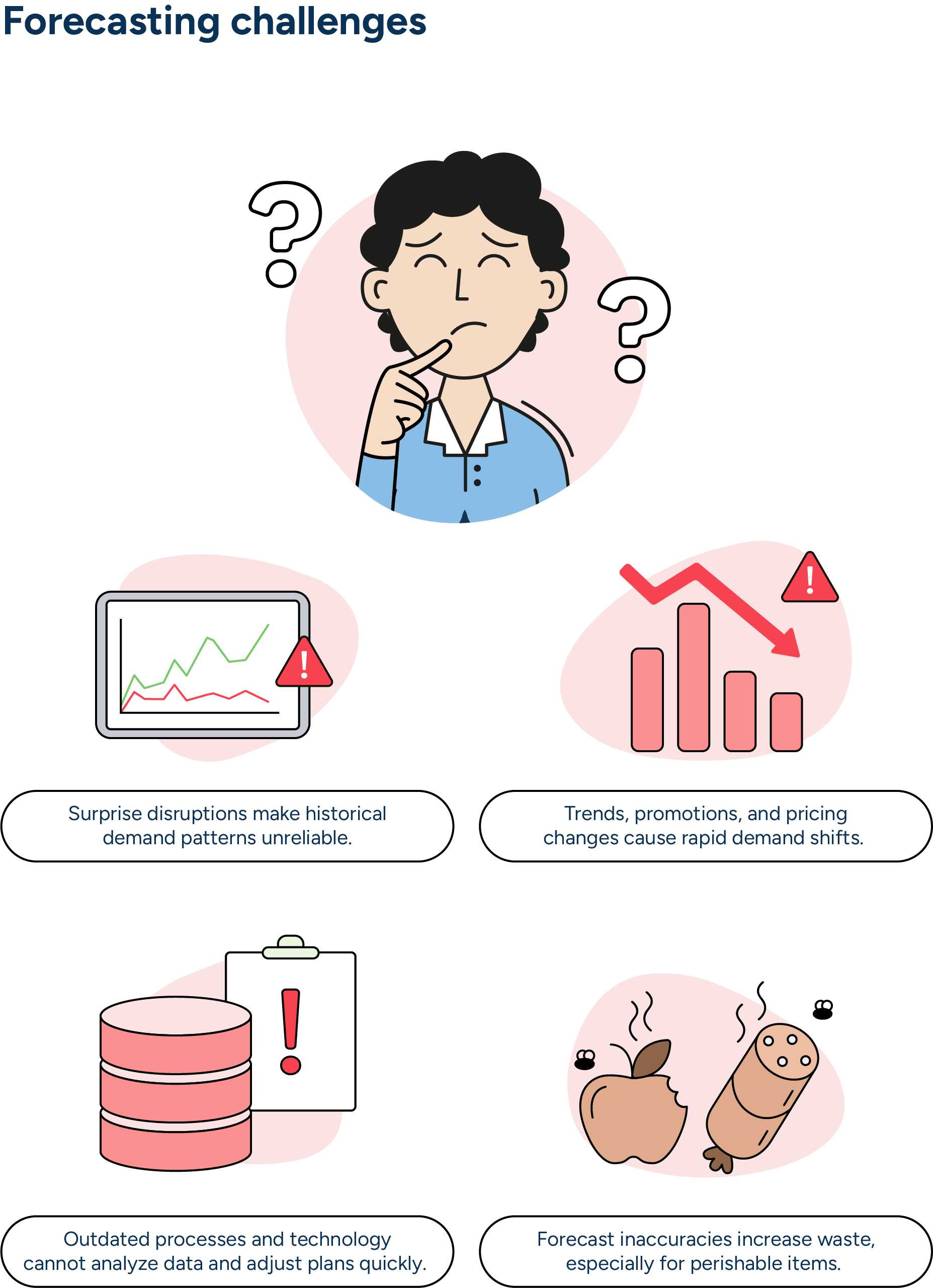
How data-poor forecasting hinders sustainability initiatives: Everything in supply chain planning rests on the forecast. If the forecast is wrong, inventory is thrown off balance, leading to excess stock, spoilage, and waste – from the production stage to the store floor.
Legacy systems and siloed planning
Suboptimal forecasting methods are often ensconced in legacy planning systems and spreadsheets. Guesstimates and human errors make their way from the forecast to other planning functions and back again, magnified.
That’s if the planning functions are communicating at all. Spreadsheets and traditional planning solutions don’t provide the scalable cloud storage, rapid data processing and analysis, and cross-functional data sharing required for collaborative planning. Instead, these disparate systems put planners in silos with poor data and limited analytics, hampering efficient end-to-end planning.
How legacy systems and planning silos hinder sustainability initiatives: Lack of visibility makes it difficult to coordinate distinct planning functions and create a cohesive, end-to-end strategy that aligns inventory with actual demand. It creates a cascade effect of inefficient resource use, increased spoilage, and waste throughout the supply chain.
4 ways to implement sustainability-enhancing AI
Good news: Many of these challenges already have solutions.
Here are some ways manufacturers, distributors, and retailers around the world use AI to develop sustainable supply chains without compromising other business objectives.
1. Diversify your AI tools to minimize power consumption
There are many types of AI in supply chain, and not all of them guzzle energy as much as gen AI currently does. One such type is specialized AI.
Madhav Durbha explains: “Unlike gen AI, specialized AI does not rely on LLMs, which can be energy-intensive and not suitable for most supply chain computations; instead, it uses less energy-intensive machine learning algorithms trained for specific tasks. By synthesizing large amounts of data and learning how to interpret that data over time, specialized AI can significantly improve end-to-end supply chain performance, from sourcing to manufacturing to inventory levels in stores or warehouses.”
“Specialized AI uses less energy-intensive machine learning algorithms trained for specific tasks.”
Specialized AI helps planners determine optimal decisions for specific planning functions – all while consuming minimal energy and producing minimal waste. For illustration purposes, let’s review some of the most noteworthy applications of specialized AI in supply chain and retail planning.
Machine learning-based forecasts
ML-based forecasting solutions easily incorporate vast data inputs and continuously assess supply chain performance to refine forecast calculations over time. They anticipate demand drivers and leverage multiple data sources to construct a clear, accurate picture of demand that forms the basis of effective supply chain planning.
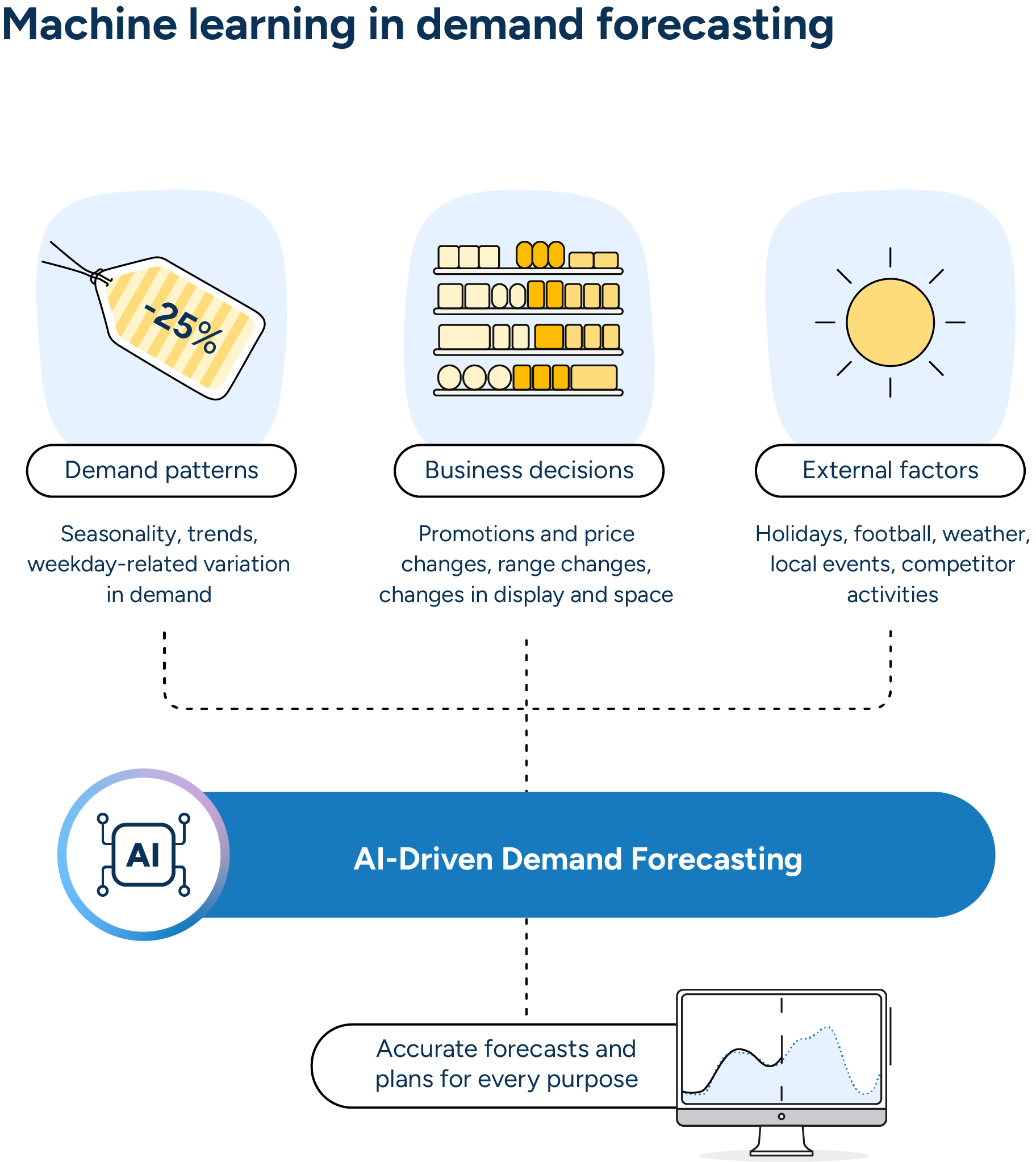
How ML-based forecasts support sustainability: ML-based forecasts pinpoint inventory needs to ensure stock, resources, and capacity are used efficiently. They help companies avoid spoilage, overproduction, and landfill-bound overstocks – not just for finished goods but at every stage of production. Better forecasts also contribute to efficient logistics and transportation, limiting associated carbon emissions.
Read more: The complete guide to machine learning in retail demand forecasting
Demand sensing
Demand sensing seamlessly integrates with machine learning forecasts and incorporates additional signals, like retail POS data, to capture sudden demand changes. Using this data, it creates accurate, dynamic forecasts that adapt to market shifts for faster responses. This is especially useful for CPG and manufacturing companies who need accurate short-term plans to adjust production quickly.
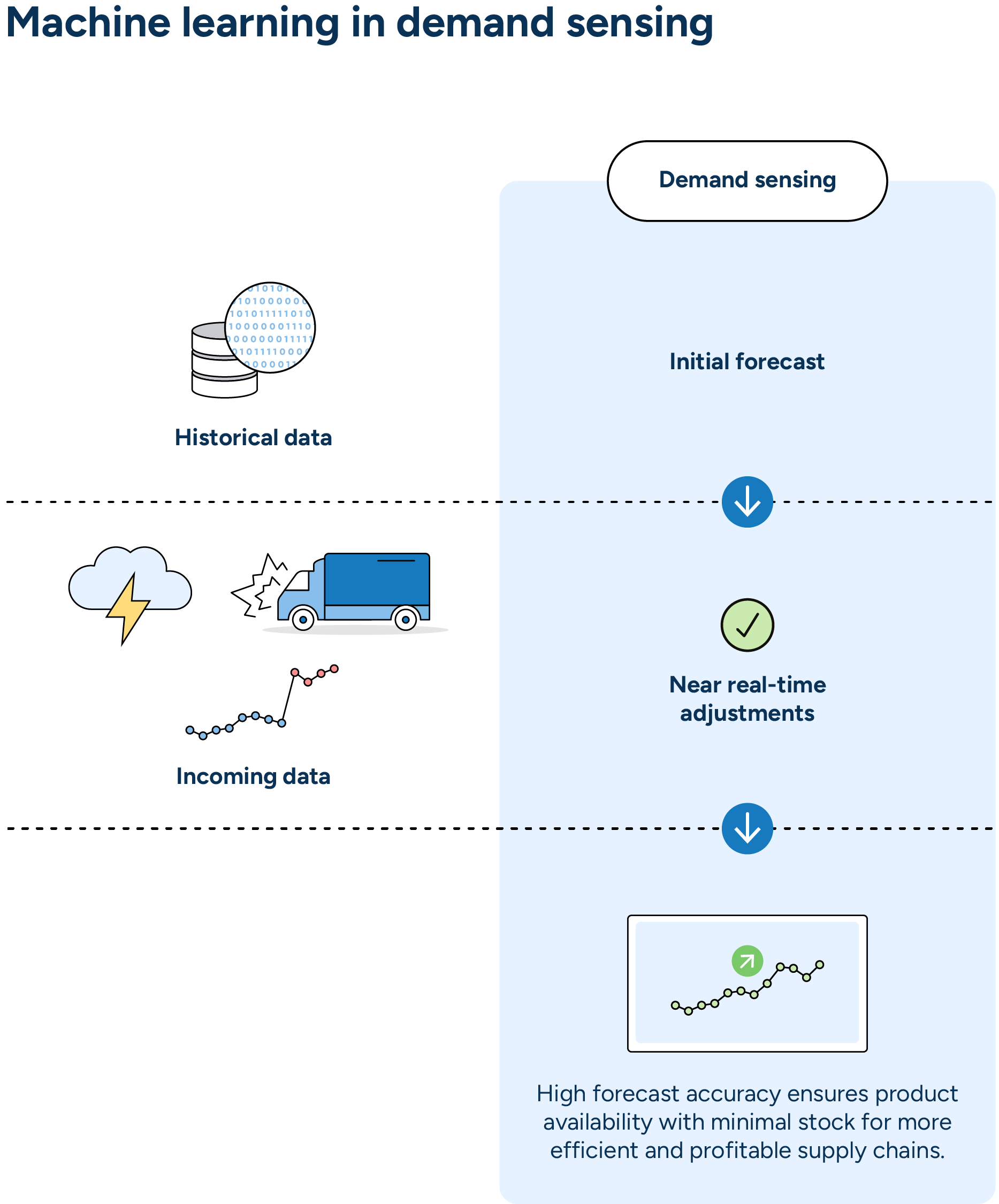
How demand sensing supports sustainability: Demand sensing improves inventory turnover by tailoring production and procurement to actual customer demand. By calculating timely, accurate order volumes for each product, it reduces excess inventory, especially for perishable or seasonal items.
Read more: Demand sensing – How to conquer CPG supply chain chaos
AI-driven promotions planning
By broadening its scope to include items not specified as part of a promotion, AI-powered promotions planning detects and models the effect of inter-item demand, accounting for cannibalization and halo effects. The halo effect represents positive impacts, such as an increase in sales for Item B caused by the promotion of Item A. Cannibalization models outline the potential negative effects, in which promoting Item A will decrease sales for Item B. In a unified solution, this information is transmitted to other teams to help refine replenishment plans and forecasts.
For instance, in the hypothetical example below, a supermarket carries both “HappyCow” and “GreenBeef” brands of lean organic ground beef. If HappyCow is promoted, this may cause cannibalization, a temporary dip in GreenBeef sales. Planners can use AI models to accurately forecast sales to avoid overstocking – and wasting – GreenBeef products.
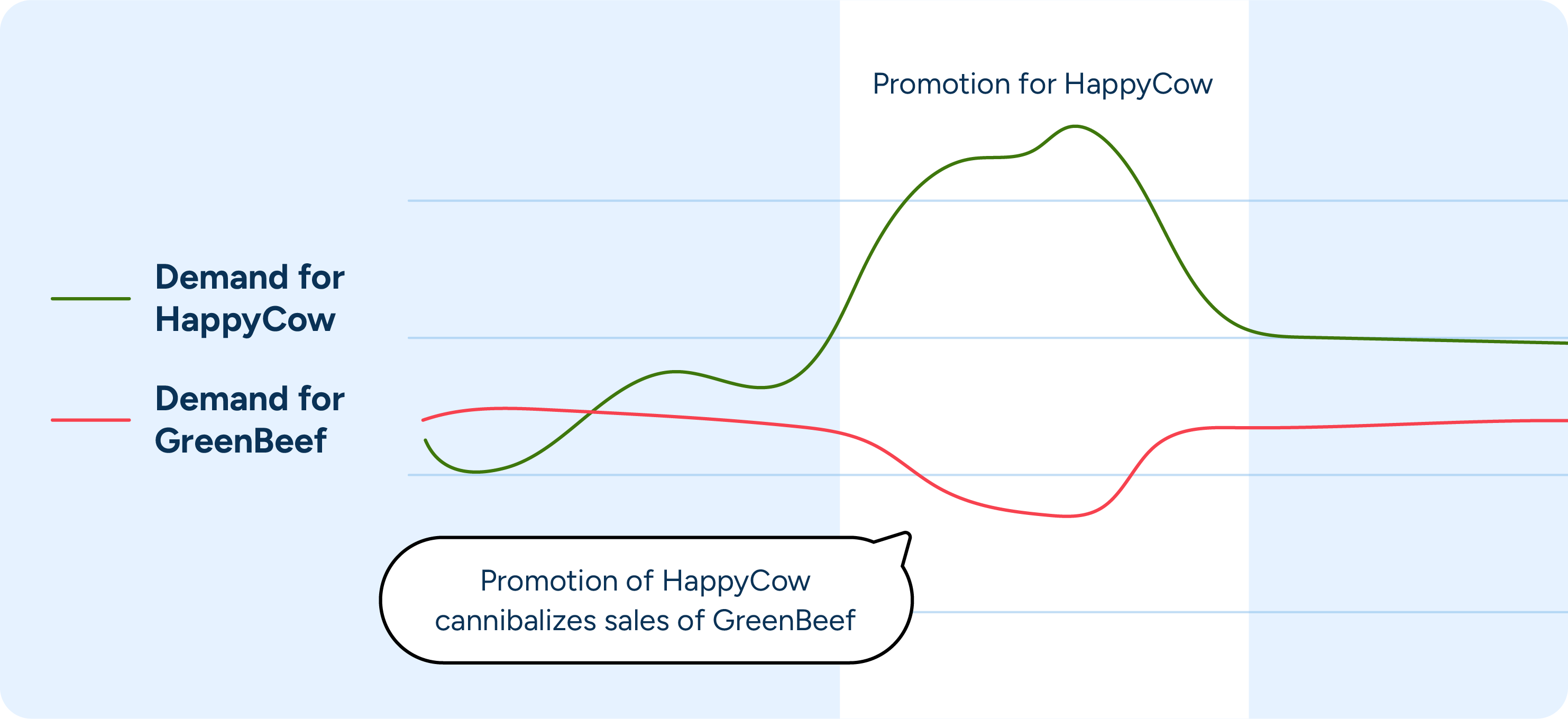
How promotions planning supports sustainability: By accurately predicting promotional impacts, companies better align inventory levels with demand, minimizing overstock and limiting the consumption of raw materials, energy, and labor. By coordinating promotions with logistics, companies optimize transportation schedules and routes, reducing the number of trips and associated carbon emissions.
Read more: Why retailers need to integrate pricing and promotions
Predictive inventory
Maintaining accurate and current inventory data for products with short shelf lives is particularly challenging. Grocery retailers need new methods to conquer issues like lost sales, spoilage, and phantom inventory, caused by data management challenges and store-level counting errors.
Predictive inventory uses statistical and machine learning methods to detect anomalies and estimate inventory drift and uncertainty, creating a synthetic view of inventory that is more accurate than actual inventory records.
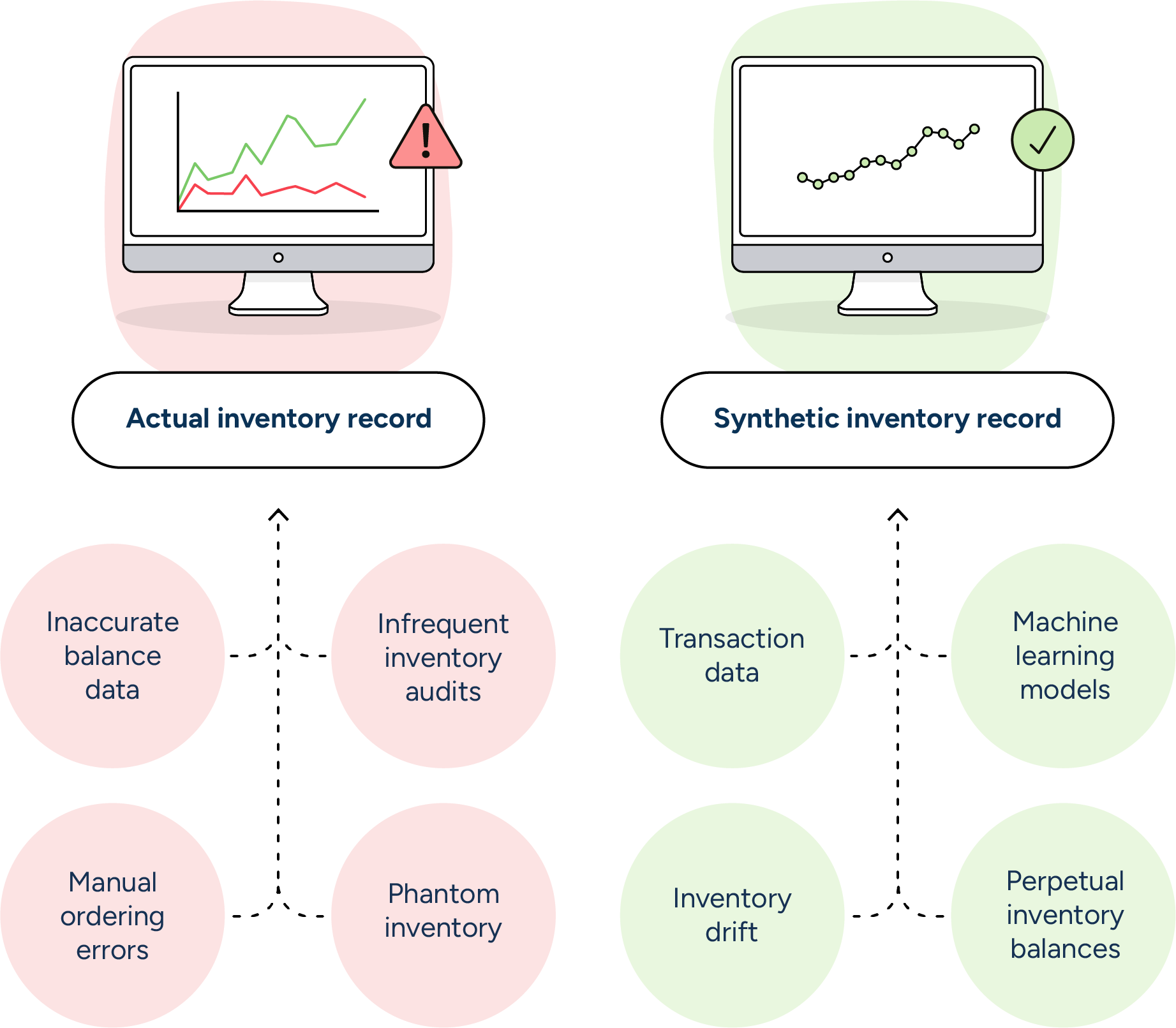
How predictive inventory supports sustainability: With a better understanding of product behavior and inventory levels, retailers can protect freshness, availability, and margins, while reducing food waste and avoiding spoilage.
Read more: Why a synthetic inventory is probably better than your real inventory
2. Implement an AI-supported emissions tracker now
Chances are companies don’t have the bandwidth and resources to develop an efficient emissions tracker in-house. The closer this tracking solution is to your planning solution, the better. The same platform that analyzes an expanded pool of data and delivers AI-supported calculations is the ideal place to track the emissions resulting from those planning decisions.
One such example is RELEX CO2 Analytics, a solution that tracks the CO2 emissions of purchased items and food spoilage so businesses can better understand and reduce their environmental impact.
Here’s how it can estimate emissions at the product or product group level.
- To establish a reference point, CO2 Analytics uses an external database containing emissions data for items similar to a company’s product portfolio.
- Using the company’s master data, RELEX applies a pre-trained neural network language model to review the product attributes and compare them to the products in the emissions database.
- By identifying similarities and matching product profiles, RELEX determines the estimated CO2 emissions associated with each of the company’s products.
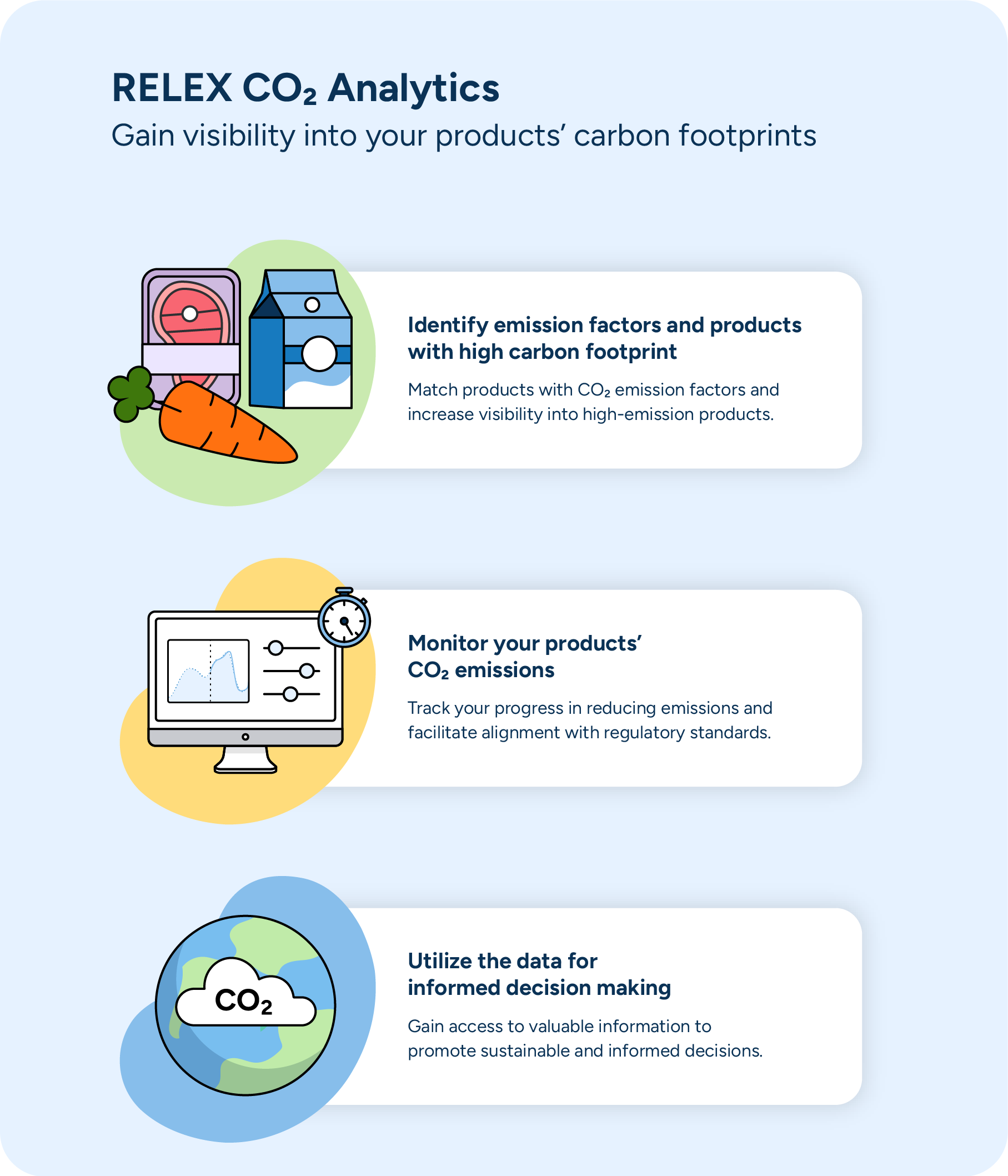
Companies can use these data insights to monitor product CO2 emissions and make better-informed decisions that promote sustainable practices. Currently, the solution focuses on food items but will eventually expand to include non-edible products.
3. Refine data and unify planning functions
AI can clean up and analyze huge amounts of data. For instance, level shift detection identifies significant step changes in demand to help planners refine forecasts. Data cleansing techniques use machine learning to improve datasets by correcting errors, removing duplicates, and filling in data gaps using statistical methods and domain knowledge.
Read more: Use AI to make messy datasets useful, not perfect.
That data can vastly improve planning outcomes, but to do that, it needs somewhere to go. The last thing you want to do is clean up and process all that data, generate those great insights, and then leave them to molder in a silo.
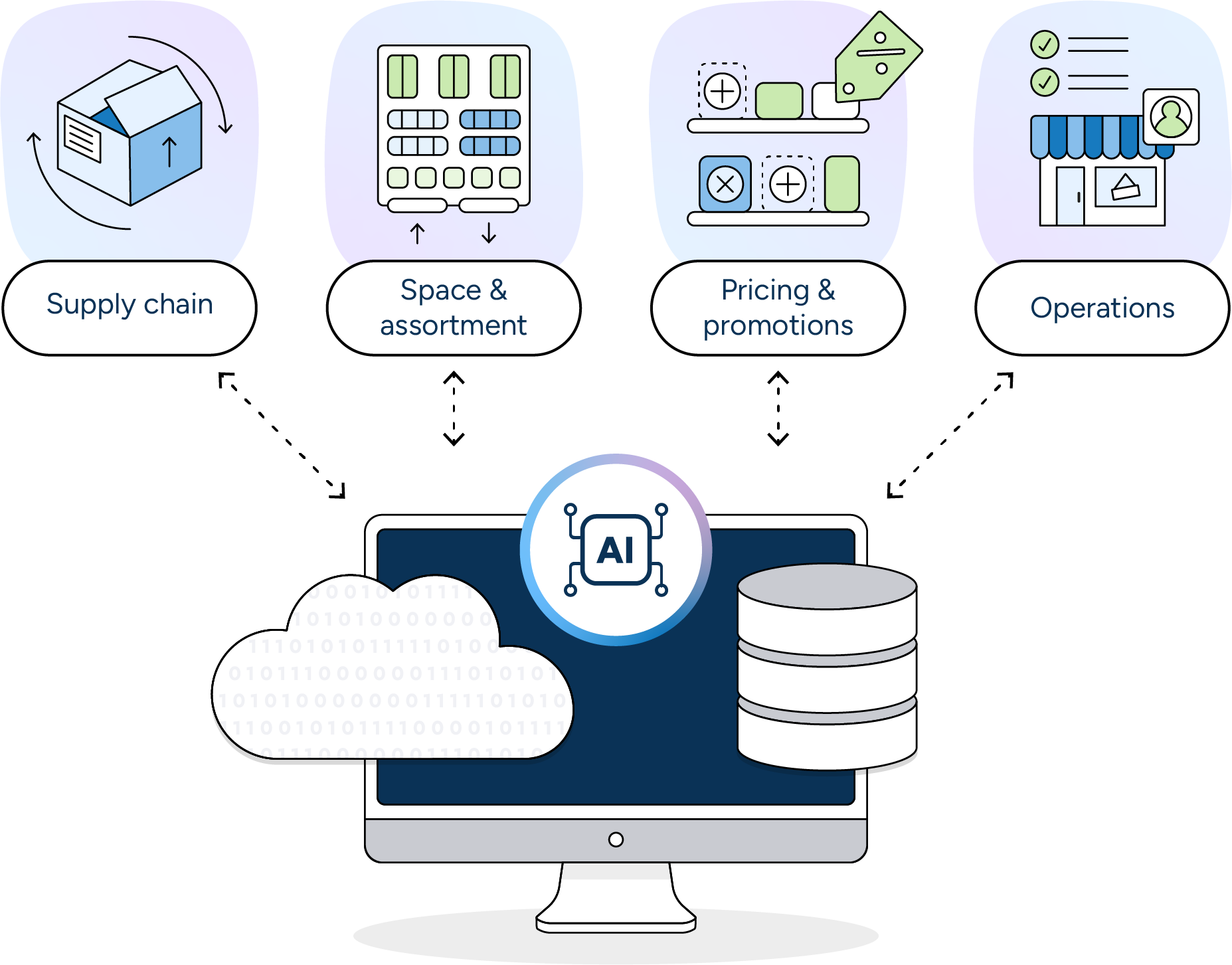
This is why companies need a solution that enables cross-organizational data sharing and connects all the AI planning capabilities on one unified platform. This platform provides visibility, so teams can collaborate in real time.
Retailers can extend this collaboration to the store level. Mobile applications ensure that store employees have access to AI-supported task management. These applications provide visibility into the logic behind those task recommendations and allow associates to contribute store-level insights to further improve calculations.
4. Don’t neglect gen AI
Like many new technologies, resolving issues like power requirements for gen AI may take a little while. In the meantime, how can businesses create a gen AI strategy that preps them for future capabilities and helps them take advantage of current benefits to achieve ROI, increase productivity, and reduce spoilage and waste?
The answer: Use gen AI to empower users and increase productivity today and integrate it into your planning solution so you can easily implement innovations in the future.
In 2023, RELEX introduced Rebot, the first gen AI supply chain assistant on the market, and customers are currently using it for best practice and solution queries. RELEX is actively exploring new ways Rebot could seamlessly integrate into daily workflows and gain the data analysis skills and tools for taking or recommending actions.
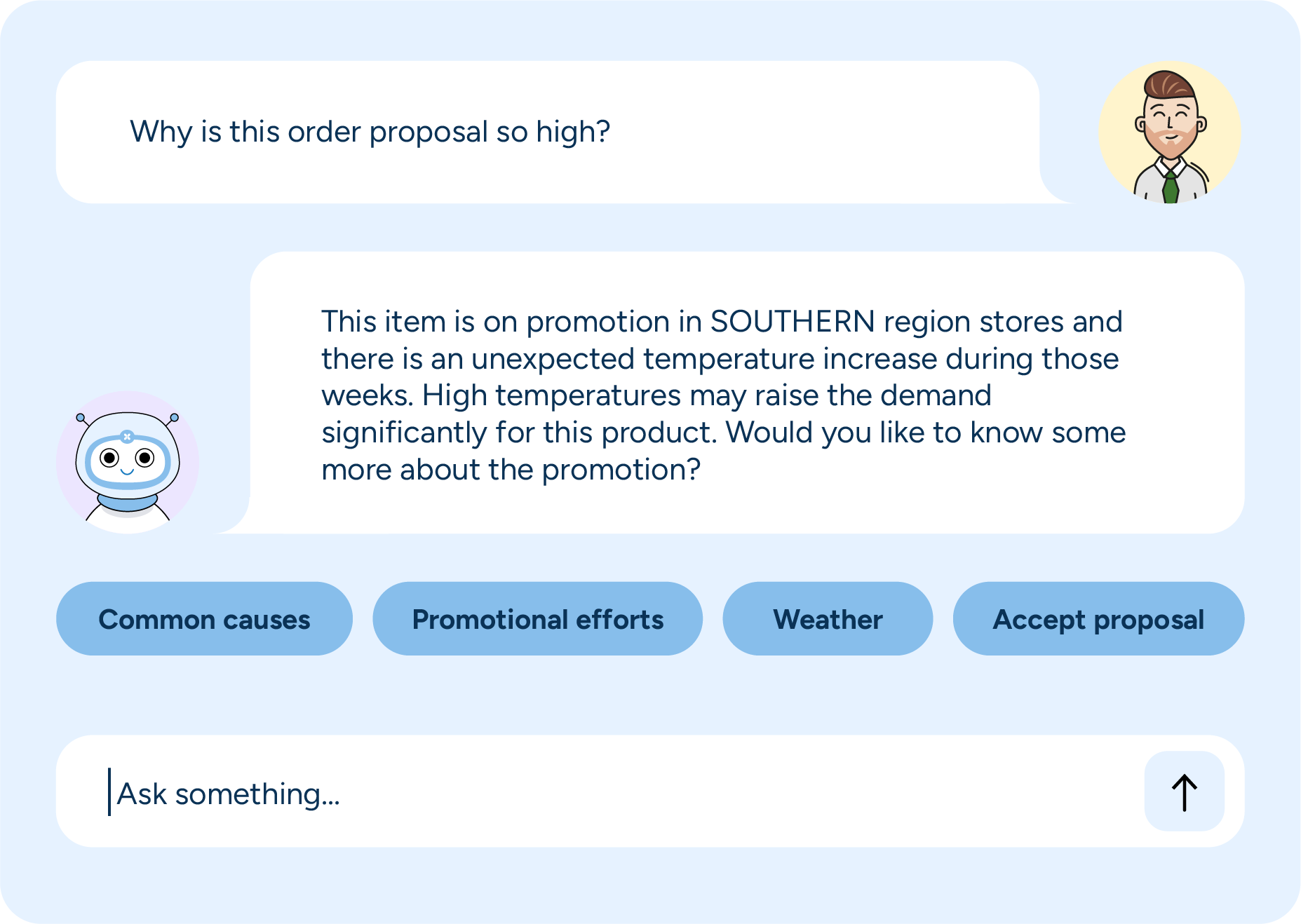
Though still under development, the possible applications of Rebot have positive implications for sustainability. For instance, Rebot could alert planners that a certain batch of inventory is about to spoil and suggest ways to mitigate those spoilage risks. It could learn specific sustainability KPIs and constantly monitor the supply chain to achieve those goals in ways that might otherwise have been missed.
Read more: Eliminating AI ambiguity
How AI benefits sustainability
With the right tools, supply chain and retail planning can become the epicenter of positive change and deliver results that benefit both the bottom line and the planet.
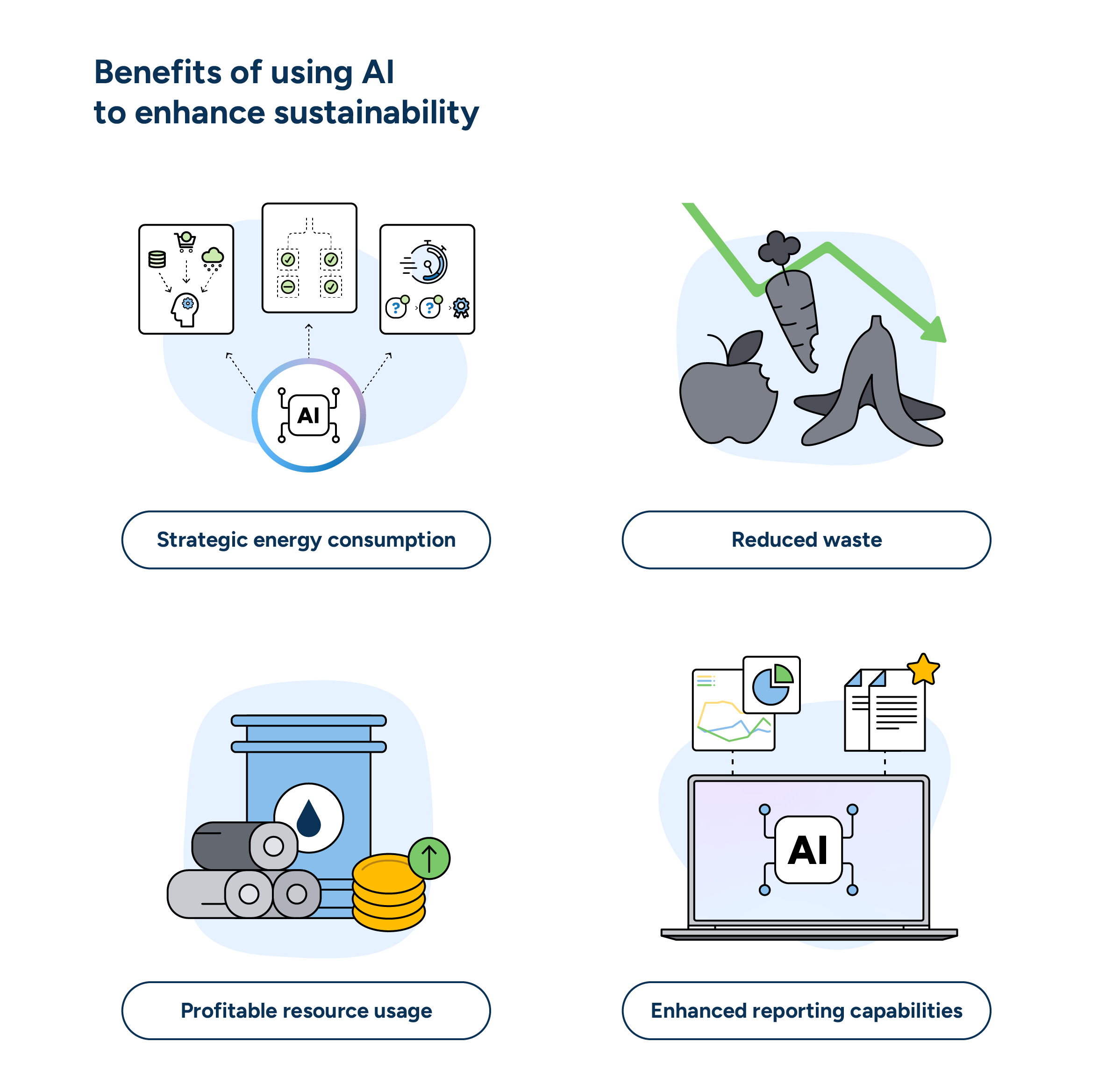
Strategic energy consumption
By using supply chain-specific gen AI agents, underpinned by the specialized AI capabilities that have been humming along for years with no noticeable strain on the power grid, companies across industries can shrink their carbon footprints and generate planet-friendly outcomes.
Reduced waste and lower emissions
Among those planet-friendly outcomes is waste reduction. Using the type of AI best-suited to each task makes it easier to unify planning processes, match inventory to demand, track obsolescence and spoilage, and optimize inventory management.
For instance, with machine learning tackling the complexity of different expiration dates and supplanting poor inventory data with accurate synthetic records, these planning systems can significantly reduce waste, especially regarding food spoilage.
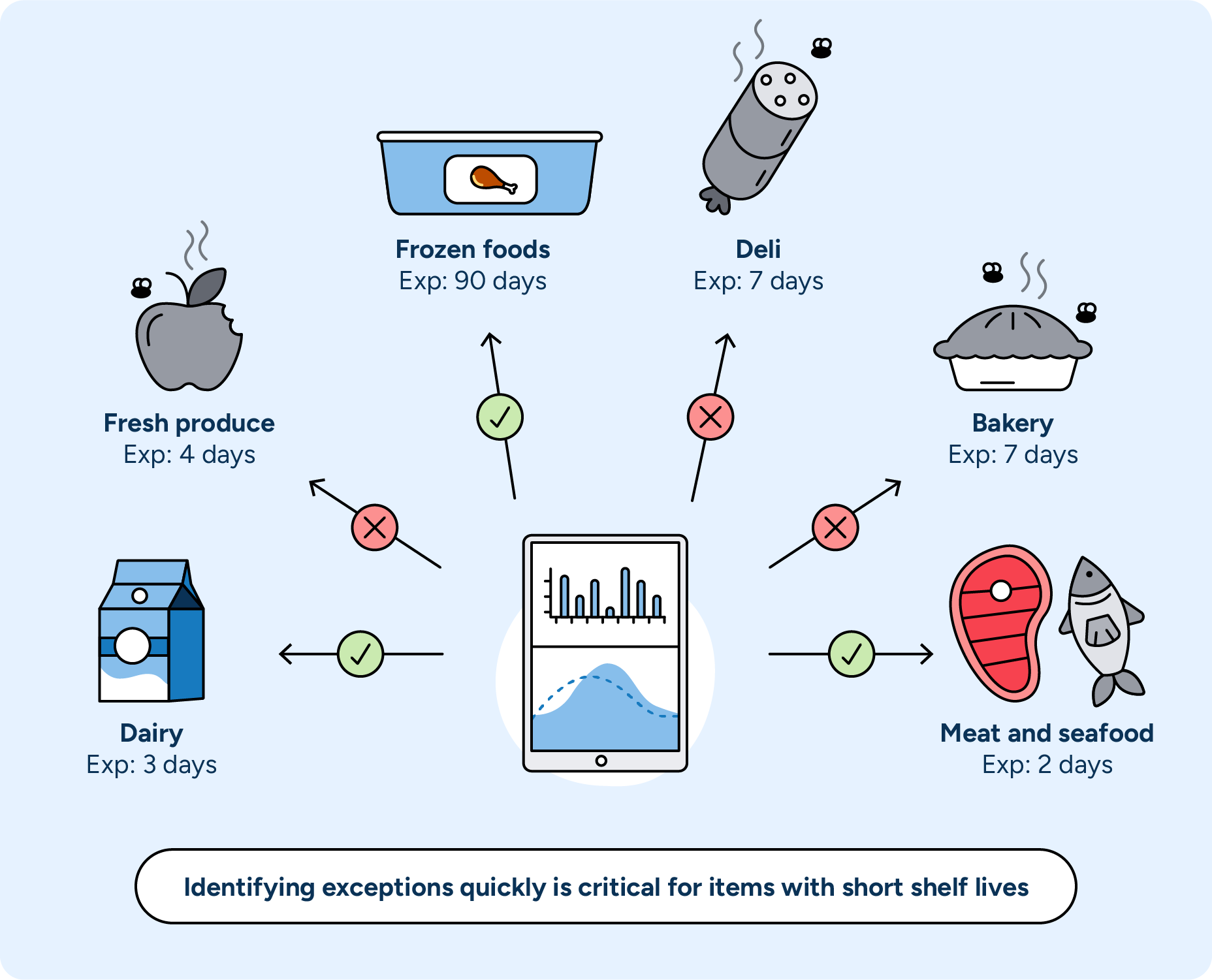
Reduced spoilage is a significant result at a company level, but accomplished on a broader scale, this level of waste reduction directly impacts greenhouse gas emissions. For instance, RELEX food retail and wholesale customers typically reduce waste by about 10-40% using our AI-driven platform. A conservative 15% reduction across all customers saves the equivalent of reducing the number of cars on the road by 200,000 in one year alone.
Better margins
Sustainability initiatives won’t be feasible long-term strategies if they rest too heavily on a business’s bottom line. The good news is that the same AI capabilities that reduce waste also protect margins. An ML-based demand planning solution fine-tunes forecasts so you’re only producing or ordering what you actually need to meet demand. AI-powered pricing and promotions ensure you’re balancing the impacts of price and demand to control stock. AI-driven inventory management guards against excess inventory at every stage of the supply chain.
You’re balancing demand, inventory, price, and availability. By reducing costs, freeing up working capital, and conserving resources, your supply chain stays efficient and profitable.
Enhanced reporting capabilities
Supply chains have an extravagant amount of data. Soon, businesses will be required to report on emissions from their immediate business operations, vendors, suppliers, franchises, and beyond.
Luckily, AI can help here, too. New techniques are emerging that enable companies to measure, track, and report emissions down to the product level. With these capabilities, companies can get ahead of the game, analyzing product emissions and developing a strategy to meet regulations before they come into effect.
How companies achieved sustainable outcomes with RELEX AI
The same technology RELEX uses to reduce operational inefficiencies in the supply chain is the same power we use to clean up the planet. By applying the right AI strategy to common industry challenges, we help customers reduce waste and lessen their environmental impact, all while achieving measurable business value like increased availability, customer satisfaction, and profitability.
Learn more about sustainability at Relex.
For example, Spanish grocery chain Ametller Origen saw a 10% increase in product availability, a 13% inventory reduction, and a 30% reduction in fresh spoilage. “We are making our way toward CO2 neutrality by 2027,” explained Roberto Gómez, Chief Operations Officer of Ametller Origen. “RELEX’s smart replenishment solution has enabled us to significantly reduce our environmental impact through more efficient processes. The high quality of their demand forecasts has led to a reduction in fresh produce shrinkage, improving sustainability throughout our supply chain. We look forward to building on this strong start to continuously improve our sustainability efforts while improving key outcomes throughout our supply chain.”
RELEX’s smart replenishment solution has enabled us to significantly reduce our environmental impact through more efficient processes.
Online grocery retailer Migros Online experienced similar results, with a 20% reduction in average spoilage rate despite 99+% availability and a 50% reduction in out-of-stock rates for promotional products.
“We were won over by RELEX’s best-in-class intelligent technology,” said Nicolas Soussan, Chief Logistics Officer at Migros Online. “We work with RELEX because it automates our goods procurement and reduces spoilage through their innovative application of AI and machine learning.”
Even during the Covid crisis, when the company experienced a massive demand spike due to consumer stockpiling, Migros Online used RELEX ML-based forecasting to increase the order growth rate by 35% and reduce spoilage to just 0.4% of total sales.
French convenience store retailer Franprix used AI-driven retail planning to decrease stockouts by 67%, achieve a $2.2 million USD reduction in inventory value, and improve margins by 2pp, all while decreasing product spoilage by 30%.
Building a planet-centric AI strategy
Let’s return to our original question: how can AI enhance sustainability in supply chain?
- It can limit power consumption through strategic use of different AI technologies.
- It reduces waste across the supply chains through improved efficiency and more accurate planning.
- It enables emissions reporting for compliance with upcoming rules and regulations.
So where do you begin?
First, look at your supply chain and identify the areas that need improvement. Where are you seeing the most waste? What areas need the most investment? Are there any small changes you could make for quick wins? What developing technology could you leverage to sustain those eco-friendly practices going into the future?
Once you’ve set your priorities, a diverse AI strategy can help you implement the right AI for each objective, reducing waste and improving resource use while delivering on promises to the end customer.